SeDR: Segment Representation Learning for Long Documents Dense Retrieval
CoRR(2022)
Abstract
Recently, Dense Retrieval (DR) has become a promising solution to document retrieval, where document representations are used to perform effective and efficient semantic search. However, DR remains challenging on long documents, due to the quadratic complexity of its Transformer-based encoder and the finite capacity of a low-dimension embedding. Current DR models use suboptimal strategies such as truncating or splitting-and-pooling to long documents leading to poor utilization of whole document information. In this work, to tackle this problem, we propose Segment representation learning for long documents Dense Retrieval (SeDR). In SeDR, Segment-Interaction Transformer is proposed to encode long documents into document-aware and segment-sensitive representations, while it holds the complexity of splitting-and-pooling and outperforms other segment-interaction patterns on DR. Since GPU memory requirements for long document encoding causes insufficient negatives for DR training, Late-Cache Negative is further proposed to provide additional cache negatives for optimizing representation learning. Experiments on MS MARCO and TREC-DL datasets show that SeDR achieves superior performance among DR models, and confirm the effectiveness of SeDR on long document retrieval.
MoreTranslated text
Key words
long documents dense retrieval,segment representation learning
AI Read Science
Must-Reading Tree
Example
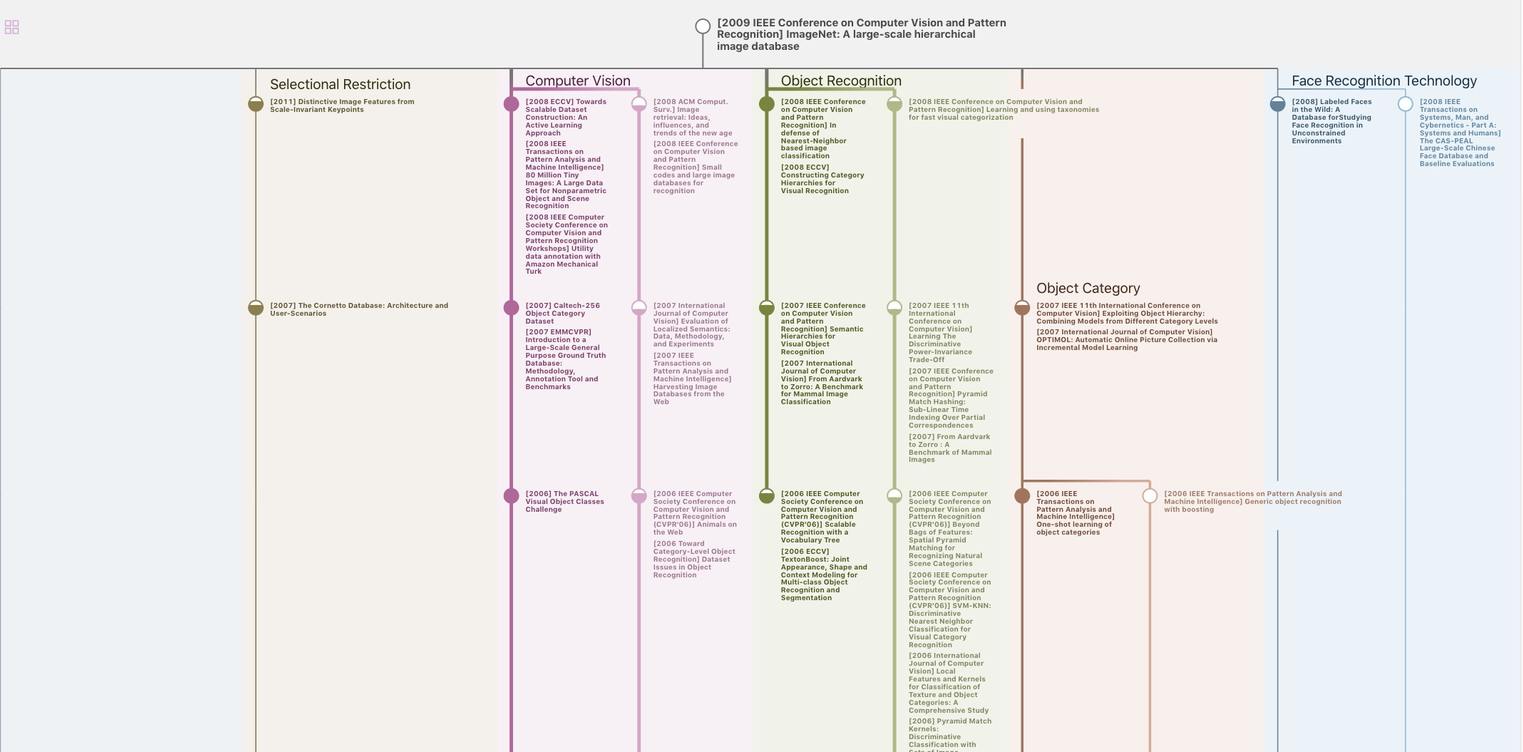
Generate MRT to find the research sequence of this paper
Chat Paper
Summary is being generated by the instructions you defined