Continual Learning-Based MIMO Channel Estimation: A Benchmarking Study
ICC 2023 - IEEE INTERNATIONAL CONFERENCE ON COMMUNICATIONS(2023)
摘要
With the proliferation of deep learning techniques for wireless communication, several works have adopted learning-based approaches to solve the channel estimation problem. While these methods are usually promoted for their computational efficiency at inference time, their use is restricted to specific stationary training settings in terms of communication system parameters, e.g., signal-to-noise ratio (SNR) and coherence time. Therefore, the performance of these learning-based solutions will degrade when the models are tested on different settings than the ones used for training. This motivates our work in which we investigate continual supervised learning (CL) to mitigate the shortcomings of the current approaches. In particular, we design a set of channel estimation tasks wherein we vary different parameters of the channel model. We focus on Gauss-Markov Rayleigh fading channel estimation to assess the impact of non-stationarity on performance in terms of the mean square error (MSE) criterion. We study a selection of state-of-the-art CL methods and we showcase empirically the importance of catastrophic forgetting in continuously evolving channel settings. Our results demonstrate that the CL algorithms can improve the interference performance in two channel estimation tasks governed by changes in the SNR level and coherence time.
更多查看译文
关键词
Continual learning,task transferability,channel estimation,MIMO,Gauss-Markov channel model
AI 理解论文
溯源树
样例
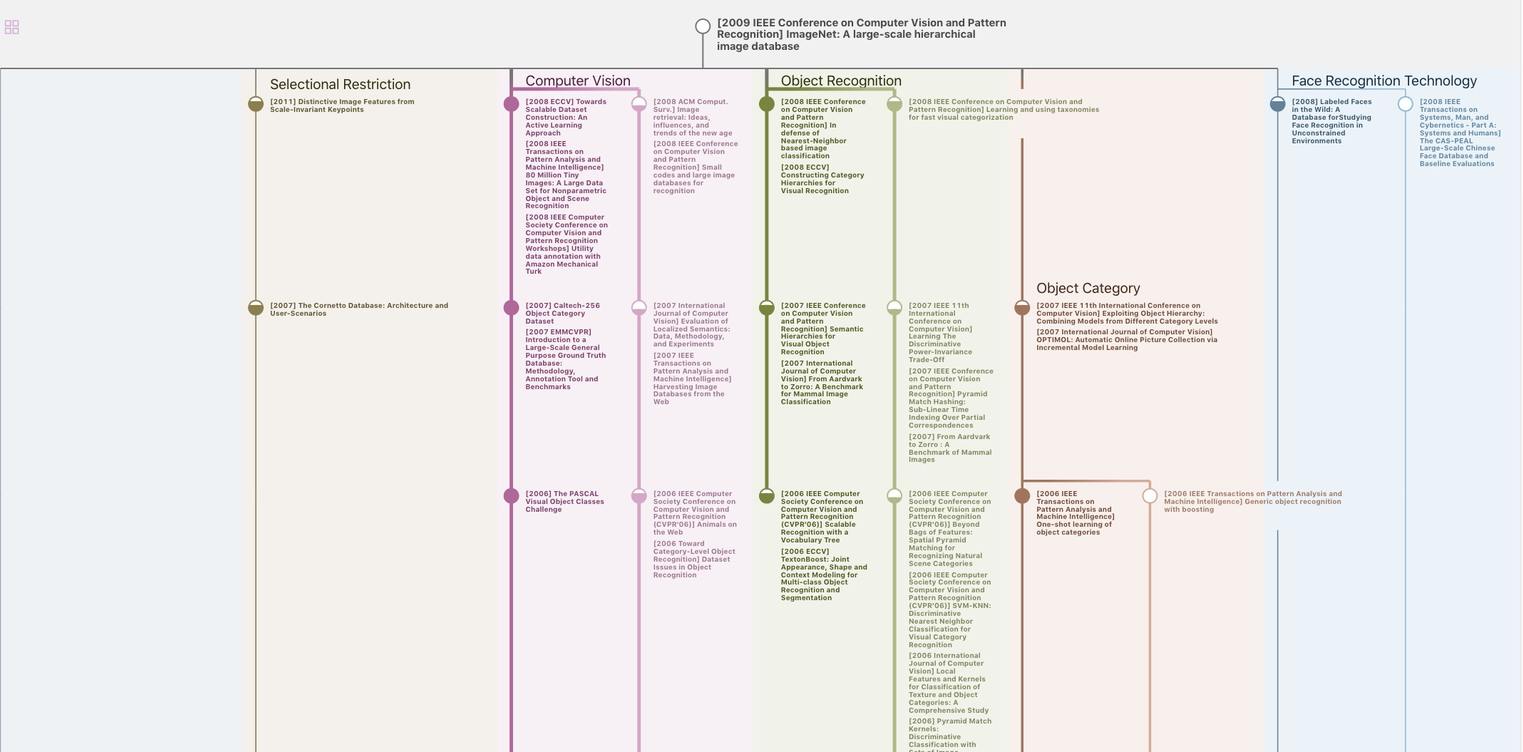
生成溯源树,研究论文发展脉络
Chat Paper
正在生成论文摘要