Simple Open-Vocabulary Object Detection.
European Conference on Computer Vision(2022)
摘要
Combining simple architectures with large-scale pre-training has led to massive improvements in image classification. For object detection, pre-training and scaling approaches are less well established, especially in the long-tailed and open-vocabulary setting, where training data is relatively scarce. In this paper, we propose a strong recipe for transferring image-text models to open-vocabulary object detection. We use a standard Vision Transformer architecture with minimal modifications, contrastive image-text pre-training, and end-to-end detection fine-tuning. Our analysis of the scaling properties of this setup shows that increasing image-level pre-training and model size yield consistent improvements on the downstream detection task. We provide the adaptation strategies and regularizations needed to attain very strong performance on zero-shot text-conditioned and one-shot image-conditioned object detection. Code and models are available on GitHub github.com/google-research/scenic/tree/main/scenic/projects/owl_vit.
更多查看译文
关键词
Open-vocabulary detection,Transformer,Vision transformer,Zero-shot detection,Image-conditioned detection,One-shot object detection,Contrastive learning,Image-text models,Foundation models,CLIP
AI 理解论文
溯源树
样例
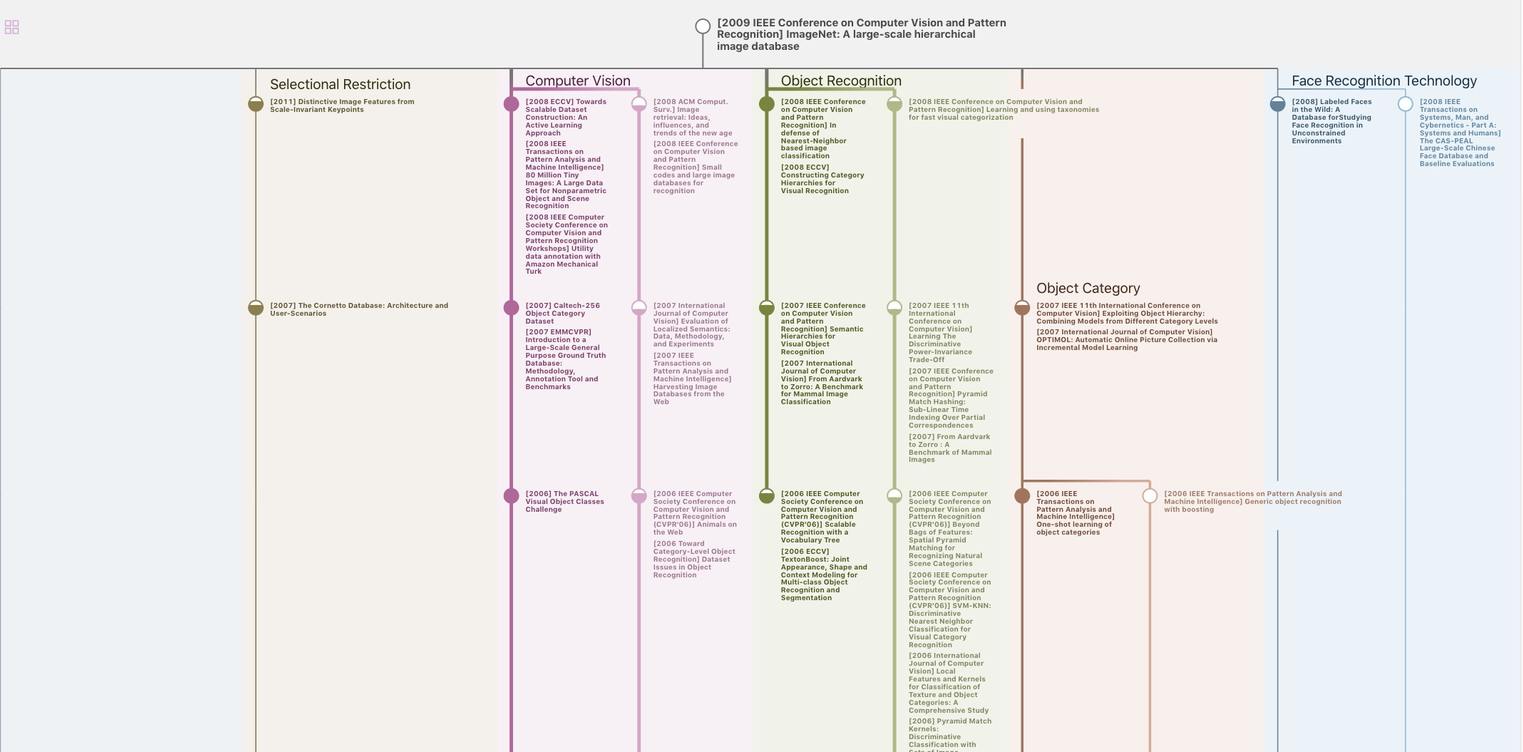
生成溯源树,研究论文发展脉络
Chat Paper
正在生成论文摘要