A Method for Forest Canopy Height Inversion Based on Machine Learning and Feature Mining Using UAVSAR
REMOTE SENSING(2022)
摘要
The mapping of tropical rainforest forest structure parameters plays an important role in biodiversity and carbon stock estimation. The current mechanism models based on PolInSAR for forest height inversion (e.g., the RVoG model) are physical process models, and realistic conditions for model parameterization are often difficult to establish for practical applications, resulting in large forest height estimation errors. As an alternative, machine learning approaches offer the benefit of model simplicity, but these tools provide limited capabilities for interpretation and generalization. To explore the forest height estimation method combining the mechanism model and the empirical model, we utilized UAVSAR multi-baseline PolInSAR L-band data from the AfriSAR project and propose a solution of a mechanism model combined with machine learning. In this paper, two mechanism models were used as controls, the RVoG three-phase method and the RVoG phase-coherence amplitude method. The vertical structure parameters of the forest obtained from the mechanism model were used as the independent variables of the machine learning model. Random forest (RF) and partial least squares (PLS) regression models were used to invert the forest canopy height. Results show that the inversion accuracy of the machine learning method, combined with the mechanism model, is significantly better than that of the single-mechanism model method. The most influential independent variables were penetration depth, volume coherence phase center height, coherence separation, and baseline selection. With the precondition that the cumulative contribution of the independent variables was greater than 90%, the number of independent variables in the two study areas was reduced from 19 to 4, and the accuracy of the RF-RVoG-DEP model was higher than that of the PLS-RVoG-DEP model. For the Lope test area, the R-2 of the RVoG phase coherence amplitude method is 0.723, the RMSE is 8.583 m, and the model bias is -2.431 m; the R-2 of the RVoG three-stage method is 0.775, the RMSE is 7.748, and the bias is 1.120 m, the R-2 of the PLS-RVoG-DEP model is 0.850, the RMSE is 6.320 m, and the bias is 0.002 m; and the R-2 of the RF-RVoG-DEP model is 0.900, the RMSE is 5.154 m, and the bias is -0.061 m. The results for the Pongara test area are consistent with the pattern for the Lope test area. The combined "fusion model" offers a substantial improvement in forest height estimation from the traditional mechanism modeling method.
更多查看译文
关键词
machine learning,mechanism model,RVoG,penetration depth model,PolInSAR,canopy height,forest height inversion
AI 理解论文
溯源树
样例
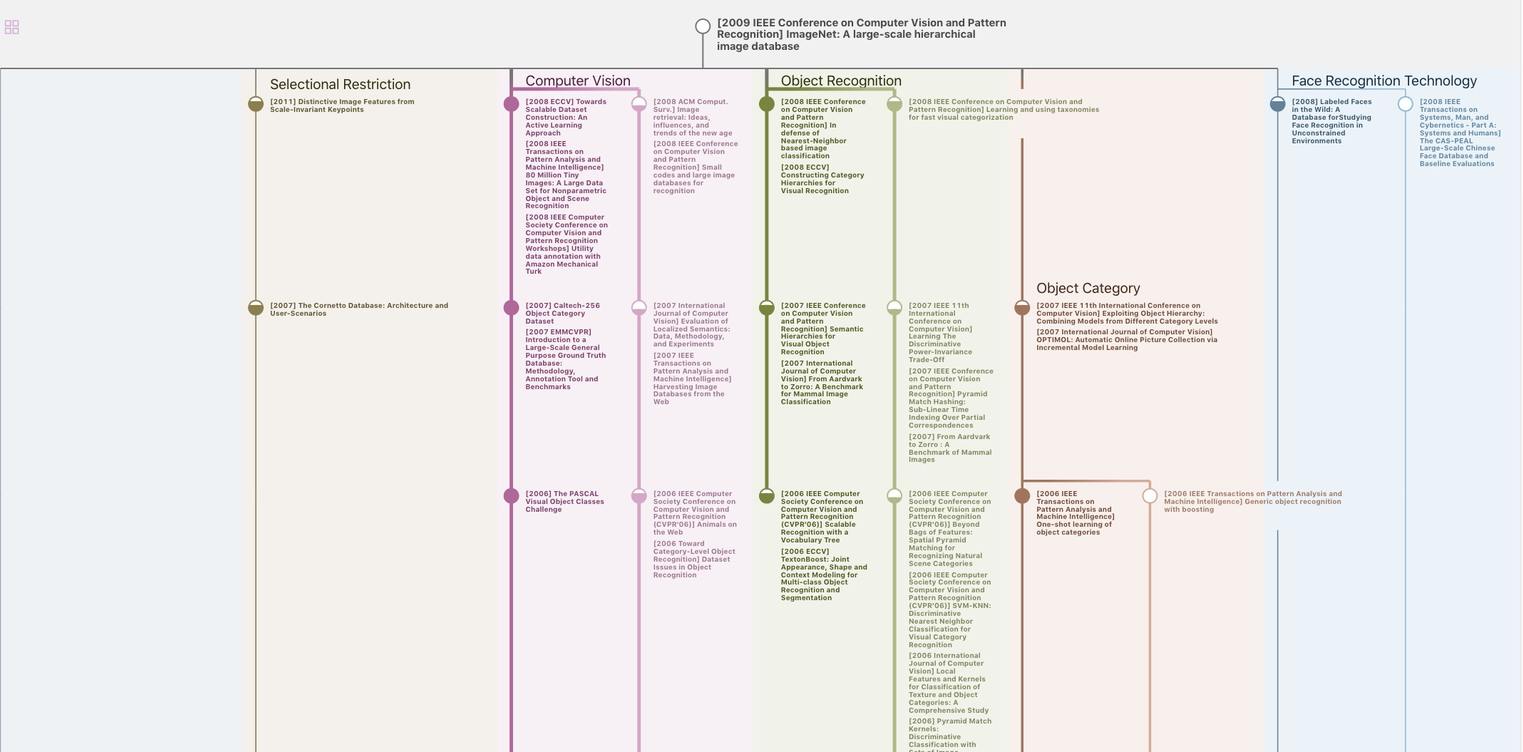
生成溯源树,研究论文发展脉络
Chat Paper
正在生成论文摘要