High-dimensional covariance matrices under dynamic volatility models: asymptotics and shrinkage estimation
Social Science Research Network(2022)
摘要
We study the estimation of the high-dimensional covariance matrix andits eigenvalues under dynamic volatility models. Data under such modelshave nonlinear dependency both cross-sectionally and temporally. We firstinvestigate the empirical spectral distribution (ESD) of the sample covariancematrix under scalar BEKK models and establish conditions under which thelimiting spectral distribution (LSD) is either the same as or different fromthe i.i.d. case. We then propose a time-variation adjusted (TV-adj) sample co-variance matrix and prove that its LSD follows the same Marcenko-Pasturlaw as the i.i.d. case. Based on the asymptotics of the TV-adj sample co-variance matrix, we develop a consistent population spectrum estimator and an asymptotically optimal nonlinear shrinkage estimator of the unconditionalcovariance matrix
更多查看译文
关键词
dynamic volatility models,shrinkage estimation,high-dimensional
AI 理解论文
溯源树
样例
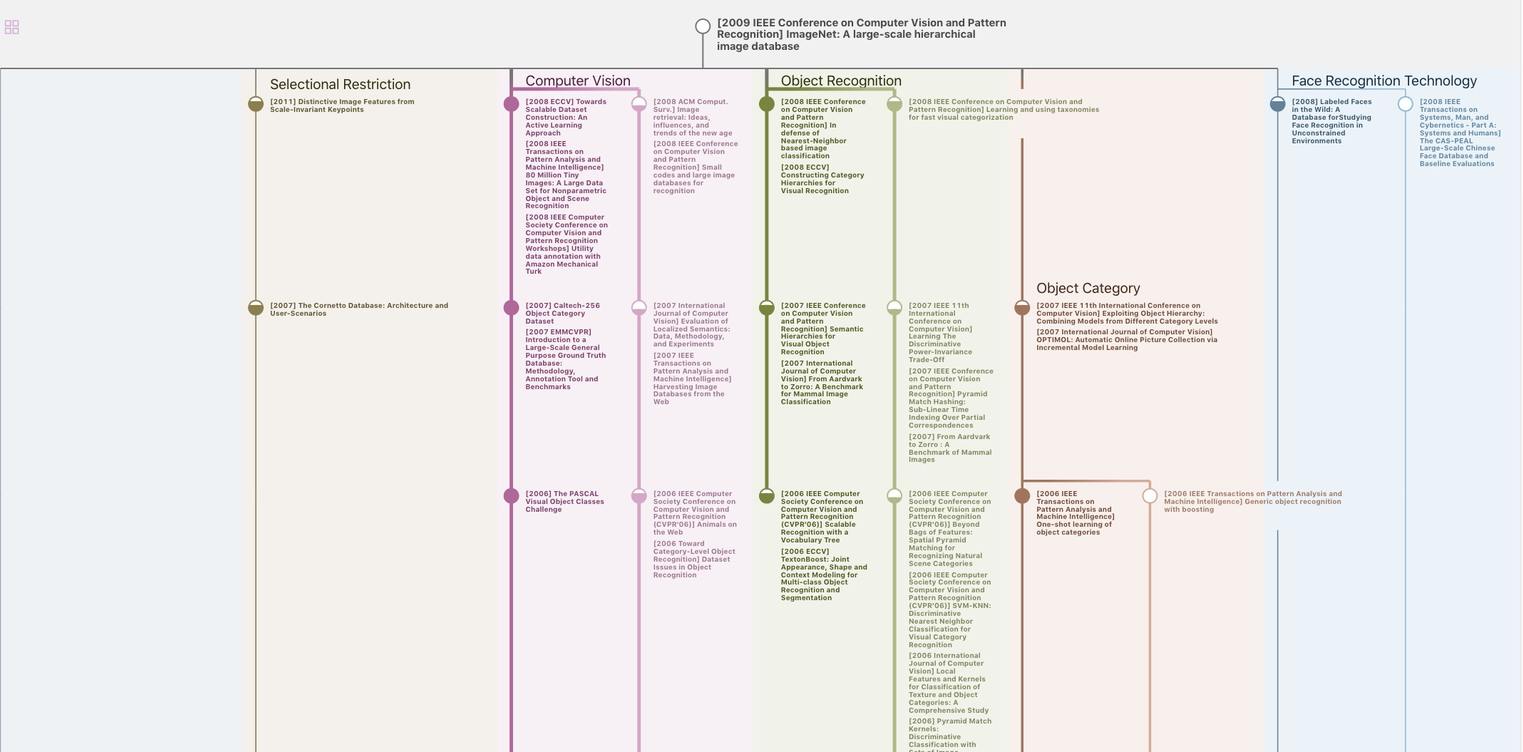
生成溯源树,研究论文发展脉络
Chat Paper
正在生成论文摘要