Rare Yet Popular: Evidence and Implications from Labeled Datasets for Network Anomaly Detection
arxiv(2022)
摘要
Anomaly detection research works generally propose algorithms or end-to-end systems that are designed to automatically discover outliers in a dataset or a stream. While literature abounds concerning algorithms or the definition of metrics for better evaluation, the quality of the ground truth against which they are evaluated is seldom questioned. In this paper, we present a systematic analysis of available public (and additionally our private) ground truth for anomaly detection in the context of network environments, where data is intrinsically temporal, multivariate and, in particular, exhibits spatial properties, which, to the best of our knowledge, we are the first to explore. Our analysis reveals that, while anomalies are, by definition, temporally rare events, their spatial characterization clearly shows some type of anomalies are significantly more popular than others. We find that simple clustering can reduce the need for human labeling by a factor of 2x-10x, that we are first to quantitatively analyze in the wild.
更多查看译文
关键词
labeled datasets,network,rare,detection,popular
AI 理解论文
溯源树
样例
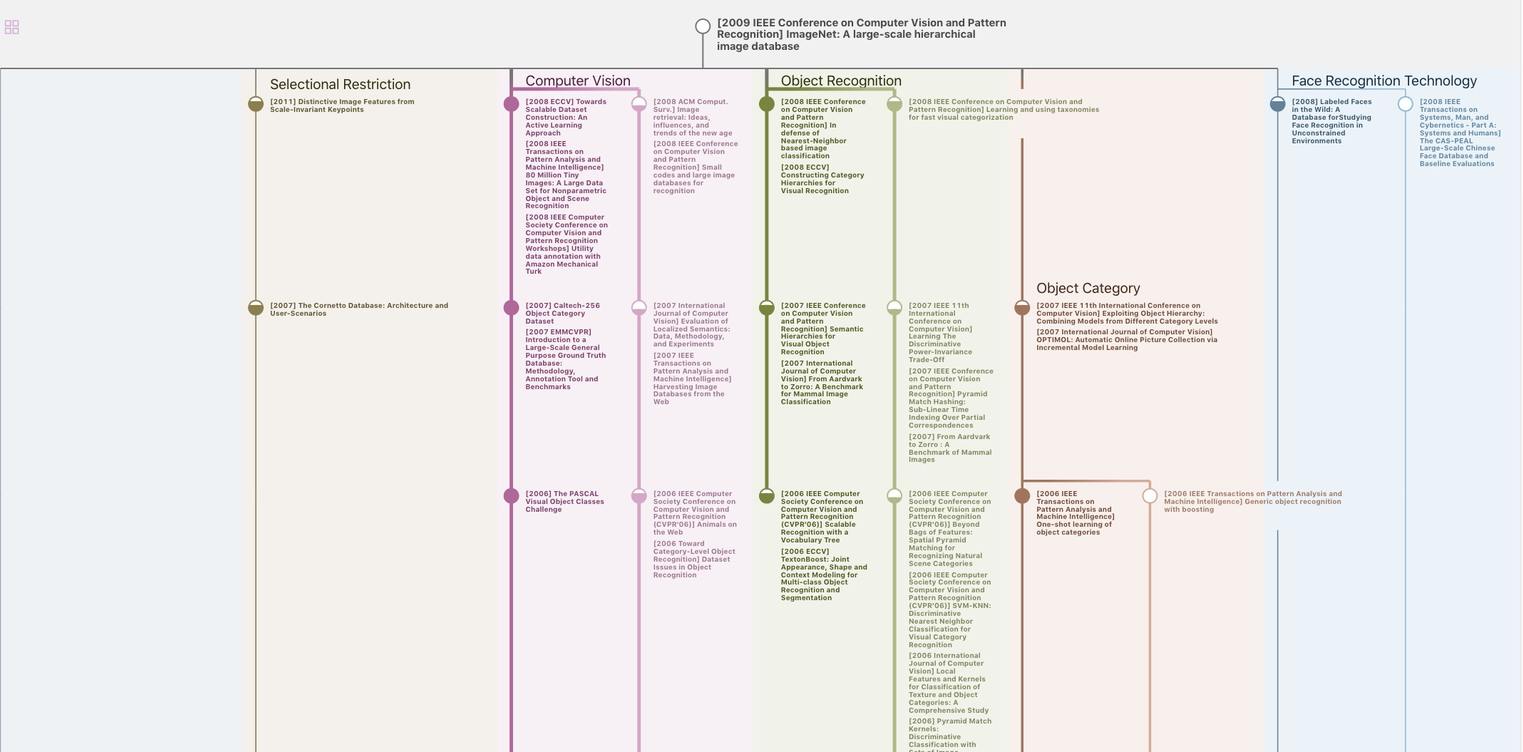
生成溯源树,研究论文发展脉络
Chat Paper
正在生成论文摘要