Deep learning for Lagrangian drift simulation at the sea surface
arxiv(2022)
摘要
We address Lagrangian drift simulation in geophysical dynamics and explore deep learning approaches to overcome known limitations of state-of-the-art model-based and Markovian approaches in terms of computational complexity and error propagation. We introduce a novel architecture, referred to as DriftNet, inspired from the Eulerian Fokker-Planck representation of Lagrangian dynamics. Numerical experiments for Lagrangian drift simulation at the sea surface demonstrates the relevance of DriftNet w.r.t. state-of-the-art schemes. Benefiting from the fully-convolutional nature of Drift-Net, we explore through a neural inversion how to diagnose modelderived velocities w.r.t. real drifter trajectories.
更多查看译文
关键词
Lagrangian drift,Deep learning,Advection scheme,Fokker-Planck,Sea Surface Dynamics
AI 理解论文
溯源树
样例
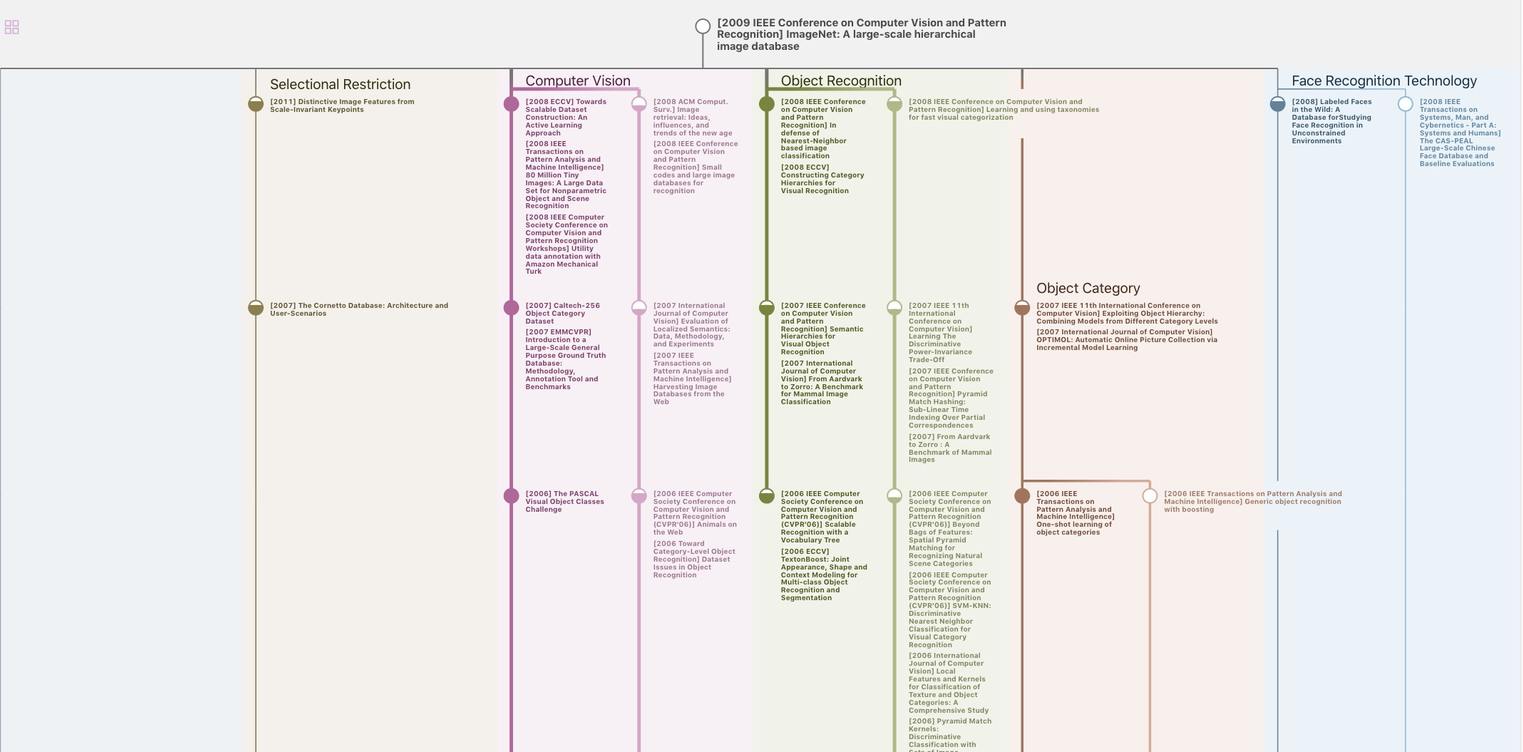
生成溯源树,研究论文发展脉络
Chat Paper
正在生成论文摘要