Severe rail wear detection with rail running band images
COMPUTER-AIDED CIVIL AND INFRASTRUCTURE ENGINEERING(2022)
摘要
Rail wear occurs continuously owing to the rolling contact load of trains and is fundamental for railway operational safety. A point-based manual rail wear inspection cannot satisfy the increasing demand for rapid, low-cost, and continuous monitoring. This paper proposes a depth-plus-region fusion network for detecting rail wear on a running band, which is a collection of wheel-rail interaction traces. The following steps are involved in the proposed method. (i) A depth map estimated by a modified MiDaS model is utilized as guidance for exploiting the depth information of the running band for rail wear detection. (ii) The running band of a rail is segmented and extracted from images using an improved mask region-based convolutional neural network that uses the scale and ratio information to perform instance segmentation of the running band images. (iii) A two-channel attention-fusion network that classifies rail wear is constructed. In this study, we collected real-world running band images and rail wear-related data to validate our approach using a high-accuracy rail-profile measurement tool. The case-study results demonstrated that the proposed method can rapidly and accurately detect rail wear under different ambient light conditions. Moreover, the recall rate of severe wear detection was 84.21%.
更多查看译文
关键词
severe rail,band images,detection
AI 理解论文
溯源树
样例
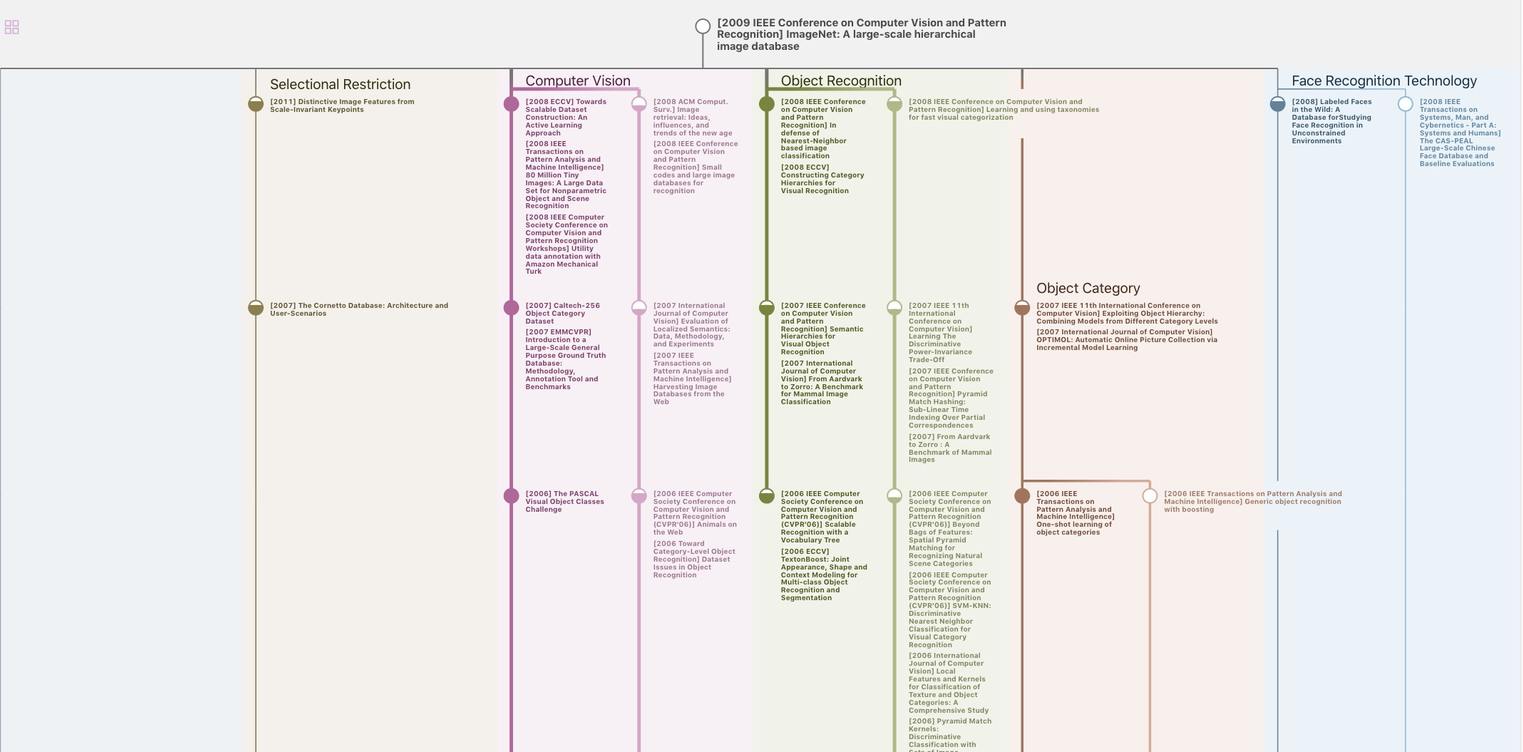
生成溯源树,研究论文发展脉络
Chat Paper
正在生成论文摘要