Machine Learning for Software Engineering: A Tertiary Study
arxiv(2022)
摘要
Machine learning (ML) techniques increase the effectiveness of software engineering (SE) lifecycle activities. We systematically collected, quality-assessed, summarized, and categorized 83 reviews in ML for SE published between 2009-2022, covering 6,117 primary studies. The SE areas most tackled with ML are software quality and testing, while human-centered areas appear more challenging for ML. We propose a number of ML for SE research challenges and actions including: conducting further empirical validation and industrial studies on ML; reconsidering deficient SE methods; documenting and automating data collection and pipeline processes; reexamining how industrial practitioners distribute their proprietary data; and implementing incremental ML approaches.
更多查看译文
关键词
Tertiary study,machine learning,software engineering,systematic literature review
AI 理解论文
溯源树
样例
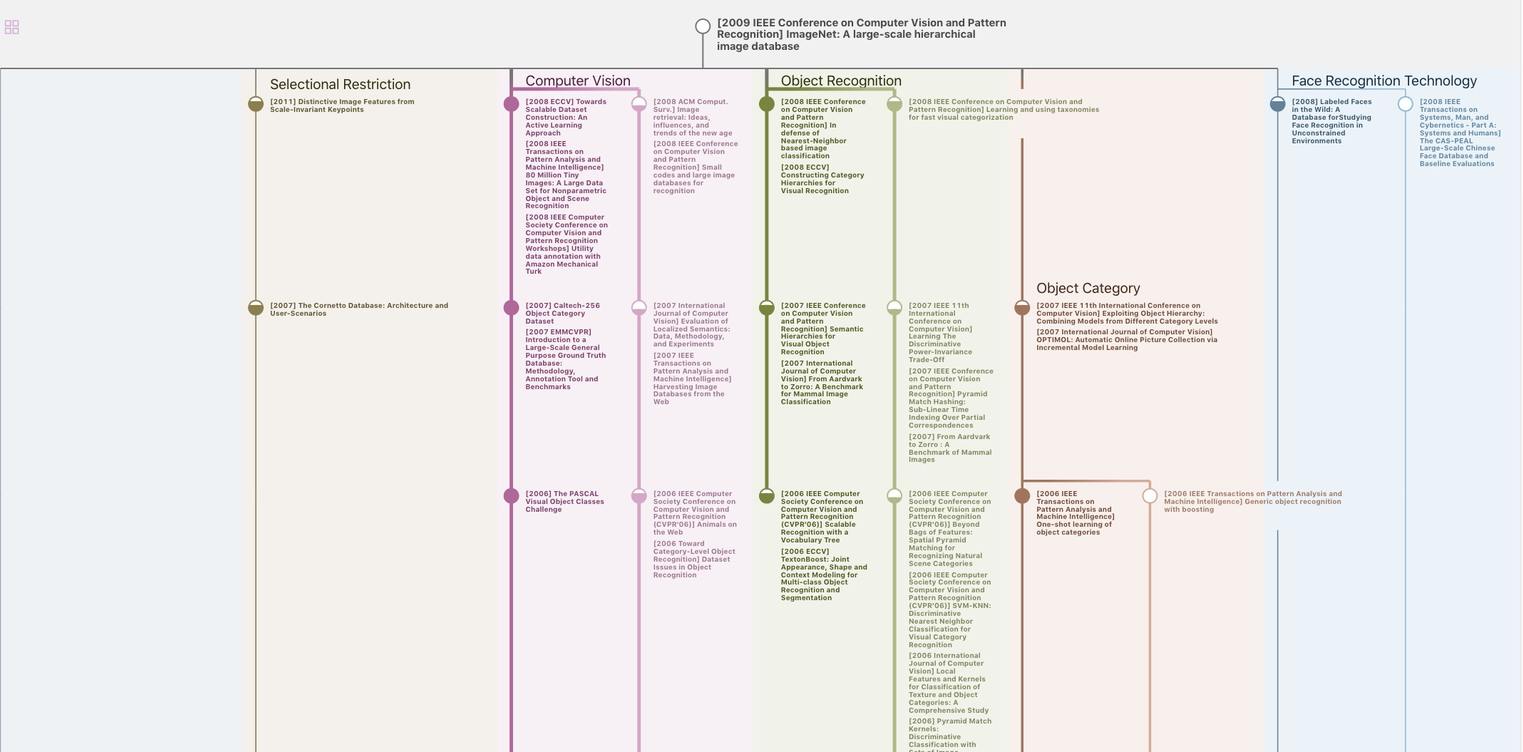
生成溯源树,研究论文发展脉络
Chat Paper
正在生成论文摘要