Reflect, Not Reflex: Inference-Based Common Ground Improves Dialogue Response Quality
emnlp 2022(2022)
Abstract
Human communication relies on common ground (CG), the mutual knowledge and beliefs shared by participants, to produce coherent and interesting conversations. In this paper, we demonstrate that current response generation (RG) models produce generic and dull responses in dialogues because they act reflexively, failing to explicitly model CG, both due to the lack of CG in training data and the standard RG training procedure. We introduce Reflect, a dataset that annotates dialogues with explicit CG (materialized as inferences approximating shared knowledge and beliefs) and solicits 9k diverse human-generated responses each following one common ground. Using Reflect, we showcase the limitations of current dialogue data and RG models: less than half of the responses in current data are rated as high quality (sensible, specific, and interesting) and models trained using this data have even lower quality, while most Reflect responses are judged high quality. Next, we analyze whether CG can help models produce better-quality responses by using Reflect CG to guide RG models. Surprisingly, we find that simply prompting GPT3 to "think" about CG generates 30% more quality responses, showing promising benefits to integrating CG into the RG process.
MoreTranslated text
Key words
dialogue
AI Read Science
Must-Reading Tree
Example
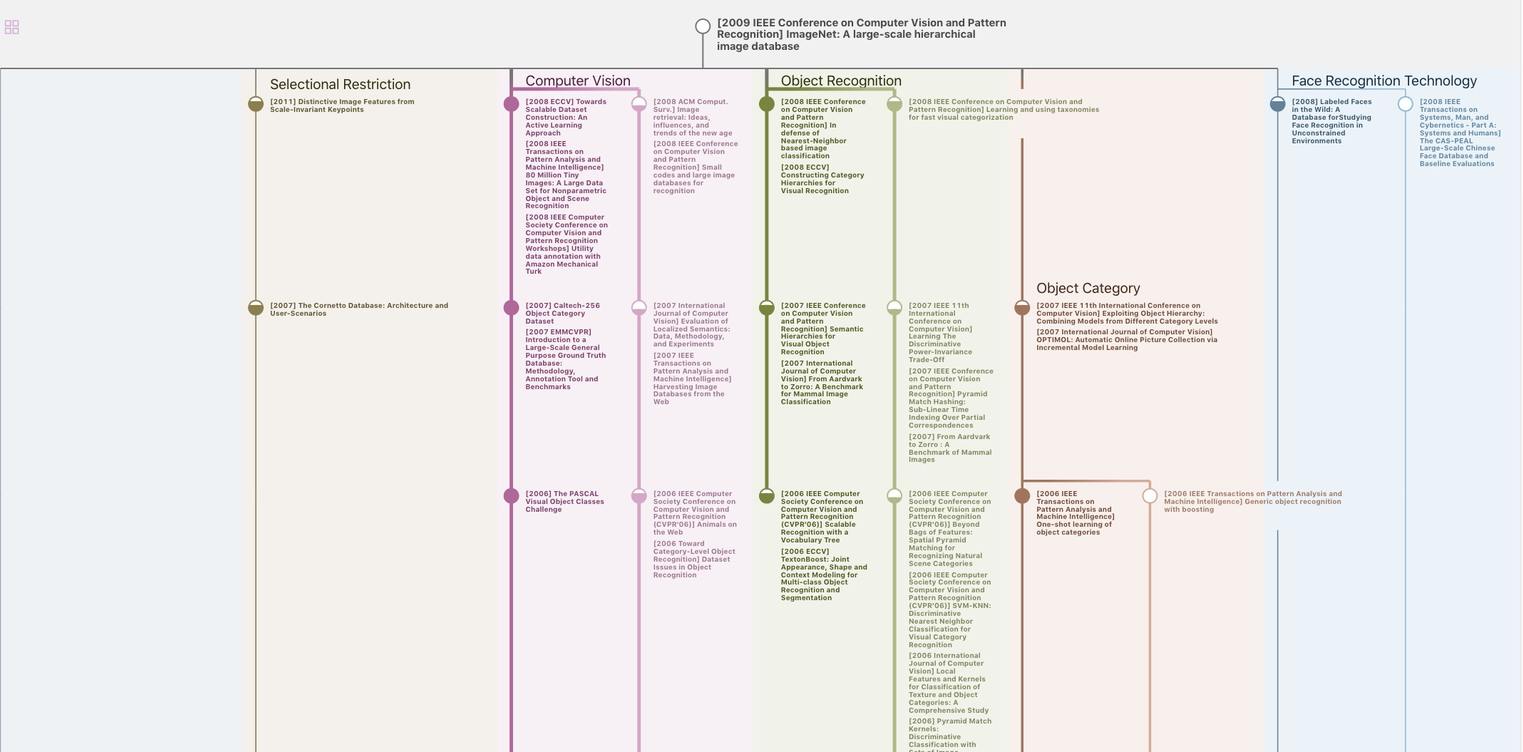
Generate MRT to find the research sequence of this paper
Chat Paper
Summary is being generated by the instructions you defined