Prompt tuning for parameter-efficient medical image segmentation
MEDICAL IMAGE ANALYSIS(2024)
摘要
Neural networks pre -trained on a self -supervision scheme have become the standard when operating in data rich environments with scarce annotations. As such, fine-tuning a model to a downstream task in a parameterefficient but effective way, e.g. for a new set of classes in the case of semantic segmentation, is of increasing importance. In this work, we propose and investigate several contributions to achieve a parameter -efficient but effective adaptation for semantic segmentation on two medical imaging datasets. Relying on the recently popularized prompt tuning approach, we provide a prompt -able UNETR (PUNETR) architecture, that is frozen after pre -training, but adaptable throughout the network by class -dependent learnable prompt tokens. We pre -train this architecture with a dedicated dense self -supervision scheme based on assignments to online generated prototypes (contrastive prototype assignment, CPA) of a student teacher combination. Concurrently, an additional segmentation loss is applied for a subset of classes during pre -training, further increasing the effectiveness of leveraged prompts in the fine-tuning phase. We demonstrate that the resulting method is able to attenuate the gap between fully fine-tuned and parameter -efficiently adapted models on CT imaging datasets. To this end, the difference between fully fine-tuned and prompt -tuned variants amounts to 7.81 pp for the TCIA/BTCV dataset as well as 5.37 and 6.57 pp for subsets of the TotalSegmentator dataset in the mean Dice Similarity Coefficient (DSC, in %) while only adjusting prompt tokens, corresponding to 0.51% of the pre -trained backbone model with 24.4M frozen parameters. The code for this work is available on https://github.com/marcdcfischer/PUNETR.
更多查看译文
关键词
Self-supervision,Semi-supervision,Prompt tuning,Semantic segmentation,Transformer,Self-attention
AI 理解论文
溯源树
样例
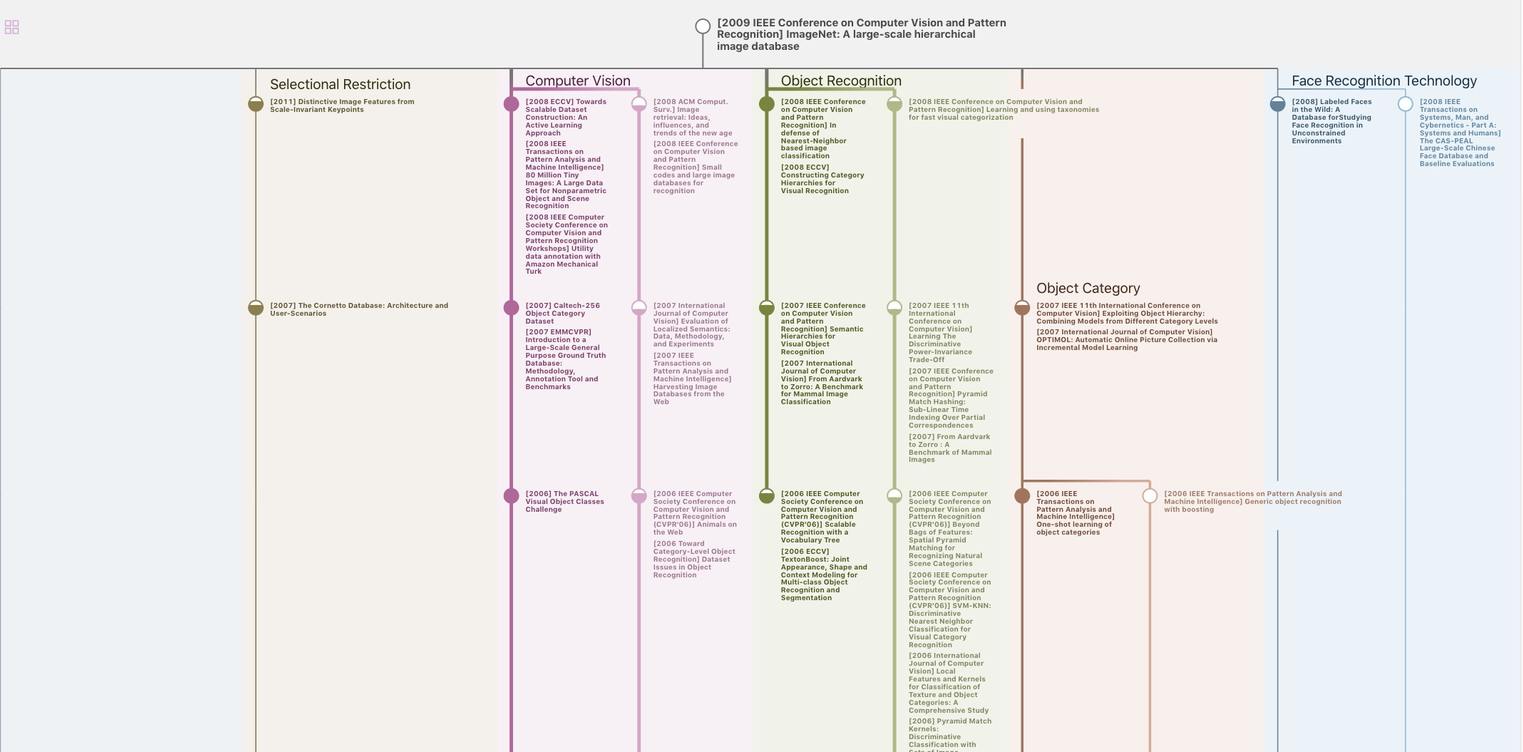
生成溯源树,研究论文发展脉络
Chat Paper
正在生成论文摘要