FlowSRNet: A multi-scale integration network for super-resolution reconstruction of fluid flows
Physics of Fluids(2022)
摘要
A wide range of research problems in physics and engineering involve the acquisition of high-resolution data. Recently, deep learning has proved to be a prospective technique for super-resolution (SR) reconstruction of fluid flows. General deep learning methods develop temporal multi-branch networks to improve SR accuracy while ignoring computational efficiency. Further, the generalization ability of the deep learning model in different fluid flow scenarios is still an unstudied issue. In this article, we propose an efficient multi-scale integration network called FlowSRNet to reconstruct the high-resolution flow fields. Specifically, we elaborately design a lightweight multi-scale aggregation block (LMAB) to capture multi-scale features of fluid data, which contains a parallel cascading architecture (PCA) and feature aggregation module (FAM). The residual backbone of FlowSRNet is built by cascading the lightweight multi-scale aggregation blocks (LMABs) (cascaded blocks number N=8) in a serial manner. Also, we present a small architecture LiteFlowSRNet (cascaded blocks number N=2) for comparison. In addition, a corresponding SR dataset is constructed to train and test the proposed model, which contains different kinds of fluid flows. Finally, extensive experiments are performed on different fluid data to evaluate the performance of the proposed model. The results demonstrate that our approach achieve state-of-the-art SR performance on various fluid flow fields. Notably, our method enjoys merit of lightweight, which facilitates the development of the complicated calculation in computational fluid dynamics.
更多查看译文
关键词
fluid flowsrnet,multi-scale,super-resolution
AI 理解论文
溯源树
样例
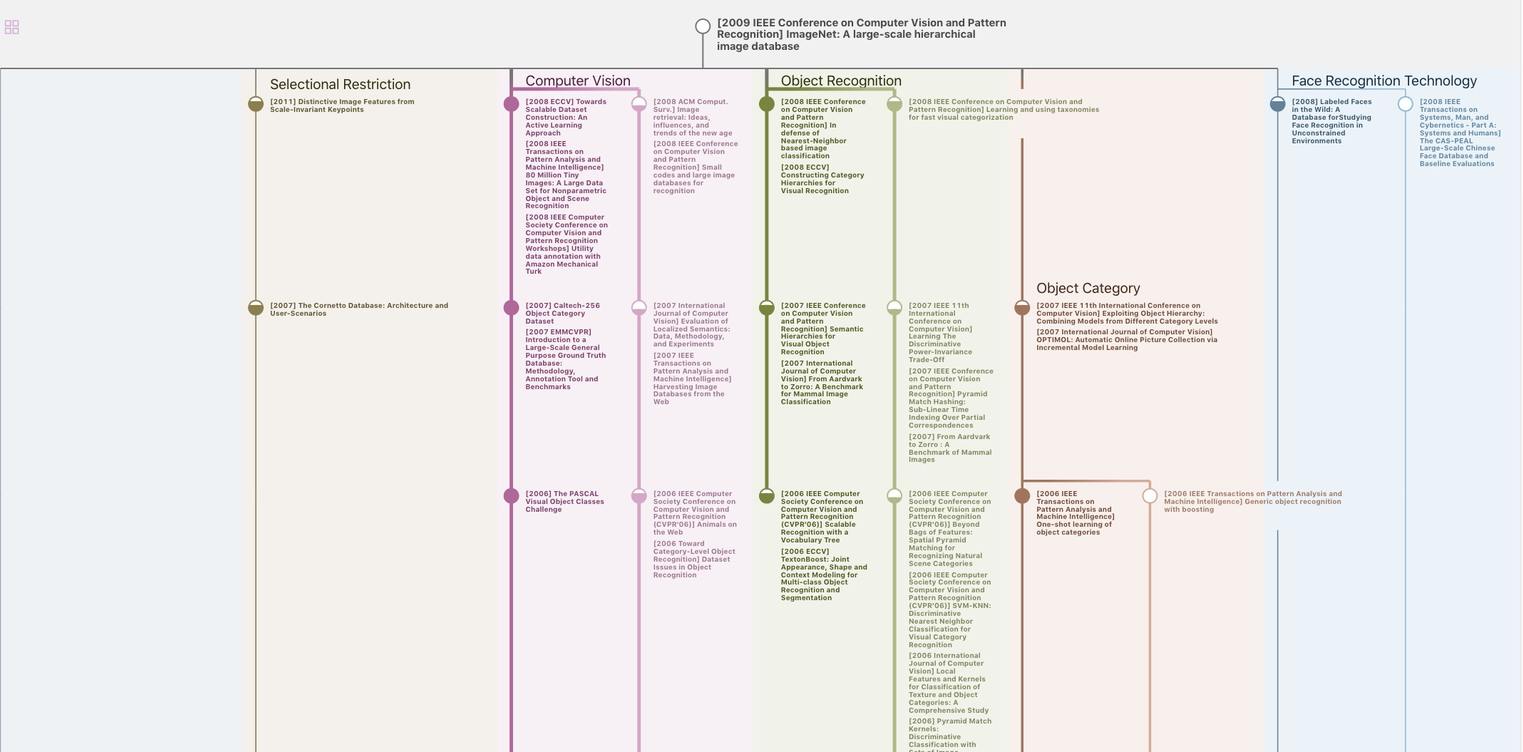
生成溯源树,研究论文发展脉络
Chat Paper
正在生成论文摘要