The Anatomy of Out-of-Sample Forecasting Accuracy
Social Science Research Network(2022)
摘要
: We introduce the performance-based Shapley value (PBSV) to measure the contributions of individual predictors to the out-of-sample loss for time-series forecasting models. Our new metric allows a researcher to anatomize out-of-sample forecasting accuracy, thereby providing valuable information for interpreting time-series forecasting models. The PBSV is model agnostic—so it can be applied to any forecasting model, including “black box” models in machine learning, and it can be used for any loss function. We also develop the TS-Shapley-VI, a version of the conventional Shapley value that gauges the importance of predictors for explaining the in-sample predictions in the entire sequence of fitted models that generates the time series of out-of-sample forecasts. We then propose the model accordance score to compare predictor ranks based on the TS-Shapley-VI and PBSV, thereby linking the predictors’ in-sample importance to their contributions to out-of-sample forecasting accuracy. We illustrate our metrics in an application forecasting US inflation.
更多查看译文
关键词
Prediction Accuracy,Forecasting,Demand Forecasting,Exponential Smoothing,Forecast Combination
AI 理解论文
溯源树
样例
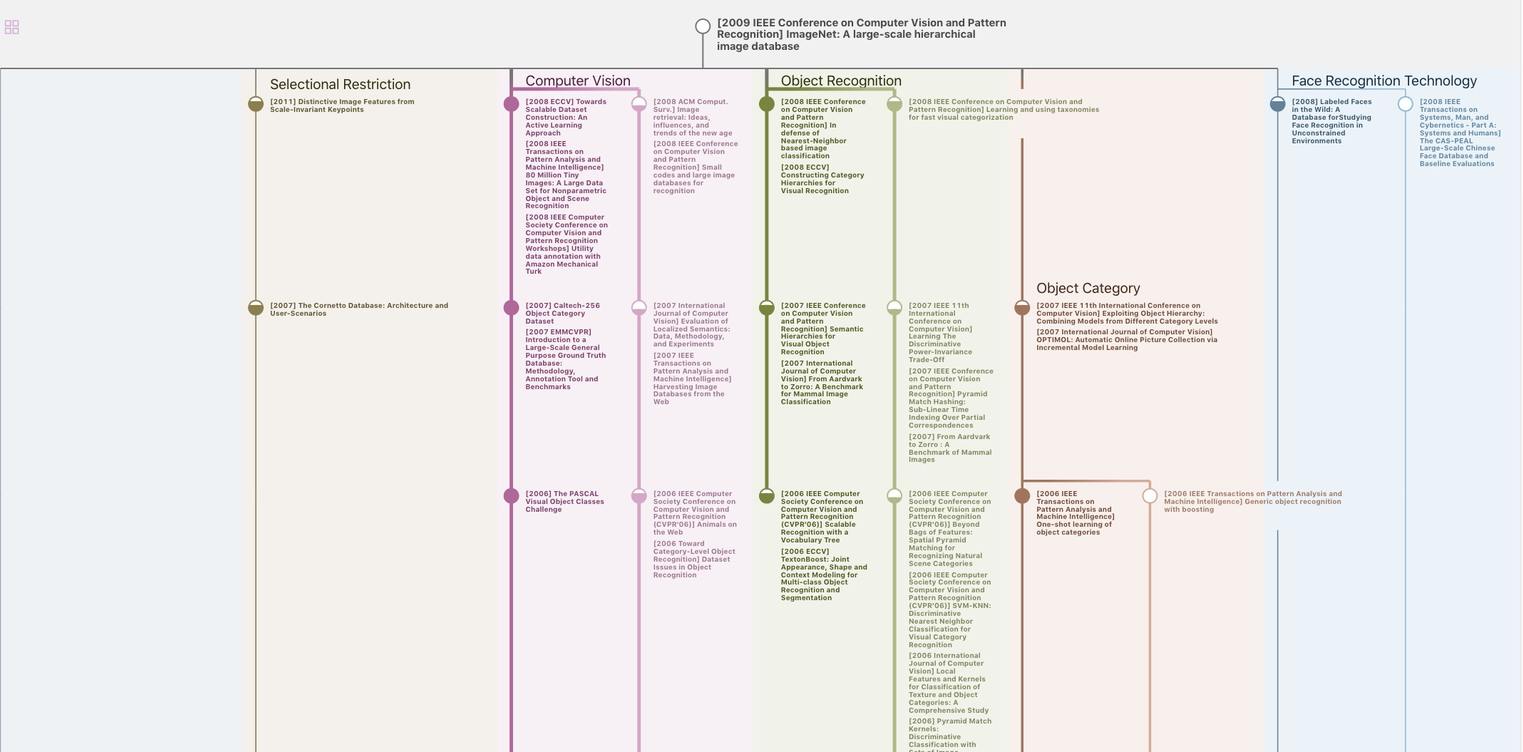
生成溯源树,研究论文发展脉络
Chat Paper
正在生成论文摘要