A Novel Self-supervised Few-shot Network Intrusion Detection Method.
WASA (1)(2022)
Abstract
Supervised models for network intrusion detection usually rely on many training samples, but the annotation costs are very high. Unlabeled network traffic data is relatively easy to obtain. However, there are only a few methods to utilize these unlabeled data adequately. We propose a novel self-supervised few-shot network intrusion detection method to address the above problems. The method consists of two models: a) network traffic representation model and b) network intrusion detection model. First, the network traffic representation model uses unlabeled network traffic data through self-supervised learning to obtain network traffic representations, which will benefit the training of network intrusion detection model. Then, the shared layers of the network traffic representation model are transferred to the network intrusion detection model and frozen. Finally, a few training samples are used to fine-tune the network intrusion detection model, and we can obtain a model with good generalization. However, self-supervised learning of the network traffic representation model requires a method for generating labels from network traffic. Therefore, we propose a novel method to generate labels based on discrete features of network traffic. Experiments show that our proposed method has better performance than other network intrusion detection models with few-shot. On NSL-KDD, only 200 labeled samples are needed to achieve 95.2% accuracy.
MoreTranslated text
Key words
Self-supervised learning,Network intrusion detection,Generating labels,Few-shot learning
AI Read Science
Must-Reading Tree
Example
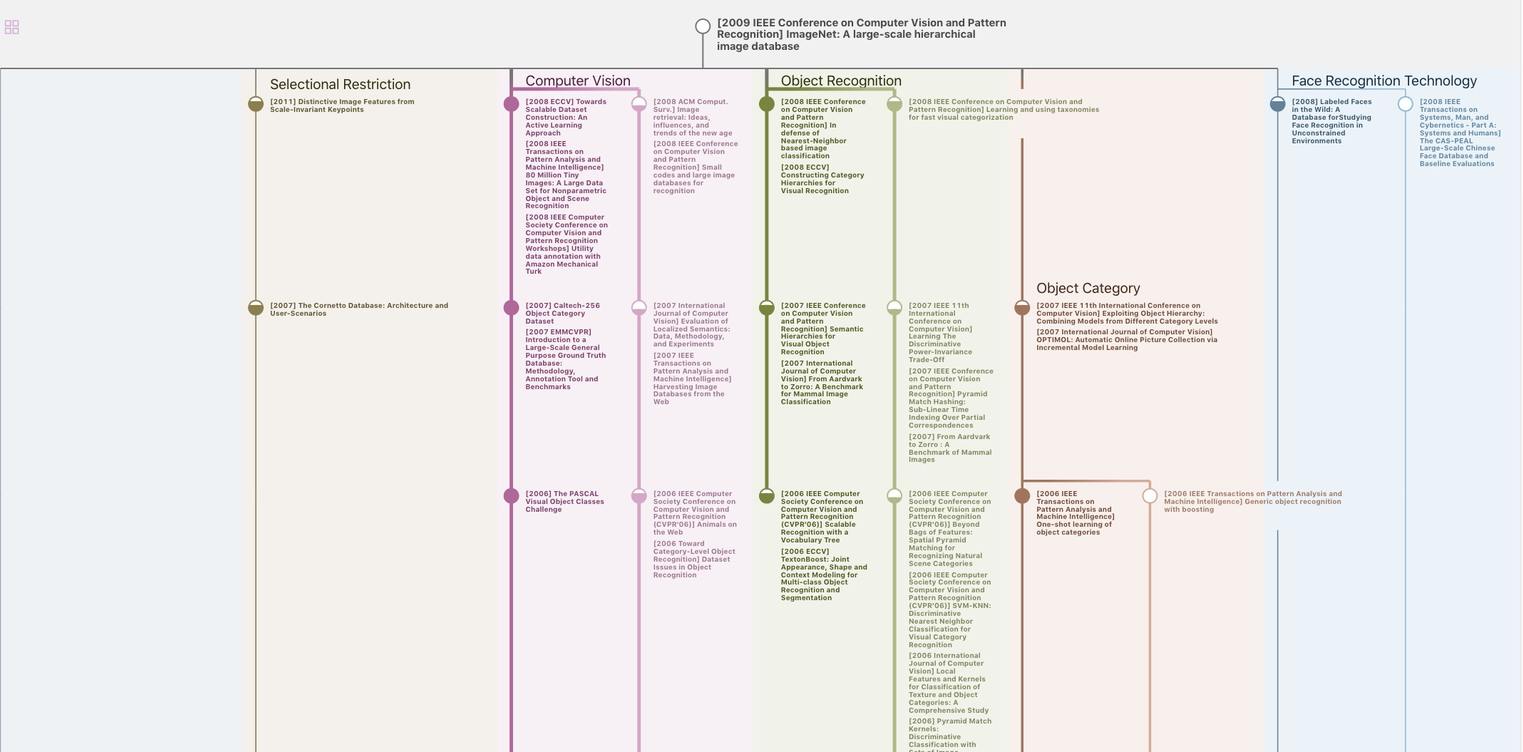
Generate MRT to find the research sequence of this paper
Chat Paper
Summary is being generated by the instructions you defined