Deep learning of spatial densities in inhomogeneous correlated quantum systems
arxiv(2022)
摘要
Machine learning has made important headway in helping to improve the treatment of quantum many-body systems. A domain of particular relevance are correlated inhomogeneous systems. What has been missing so far is a general, scalable deep-learning approach that would enable the rapid prediction of spatial densities for strongly correlated systems in arbitrary potentials. In this work, we present a straightforward scheme, where we learn to predict densities using convolutional neural networks trained on random potentials. While we demonstrate this approach in 1D and 2D lattice models using data from numerical techniques like Quantum Monte Carlo, it is directly applicable as well to training data obtained from experimental quantum simulators. We train networks that can predict the densities of multiple observables simultaneously and that can predict for a whole class of many-body lattice models, for arbitrary system sizes. We show that our approach can handle well the interplay of interference and interactions and the behaviour of models with phase transitions in inhomogeneous situations, and we also illustrate the ability to solve inverse problems, finding a potential for a desired density.
更多查看译文
关键词
inhomogeneous correlated quantum,spatial densities,deep learning
AI 理解论文
溯源树
样例
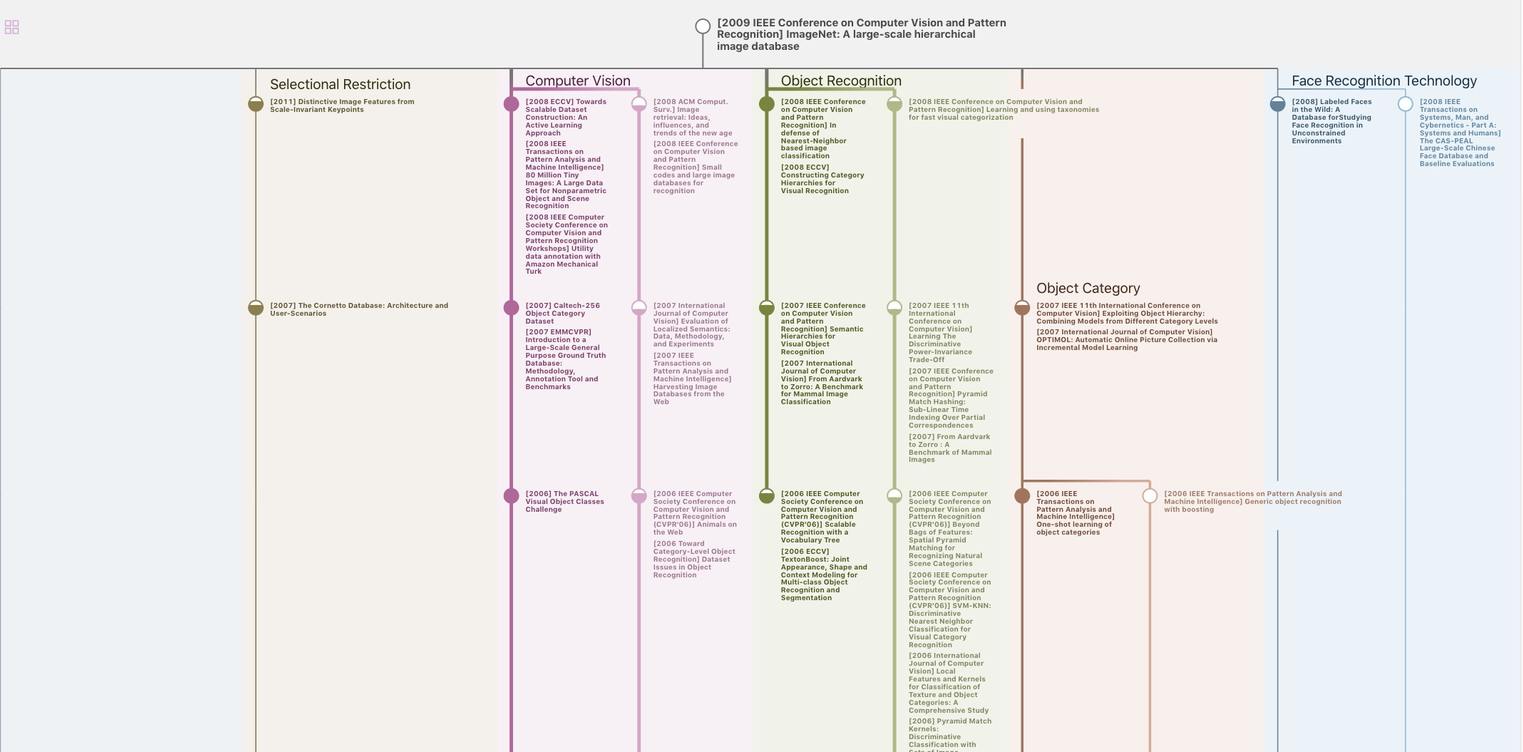
生成溯源树,研究论文发展脉络
Chat Paper
正在生成论文摘要