Parameter-Efficient Tuning on Layer Normalization for Pre-trained Language Models
arxiv(2022)
摘要
Conventional fine-tuning encounters increasing difficulties given the size of current Pre-trained Language Models, which makes parameter-efficient tuning become the focal point of frontier research. Previous methods in this field add tunable adapters into MHA or/and FFN of Transformer blocks to enable PLMs achieve transferability. However, as an important part of Transformer architecture, the power of layer normalization for parameter-efficent tuning is ignored. In this paper, we first propose LN-tuning, by tuning the gain and bias term of Layer Normalization module with only 0.03\% parameters, which is of high time-efficency and significantly superior to baselines which are less than 0.1\% tunable parameters. Further, we study the unified framework of combining LN-tuning with previous ones and we find that: (1) the unified framework of combining prefix-tuning, the adapter-based method working on MHA, and LN-tuning achieves SOTA performance. (2) unified framework which tunes MHA and LayerNorm simultaneously can get performance improvement but those which tune FFN and LayerNorm simultaneous will cause performance decrease. Ablation study validates LN-tuning is of no abundant parameters and gives a further understanding of it.
更多查看译文
关键词
layer normalization,tuning,language,parameter-efficient,pre-trained
AI 理解论文
溯源树
样例
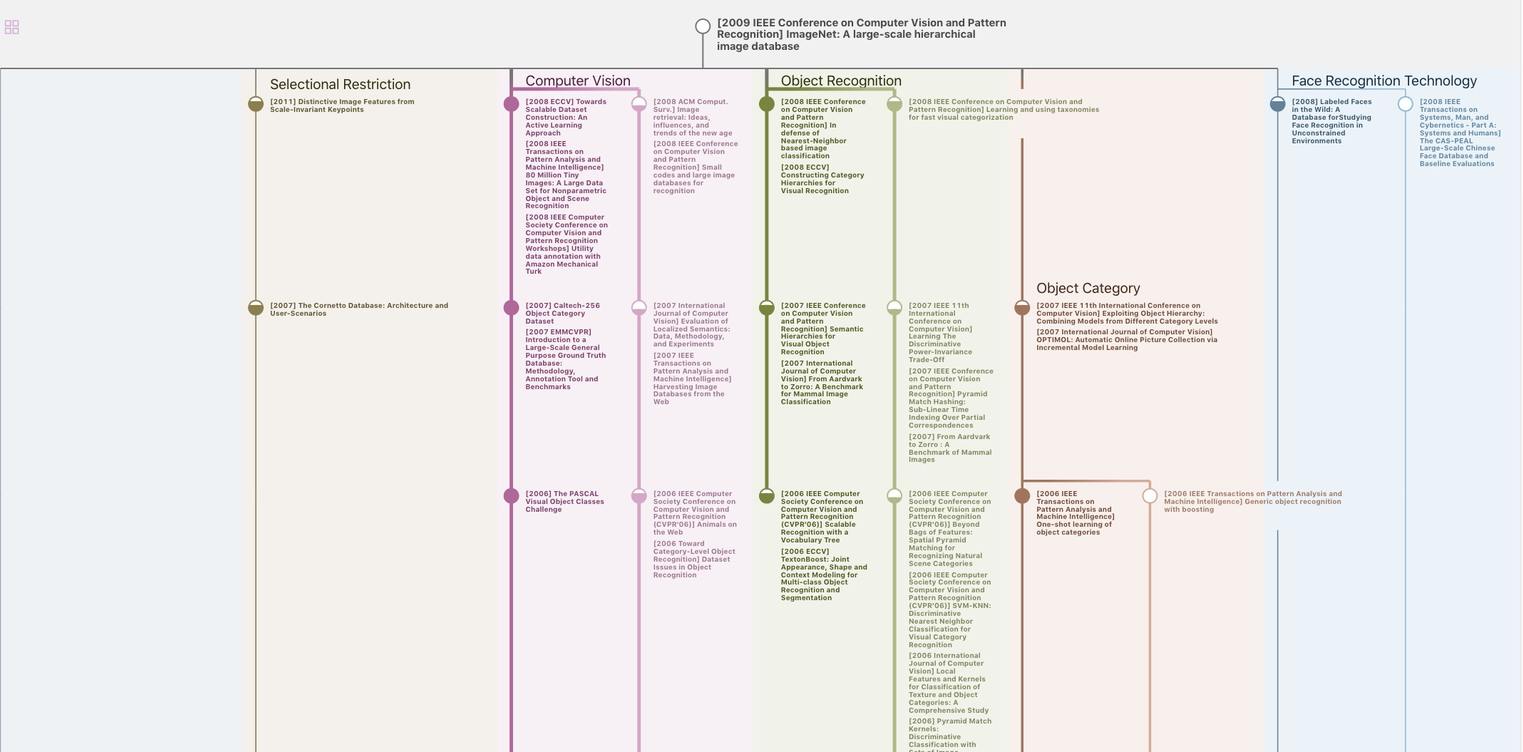
生成溯源树,研究论文发展脉络
Chat Paper
正在生成论文摘要