DAESTB: inferring associations of small molecule-miRNA via a scalable tree boosting model based on deep autoencoder
Briefings Bioinform.(2022)
Abstract
MicroRNAs (miRNAs) are closely related to a variety of human diseases, not only regulating gene expression, but also having an important role in human life activities and being viable targets of small molecule drugs for disease treatment. Current computational techniques to predict the potential associations between small molecule and miRNA are not that accurate. Here, we proposed a new computational method based on a deep autoencoder and a scalable tree boosting model (DAESTB), to predict associations between small molecule and miRNA. First, we constructed a high-dimensional feature matrix by integrating small molecule-small molecule similarity, miRNA-miRNA similarity and known small molecule-miRNA associations. Second, we reduced feature dimensionality on the integrated matrix using a deep autoencoder to obtain the potential feature representation of each small molecule-miRNA pair. Finally, a scalable tree boosting model is used to predict small molecule and miRNA potential associations. The experiments on two datasets demonstrated the superiority of DAESTB over various state-of-the-art methods. DAESTB achieved the best AUC value. Furthermore, in three case studies, a large number of predicted associations by DAESTB are confirmed with the public accessed literature. We envision that DAESTB could serve as a useful biological model for predicting potential small molecule-miRNA associations.
MoreTranslated text
Key words
microRNA,small molecule,association prediction,deep autoencoder,scalable tree boosting
AI Read Science
Must-Reading Tree
Example
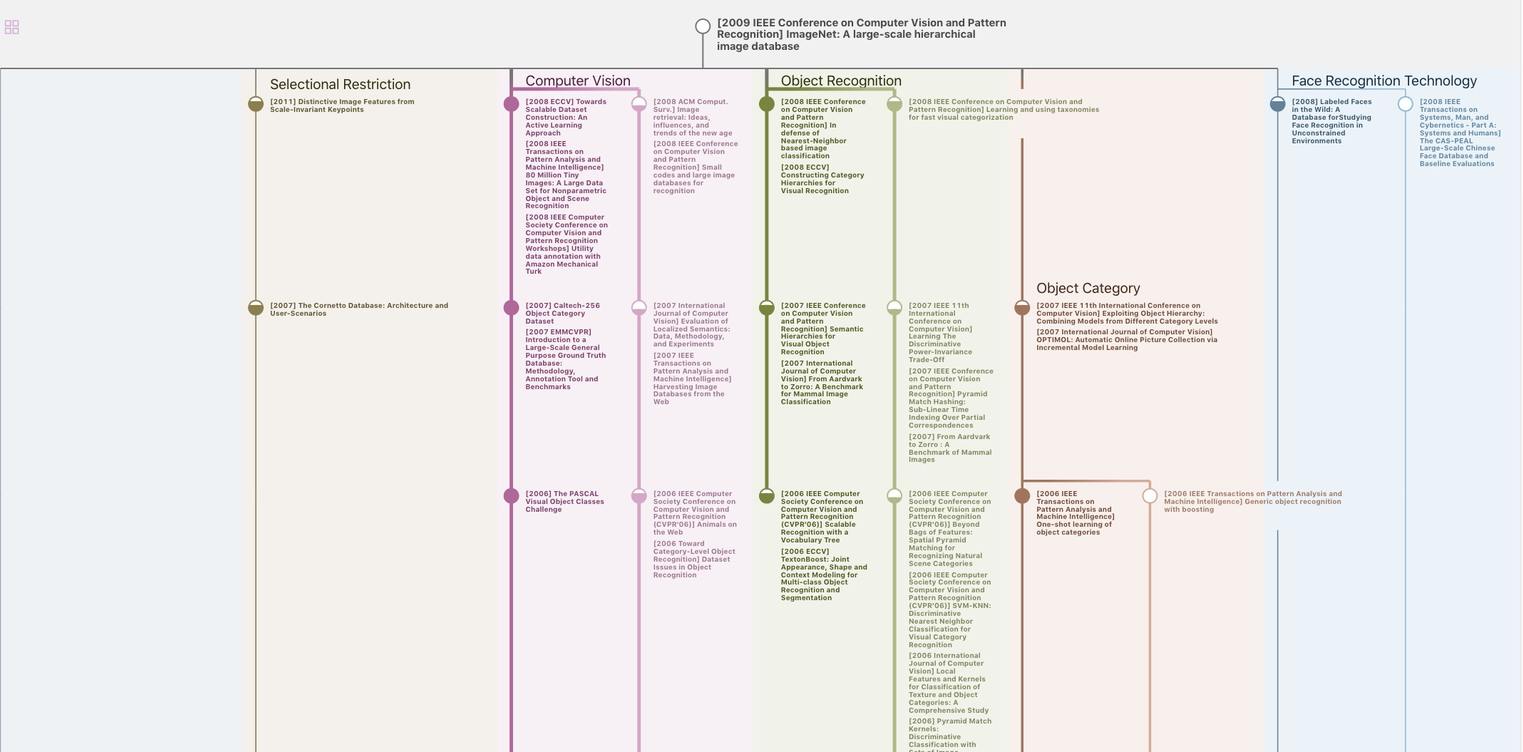
Generate MRT to find the research sequence of this paper
Chat Paper
Summary is being generated by the instructions you defined