A semiparametric joint model for cluster size and subunit-specific interval-censored outcomes.
Biometrics(2022)
摘要
Clustered data frequently arise in biomedical studies, where observations, or subunits, measured within a cluster are associated. The cluster size is said to be informative, if the outcome variable is associated with the number of subunits in a cluster. In most existing work, the informative cluster size issue is handled by marginal approaches based on within-cluster resampling, or cluster-weighted generalized estimating equations. Although these approaches yield consistent estimation of the marginal models, they do not allow estimation of within-cluster associations and are generally inefficient. In this paper, we propose a semiparametric joint model for clustered interval-censored event time data with informative cluster size. We use a random effect to account for the association among event times of the same cluster as well as the association between event times and the cluster size. For estimation, we propose a sieve maximum likelihood approach and devise a computationally-efficient expectation-maximization algorithm for implementation. The estimators are shown to be strongly consistent, with the Euclidean components being asymptotically normal and achieving semiparametric efficiency. Extensive simulation studies are conducted to evaluate the finite-sample performance, efficiency and robustness of the proposed method. We also illustrate our method via application to a motivating periodontal disease dataset.
更多查看译文
关键词
EM algorithm,dental study,informative cluster size,random effect model,sieve estimation
AI 理解论文
溯源树
样例
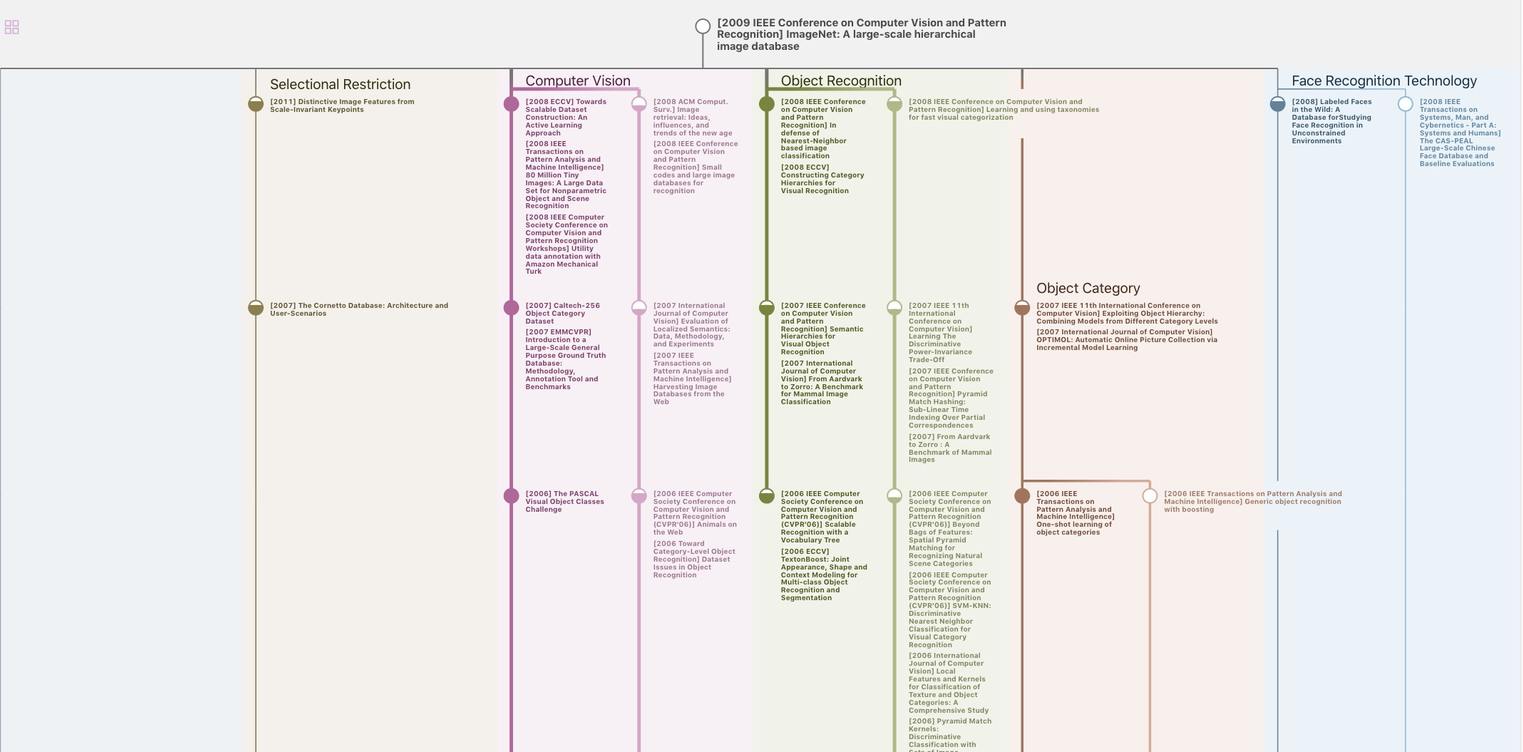
生成溯源树,研究论文发展脉络
Chat Paper
正在生成论文摘要