Evaluating the Optimal Number of Clusters to Identify Similar Gene Expression Patterns During Erythropoiesis
2022 International Conference on Computer, Information and Telecommunication Systems (CITS)(2022)
摘要
Haematopoietic stem cells (HSC) are differentiated into red blood cells (erythrocytes) through a process called Erythropoiesis. During this process, the genes undergo global gene expression changes to reflect the present developmental stage. Unsupervised clustering aims at highlighting the co-expressed genes that share similar expression profiles. Some clustering algorithms, like the well-known and most commonly used K-means, need the number of clusters as input in order to group the data based on similarity measurements. Determining a sufficient number of clusters is not a straightforward task and might be tricky. Furthermore, the quality of the obtained clusters depends on how many clusters were used. In this study, three cluster validation metrics; Silhouette Score, Calinski Harabaz Index, and DaviesBouldin Score were used to evaluate the clusters obtained from the different clustering algorithms applied. For the data of Erythropoiesis, two clusters were identified as sufficient.
更多查看译文
关键词
Clustering,Gene Expression,K-means,Hierarchical Clustering,Mean Shift,Gaussian Mixture Model
AI 理解论文
溯源树
样例
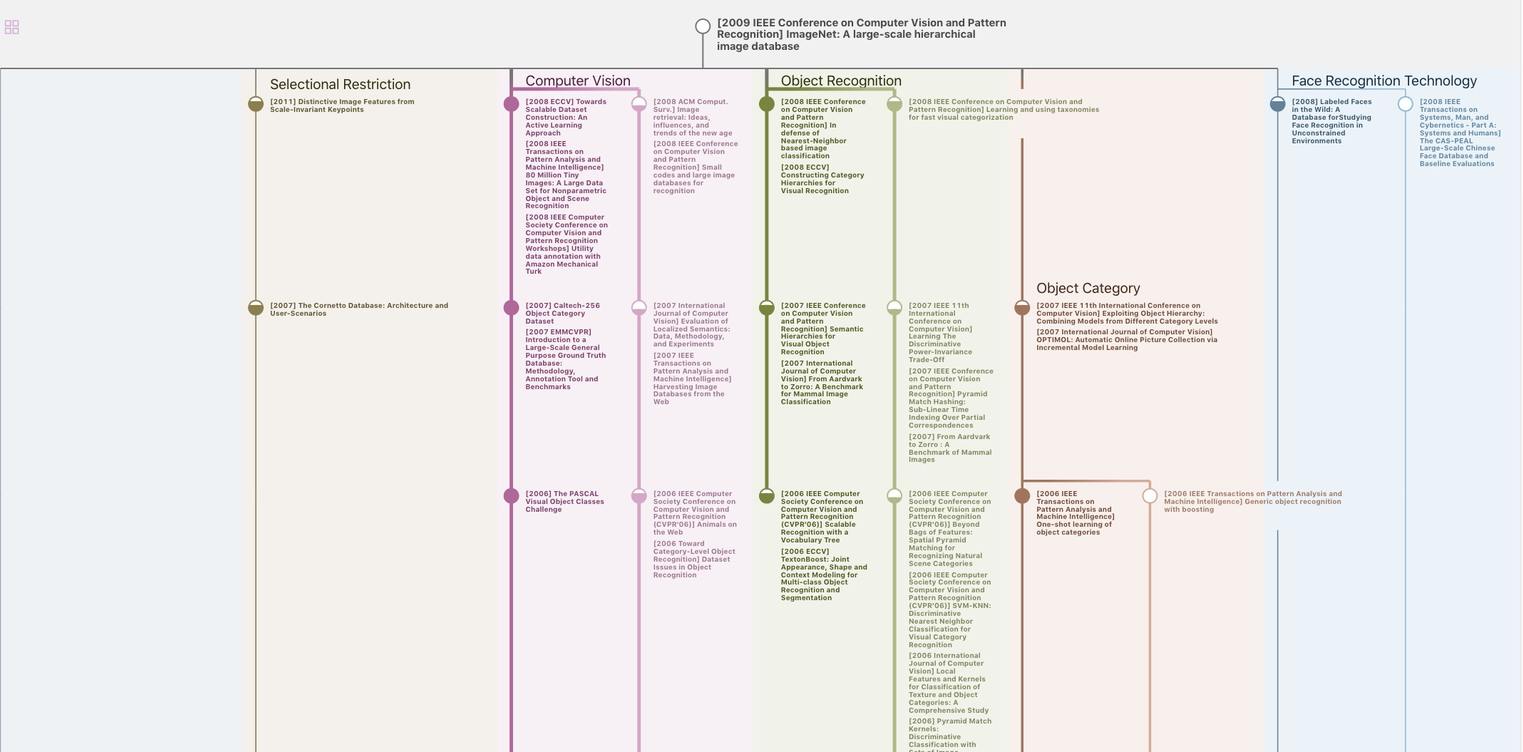
生成溯源树,研究论文发展脉络
Chat Paper
正在生成论文摘要