On the Performance of Direct Loss Minimization for Bayesian Neural Networks
arxiv(2022)
摘要
Direct Loss Minimization (DLM) has been proposed as a pseudo-Bayesian method motivated as regularized loss minimization. Compared to variational inference, it replaces the loss term in the evidence lower bound (ELBO) with the predictive log loss, which is the same loss function used in evaluation. A number of theoretical and empirical results in prior work suggest that DLM can significantly improve over ELBO optimization for some models. However, as we point out in this paper, this is not the case for Bayesian neural networks (BNNs). The paper explores the practical performance of DLM for BNN, the reasons for its failure and its relationship to optimizing the ELBO, uncovering some interesting facts about both algorithms.
更多查看译文
关键词
direct loss minimization,bayesian neural
AI 理解论文
溯源树
样例
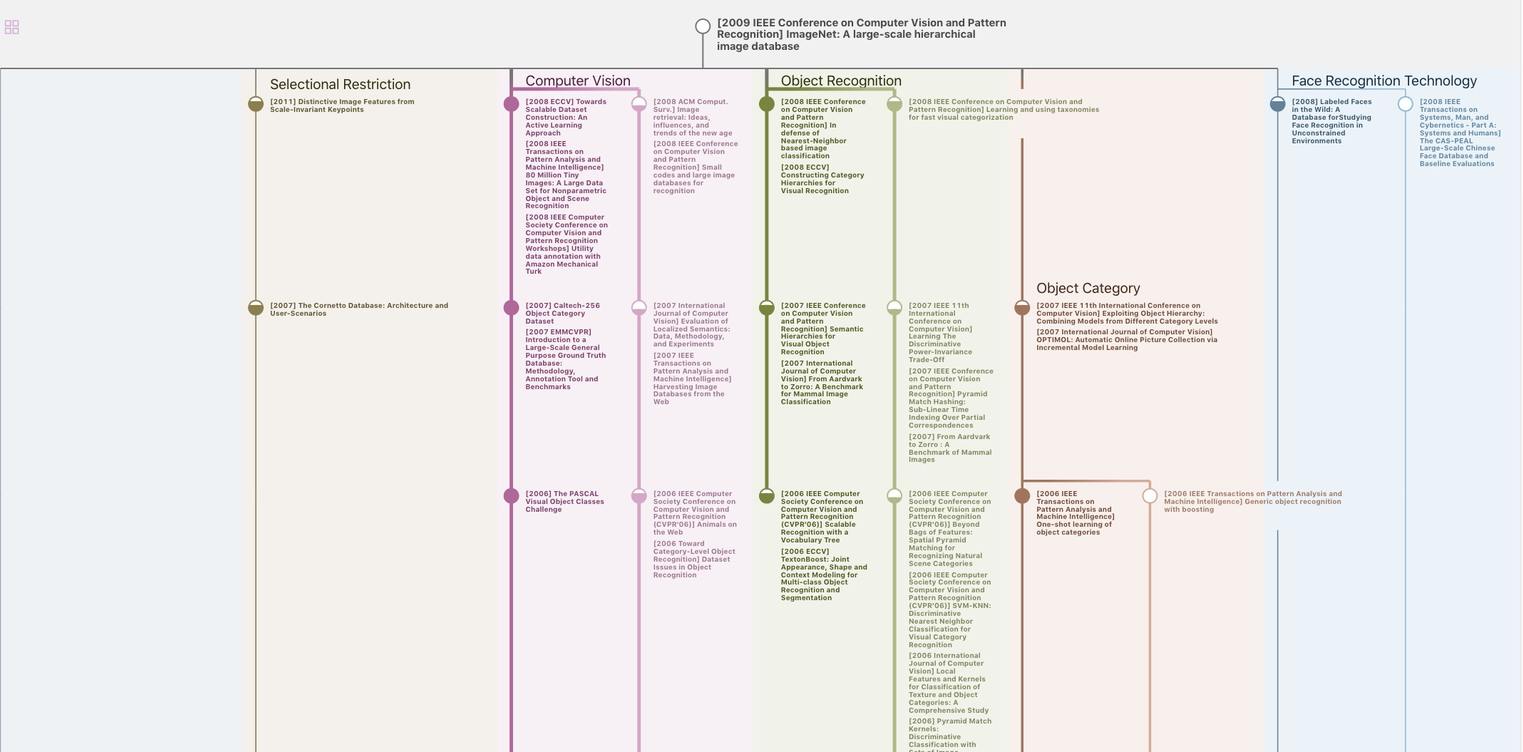
生成溯源树,研究论文发展脉络
Chat Paper
正在生成论文摘要