Adaptation Approaches for Nearest Neighbor Language Models
conf_acl(2022)
摘要
Semi-parametric Nearest Neighbor Language Models ($k$NN-LMs) have produced impressive gains over purely parametric LMs, by leveraging large-scale neighborhood retrieval over external memory datastores. However, there has been little investigation into adapting such models for new domains. This work attempts to fill that gap and suggests the following approaches for adapting $k$NN-LMs -- 1) adapting the underlying LM (using Adapters), 2) expanding neighborhood retrieval over an additional adaptation datastore, and 3) adapting the weights (scores) of retrieved neighbors using a learned Rescorer module. We study each adaptation strategy separately, as well as the combined performance improvement through ablation experiments and an extensive set of evaluations run over seven adaptation domains. Our combined adaptation approach consistently outperforms purely parametric adaptation and zero-shot ($k$NN-LM) baselines that construct datastores from the adaptation data. On average, we see perplexity improvements of 17.1\% and 16\% for these respective baselines, across domains.
更多查看译文
关键词
language,nearest,models,neighbor
AI 理解论文
溯源树
样例
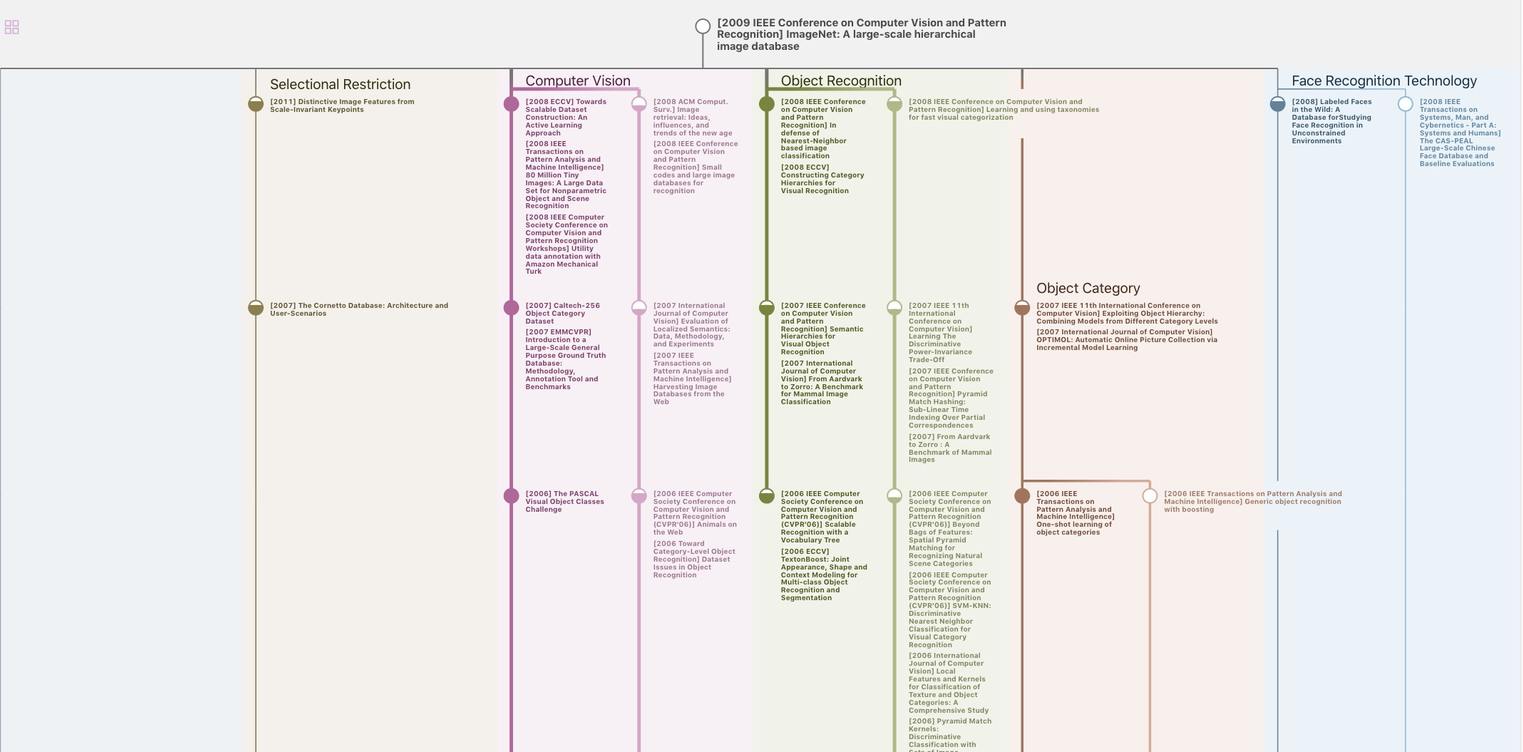
生成溯源树,研究论文发展脉络
Chat Paper
正在生成论文摘要