Ensemble Machine Learning Algorithms for Anomaly Detection in Multivariate Time-Series
2022 International Conference on Control, Automation and Diagnosis (ICCAD)(2022)
摘要
This paper proposes a multivariate time-series anomaly detection approach using multiple transform techniques and ensemble machine learning (EML) algorithms. The objective is to detect the presence of abnormal wafers during the semiconductor manufacturing process. Therefore, we evaluate a set of eleven features derived from an intermediate manufacturing chain to characterize the wafer status. Data from each feature is recorded over a 150-second time frame. To address the computational complexity of large-scale data processing, a dimensionality reduction step is highly desirable. Indeed, independent component analysis (ICA), principal component analysis (PCA), and factor analysis (FA) are used for comparison purposes. As well, to extract the most significant components from each feature sequence and build a thoroughly combined subset of characteristics. In the sequel, decision trees, bootstrap aggregating, boosting, one of the prevalent evolutions of EML algorithms, are fitted to the obtained characteristics to define the best anomaly detection ranking. The selected model is validated using 7000 samples (i.e. wafers) divided into 5000 normal samples and 2000 abnormal samples. The results highlight the strengths of the proposed approach, which could serve as a valuable decision-making support for abnormal wafer detection in the semiconductor manufacturing process.
更多查看译文
关键词
Anomaly detection,Data-driven methods,Ensemble machine learning,Multivariate time series analysis,Semiconductor manufacturing
AI 理解论文
溯源树
样例
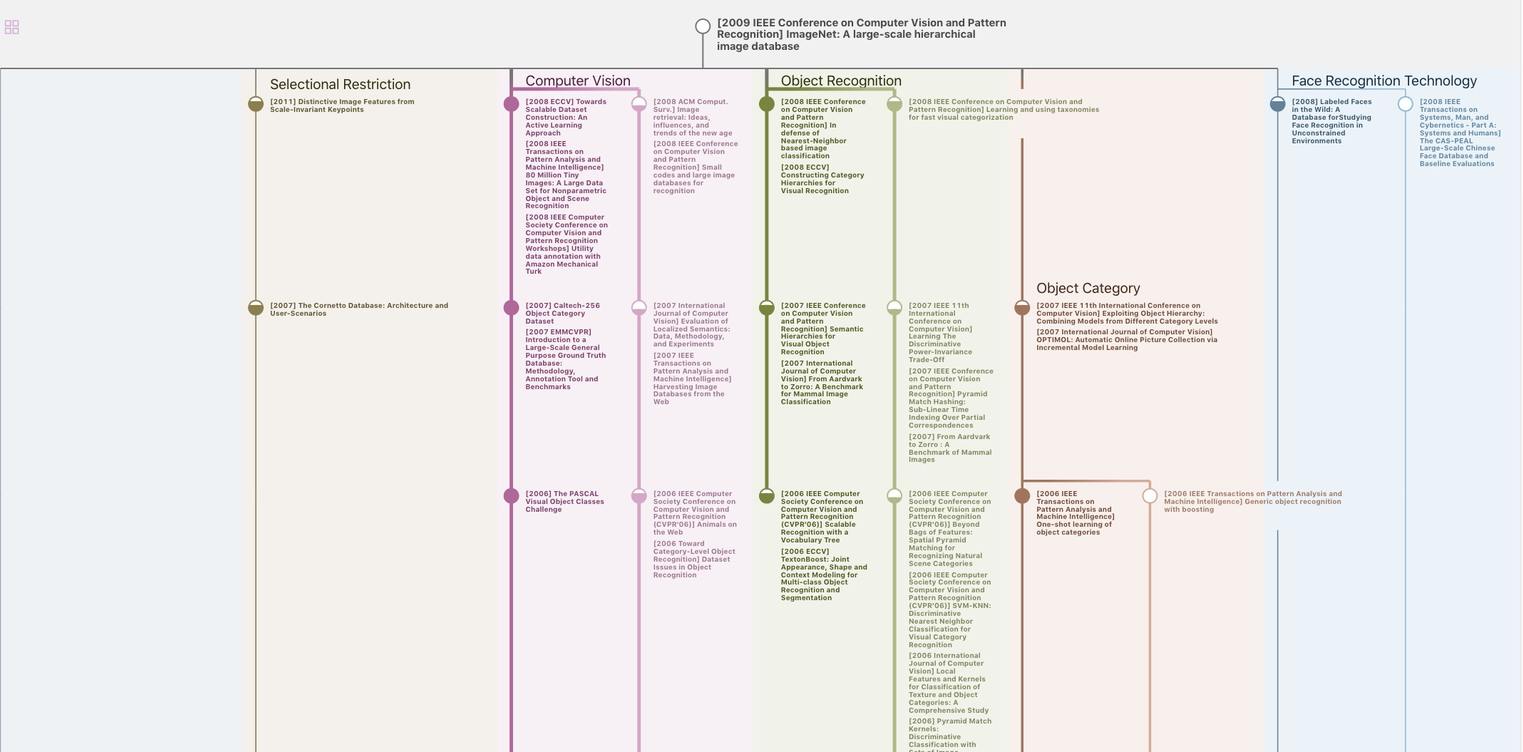
生成溯源树,研究论文发展脉络
Chat Paper
正在生成论文摘要