Shared-Private Memory Networks For Multimodal Sentiment Analysis
IEEE TRANSACTIONS ON AFFECTIVE COMPUTING(2023)
摘要
Text, visual, and acoustic are usually complementary in the Multimodal Sentiment Analysis (MSA) task. However, current methods primarily concern shared representations while neglecting the critical private aspects of data within individual modalities. In this work, we propose shared-private memory networks based on the recent advances in the attention mechanism, called SPMN, to decouple multimodal representation from shared and private perspectives. It contains three components: a) a shared memory to learn the shared representations of multimodal data; b) three private memories to learn the private representations of individual modalities, respectively; c) and adaptive fusion gates to fuse multimodal private and shared representations. To evaluate the effectiveness of SPMN, we integrate it into different pre-trained language representation models, such as BERT and XLNET, and conduct experiments on two public datasets, CMU-MOSI and CMU-MOSEI. Experimental results indicate that the performances of pre-trained language representation models are significantly improved because of SPMN and demonstrate the superiority of our model compared to the state-of-the-art methods. SPMN's source code is publicly available at: https://github.com/xiaobaicaihhh/SPMN.
更多查看译文
关键词
Multimodal sentiment analysis,shared-private memory netwoks,adaptive fusion gate,BERT
AI 理解论文
溯源树
样例
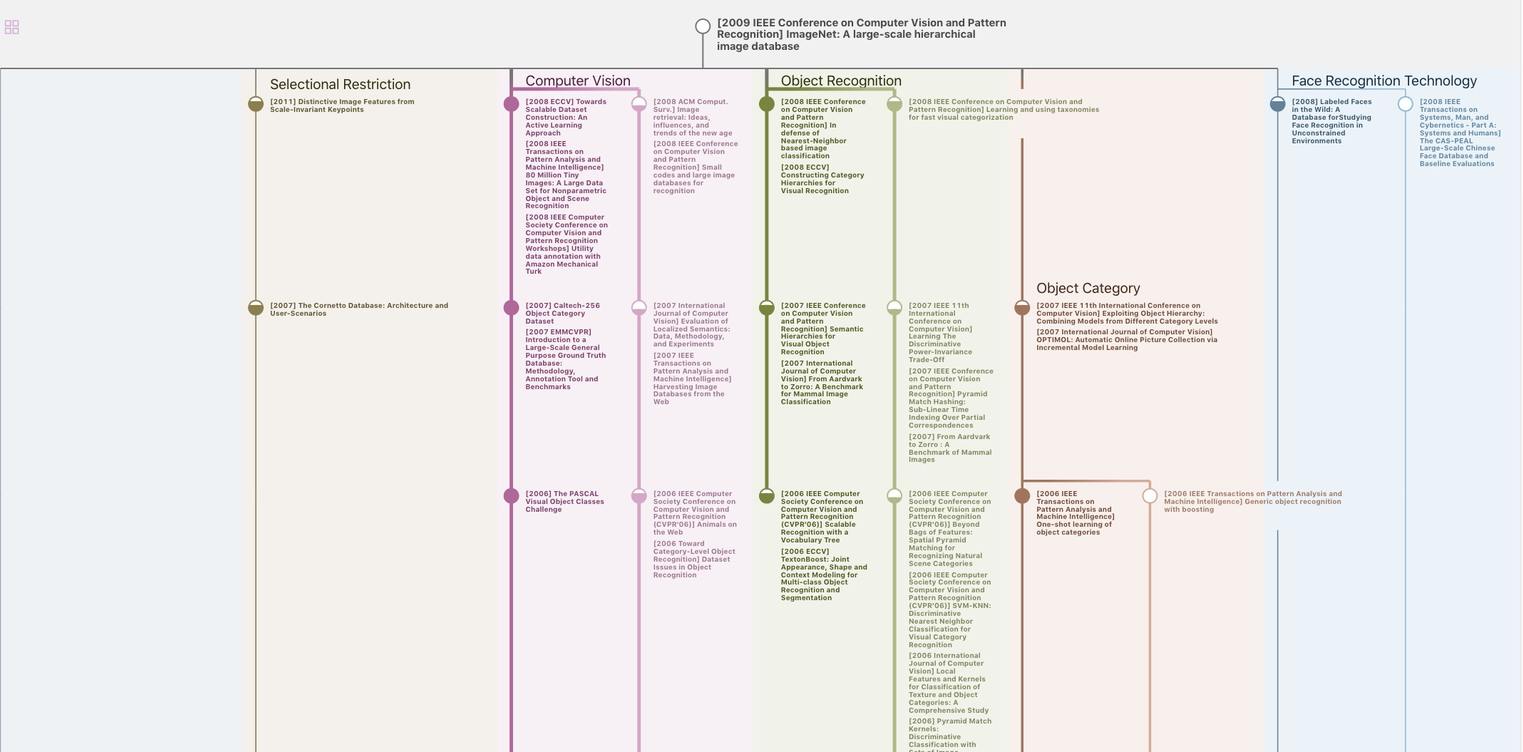
生成溯源树,研究论文发展脉络
Chat Paper
正在生成论文摘要