Prediction of electronic work function of the second phase in binary magnesium alloy based on machine learning method
Journal of Materials Research(2022)
摘要
In this paper, 150 pieces of the work function of Mg-based solid solutions and Mg-containing second phases calculated by our group based on density functional theory (DFT) were collected to build a data set. According to the analysis of Pearson and Spearman correlation, 14 features were used as input variables. Four machine learning (ML) models including multiple linear regression, support vector regression (SVR), gradient boosting regression tree, extreme gradient boosting tree were established to predict the work function of second phases. The result shows that the SVR model has the highest accuracy and best generalization ability to predict the work function. The work function of Mg 7 Zn 3 phase predicted from the SVR model is very close to that calculated from DFT, which suggests that ML is an effective method to predict the work function in Mg alloy. Graphical abstract
更多查看译文
关键词
Mg, Alloy, Second phases, Corrosion, Machine learning
AI 理解论文
溯源树
样例
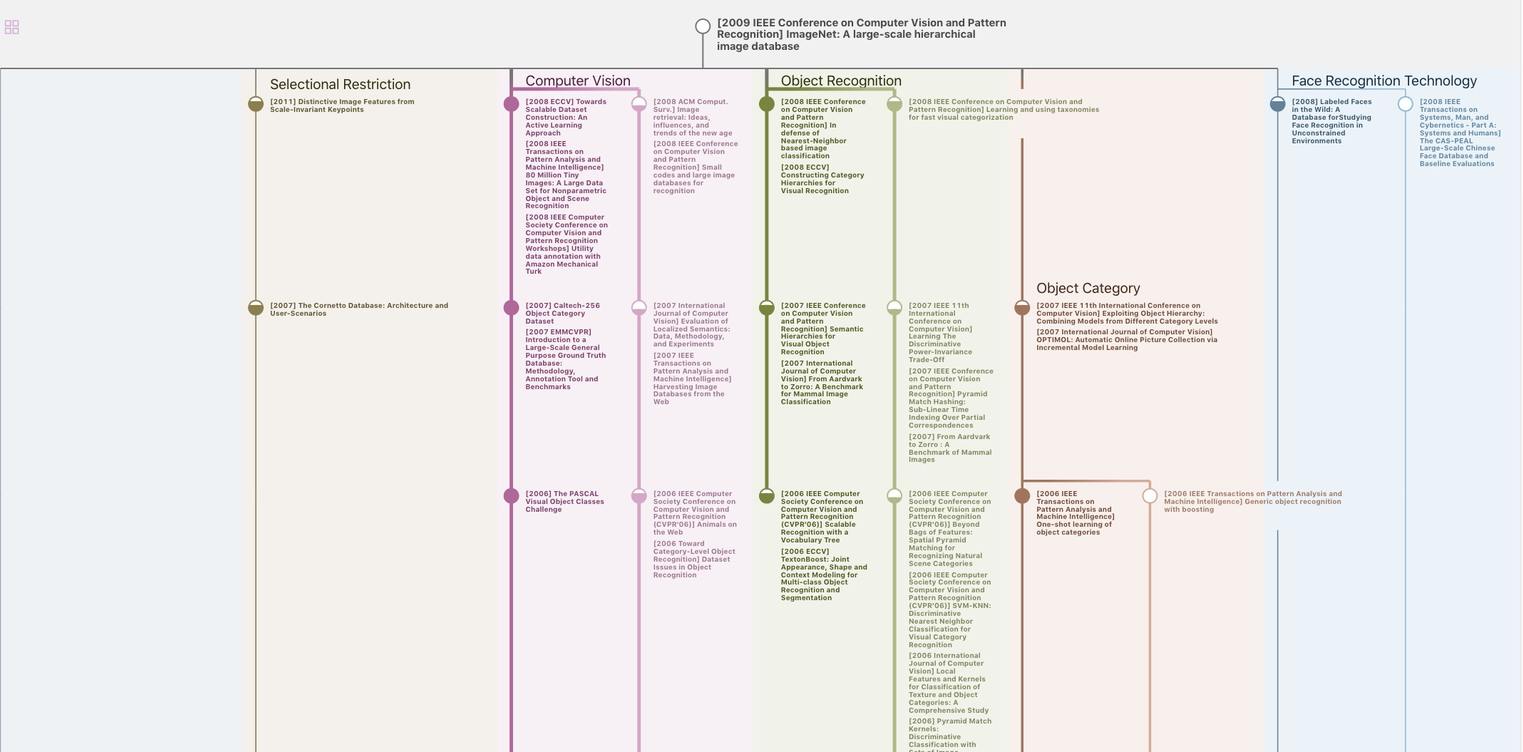
生成溯源树,研究论文发展脉络
Chat Paper
正在生成论文摘要