Pixel-Level Self-Supervised Learning for Semi-Supervised Building Extraction From Remote Sensing Images
IEEE GEOSCIENCE AND REMOTE SENSING LETTERS(2022)
摘要
Building extraction from remotely sensed images has been challenging yet vital for applicable purposes such as urban monitoring and cartography. Most existing learning-based approaches focus on supervised methods, in which the models should be trained with images and the corresponding labels. This research exploits a semi-supervised approach for building extraction. Specifically, the backbone is first trained in pixel-level self-supervised learning (SSL) manner without labels rather than commonly used supervised or instance-level SSL methods. Next, the pretrained backbone is combined with a prediction head using multiscale features, and the whole network is tuned with limited annotations. Experimental results conducted on three popular datasets show that our method achieves improvements regarding both intersection over union (IoU) and F1-score compared to both supervised, and the methods with instance-level SSL pretrained backbone. Our study confirms the potential of the proposed approach for building extraction from remote sensing images.
更多查看译文
关键词
Building extraction, remote sensing, semantic segmentation, semi-supervised learning (SSL), transfer learning
AI 理解论文
溯源树
样例
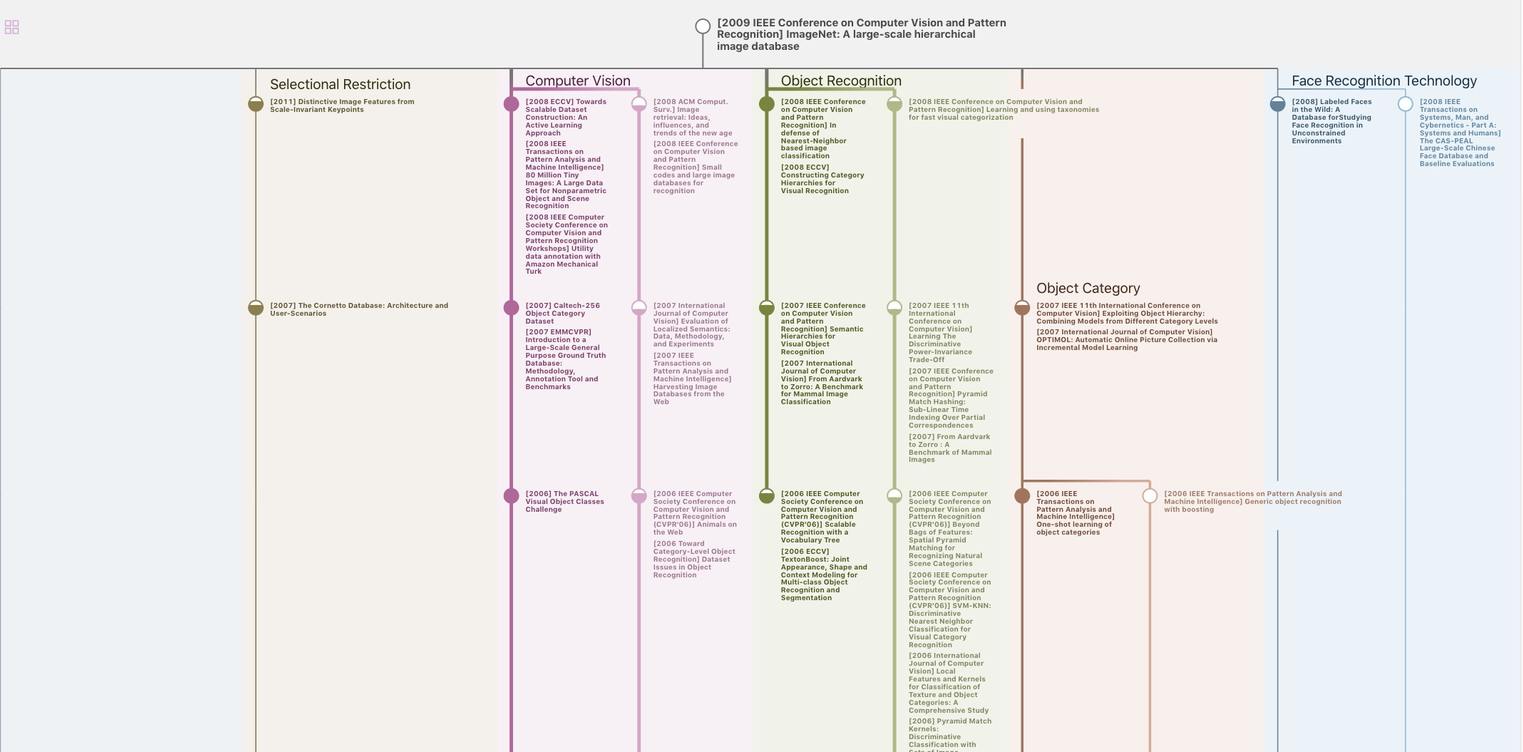
生成溯源树,研究论文发展脉络
Chat Paper
正在生成论文摘要