Dense Feature Learning and Compact Cost Aggregation for Deep Stereo Matching
IEEE ACCESS(2022)
摘要
Recently, Convolutional Neural Networks (CNN) based deep models have been successfully applied to the task of stereo matching. In this paper, we propose a novel deep stereo matching network based on the strategies of dense feature learning and compact cost aggregation, namely DFL-CCA-Net. It consists of three modules: Dense Feature Learning (DFL), Compact Cost Aggregation (CCA) and the disparity regression module. In DFL module, the CNN backbone with Dense Atrous Spatial Pyramid Pooling (DenseASPP) is employed to extract multi-scale deep feature maps of the given left and right images respectively. Then an initial 4D cost volume is obtained by concatenating left feature maps with their corresponding right feature maps across each disparity level. In the following CCA module, each initial 3D cost volume component (i.e., the component across the left or right image feature channel dimension) is aggregated into a more compact one by using the atrous convolution operation with different expansion rates. These updated 3D cost volume components are then fed into the disparity regression module, which consisting of a 3D CNN network with a stacked hourglass structure, to estimate the final disparity map. Comprehensive experimental results demonstrated on the Scene Flow, KITTI 2012 and KITTI 2015 datasets show that the 3D cost volume components obtained by the proposed DFL and CCA modules generally containing more multi-scale semantic information and thus can largely improve the final disparity regression accuracies. Compared with other deep stereo matching methods, DFL-CCA-Net achieves very competitive prediction accuracies especially in the reflective regions and regions containing detail information.
更多查看译文
关键词
Costs, Three-dimensional displays, Feature extraction, Representation learning, Convolution, Data mining, Correlation, Stereo vision, Deep stereo matching, dense feature learning, compact cost aggregation
AI 理解论文
溯源树
样例
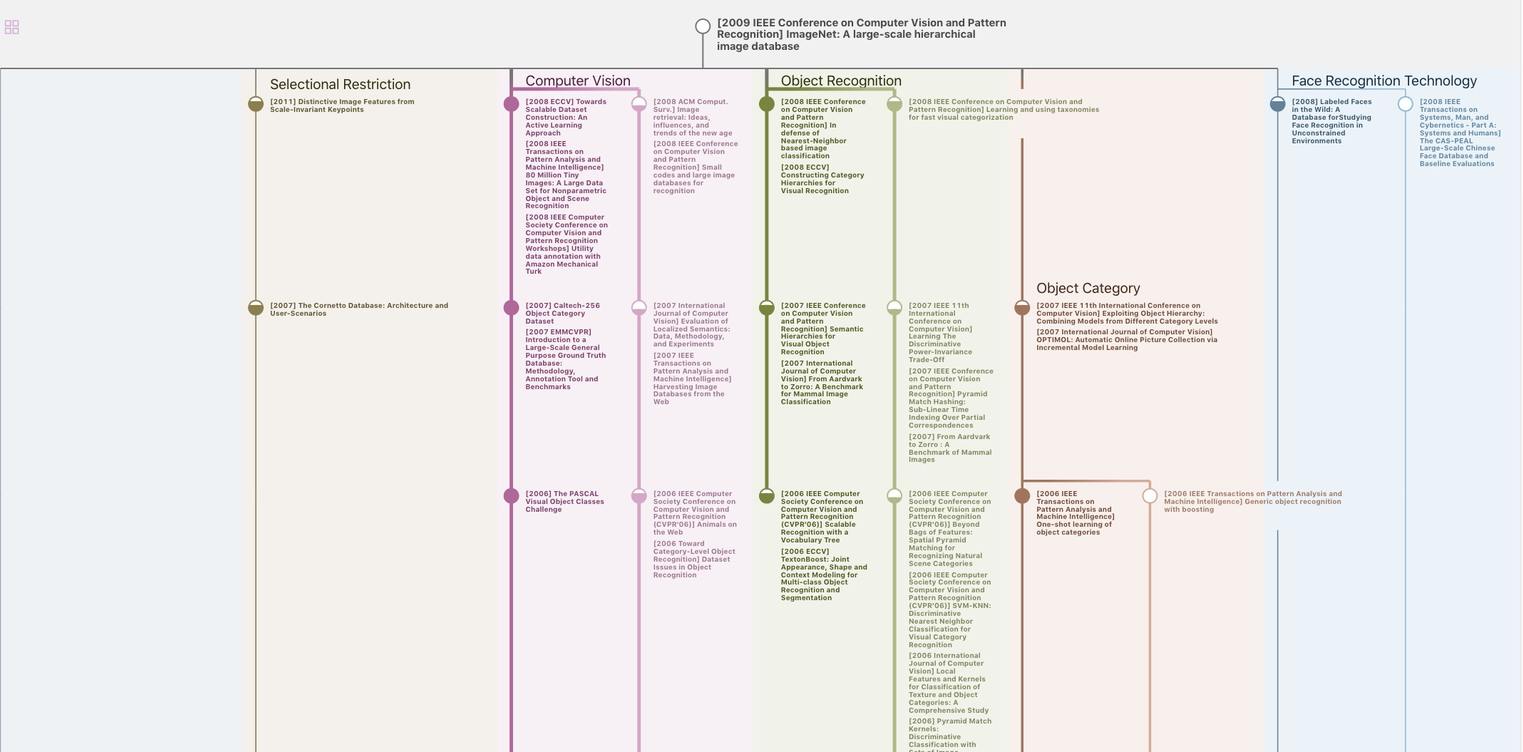
生成溯源树,研究论文发展脉络
Chat Paper
正在生成论文摘要