Detecting Wheat Heads from UAV Low-Altitude Remote Sensing Images Using Deep Learning Based on Transformer
REMOTE SENSING(2022)
摘要
The object detection method based on deep learning convolutional neural network (CNN) significantly improves the detection performance of wheat head on wheat images obtained from the near ground. Nevertheless, for wheat head images of different stages, high density, and overlaps captured by the aerial-scale unmanned aerial vehicle (UAV), the existing deep learning-based object detection methods often have poor detection effects. Since the receptive field of CNN is usually small, it is not conducive to capture global features. The visual Transformer can capture the global information of an image; hence we introduce Transformer to improve the detection effect and reduce the computation of the network. Three object detection networks based on Transformer are designed and developed, including the two-stage method FR-Transformer and the one-stage methods R-Transformer and Y-Transformer. Compared with various other prevalent object detection CNN methods, our FR-Transformer method outperforms them by 88.3% for AP(50) and 38.5% for AP(75). The experiments represent that the FR-Transformer method can gratify requirements of rapid and precise detection of wheat heads by the UAV in the field to a certain extent. These more relevant and direct information provide a reliable reference for further estimation of wheat yield.
更多查看译文
关键词
object detection, deep learning, remote sensing monitoring, phenotyping analysis, wheat head counting, UAV, transformer
AI 理解论文
溯源树
样例
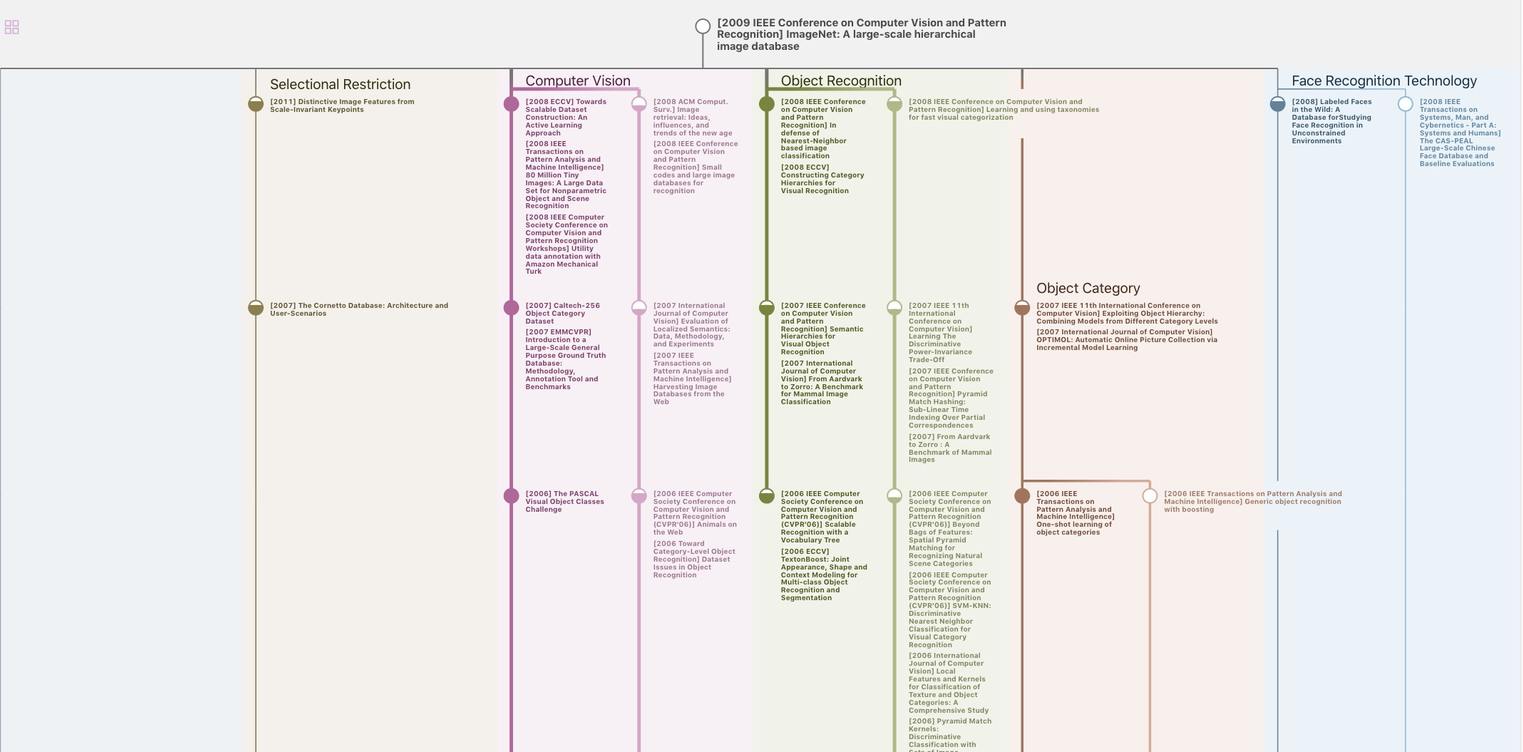
生成溯源树,研究论文发展脉络
Chat Paper
正在生成论文摘要