Impact of data quality on supervised machine learning: Case study on drilling vibrations
Journal of Petroleum Science and Engineering(2022)
Abstract
Training complex machine learning and deep learning models has become straightforward with the advent of highly efficient, open-source machine learning libraries. Supervised classification techniques such as logistic regression, random forests, and neural networks have also gained popularity in the drilling industry on the back of promising results. As a result, these techniques have been increasingly researched, especially in the domain of drilling vibrations. However, much of this research interest has been limited to finding the best classification model for estimating severity of downhole vibrations. While the choice of classification model is important, we argue that the successful implementation and adoption of machine learning technologies is equally dependent on correctly studying, cleaning, pre-processing the vibration drilling data before applying machine learning techniques. We show that, in certain cases, correctly pre-processing the data guarantees competitive classification performance regardless of the choice of classification model. Specifically, we empirically investigate how factors such as data sampling frequency, data labeling technique, feature extraction technique, and class imbalance impact the performance of different popular classifiers, when dealing with drilling data. We make recommendations specific to vibration classification and highlight pitfalls of certain techniques in that context. Finally, we also develop a step-by-step workflow which enables users to select the correct parameters and techniques at every step, from data collection to model training.
MoreTranslated text
Key words
Data quality,Supervised learning,Classification,Drilling vibrations,Sampling frequency,Data imbalance,Feature extraction,Data labeling
AI Read Science
Must-Reading Tree
Example
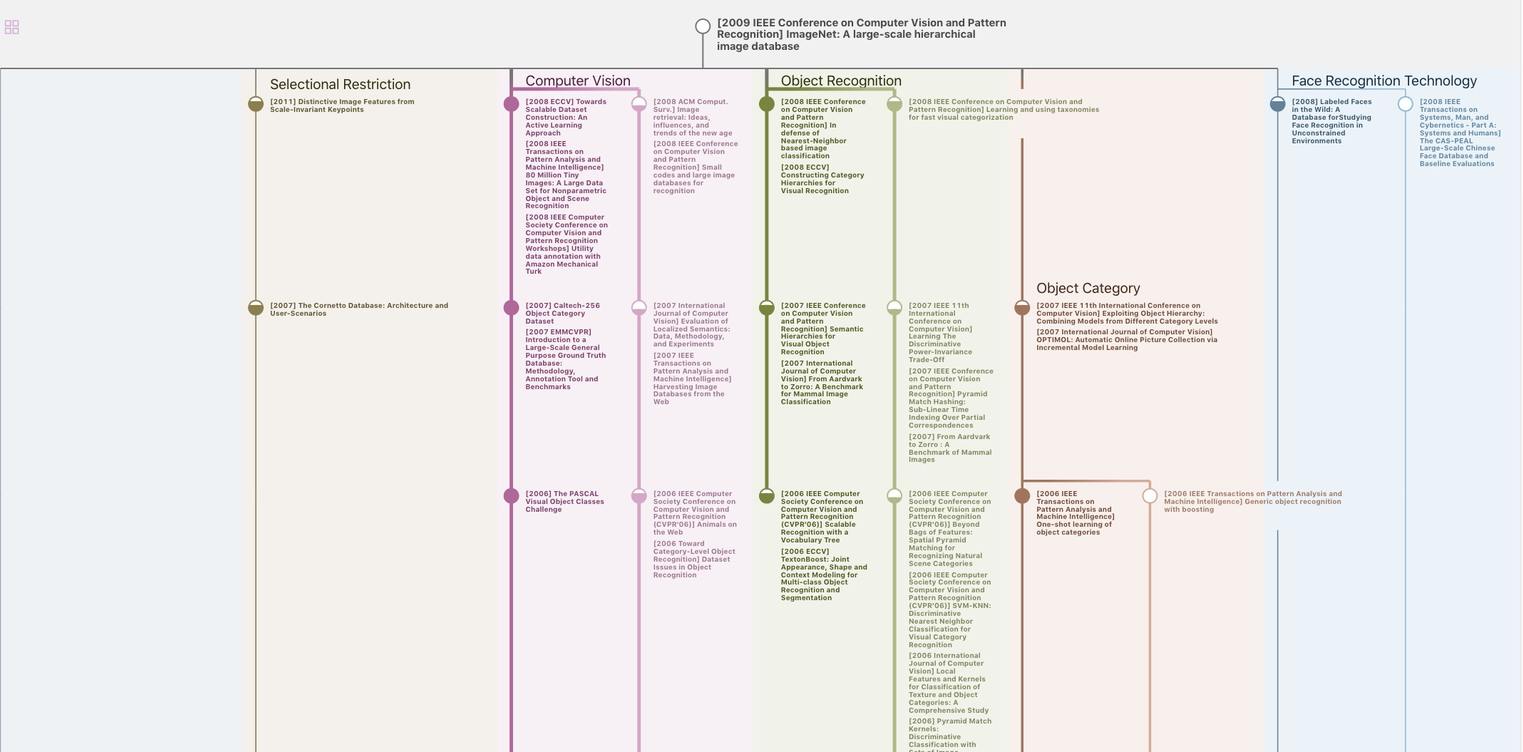
Generate MRT to find the research sequence of this paper
Chat Paper
Summary is being generated by the instructions you defined