Comparing observed and unobserved fishing characteristics in the drift gillnet fishery for swordfish
Fisheries Research(2022)
摘要
Central to the success of partial coverage observer programs is the assumption that observed fishing behaviors are representative of unobserved fishing behaviors. Traditionally, this was a difficult assumption to test beyond comparing catch compositions for observed and unobserved trips. With the proliferation of vessel monitoring systems (VMS) however, a suite of trip- and set-level fishing characteristics can be engineered from vessel locations that facilitate quantitative comparisons between observed and unobserved behaviors. The U.S. West Coast drift gillnet (DGN) fishery for swordfish (Xiphias gladius) is a small fishery with a long history of bycatch concerns and an observer coverage rate around 20–30 %. We developed an integrated data set composed of VMS, logbook, landings, and observer data for the DGN fishery from 2013 to 2019. We used a suite of machine learning models to classify unobserved fishing behaviors and we characterized these fishing behaviors at the trip- and set-level. Analyses of the trip- and set-level metrics (e.g., trip duration, fishing depth, sea surface temperatures, distance from port, and catch per unit effort) revealed that operational characteristics of the fishery were largely similar for observer and unobserved trips and vessels, but also highly variable. In the fall season, observed trip distances were an average of 53 % (std. error 20 %) longer than unobserved trips for the same vessels. These longer trips occurred in water that was, on average, about 39 % (std. error 16 %) deeper. However, characteristics like amounts of fishing effort, revenue, and water temperatures were generally similar between observed and unobserved trips. We present a methodical workflow for data integration and feature engineering and a simple approach for assessing differences across fleet behaviors in space and time that could be applied to many fisheries with limited observer coverage. This approach could also be used to target specific strata within fisheries that may benefit from increases or shifts in observer coverage.
更多查看译文
关键词
Observer effects,Machine learning,Fleet dynamics,Drift gillnet,Swordfish
AI 理解论文
溯源树
样例
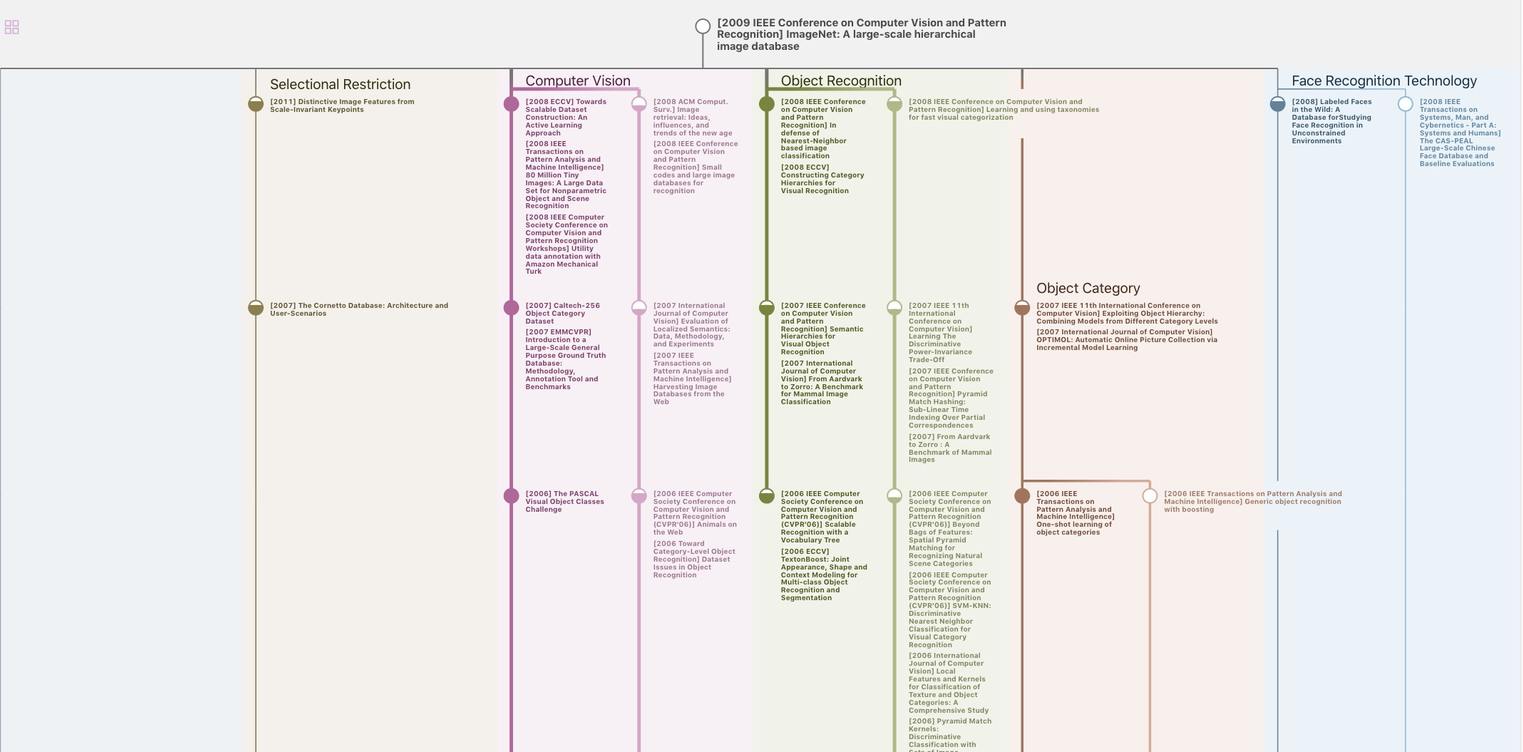
生成溯源树,研究论文发展脉络
Chat Paper
正在生成论文摘要