Weighted Naive Bayes Approach for Imbalanced Indoor Positioning System Using UWB
2022 IEEE International Black Sea Conference on Communications and Networking (BlackSeaCom)(2022)
摘要
The accuracy and reliability of an Ultra- WideBand (UWB) Indoor Positioning System (IPS) are compromised owing to the positioning error caused by the Non-Line-of-Sight (NLoS) signals. To address this, Machine Learning (ML) has been employed to classify Line-of-Sight (LoS) and NLoS components. However, the performance of ML algorithms degrades due to the disproportion of the number of LoS and NLoS signal components. A Weighted Naive Bayes (WNB) algorithm is proposed in this paper to mitigate this issue. The performance of the proposed algorithm is compared with conventional state-of-the-art ML algorithms such as K-Nearest Neighbor (KNN), Support Vector Machine (SVM), and Decision Tree (DT) using the Receiver Operating Characteristic (ROC) and Area Under the Curve (AUC). The results prove that the WNB classifier can significantly reduce the impact of the limited number of NLoS components that are available for training the model. The proposed WNB algorithm also maintains a high classification accuracy and robustness in mixed LoS/NLoS conditions.
更多查看译文
关键词
Ultra-wideBand,Indoor Positioning System,Machine Learning,Non-Line-of-Sight Identification,Naive Bayes,Weighted Naive Bayes
AI 理解论文
溯源树
样例
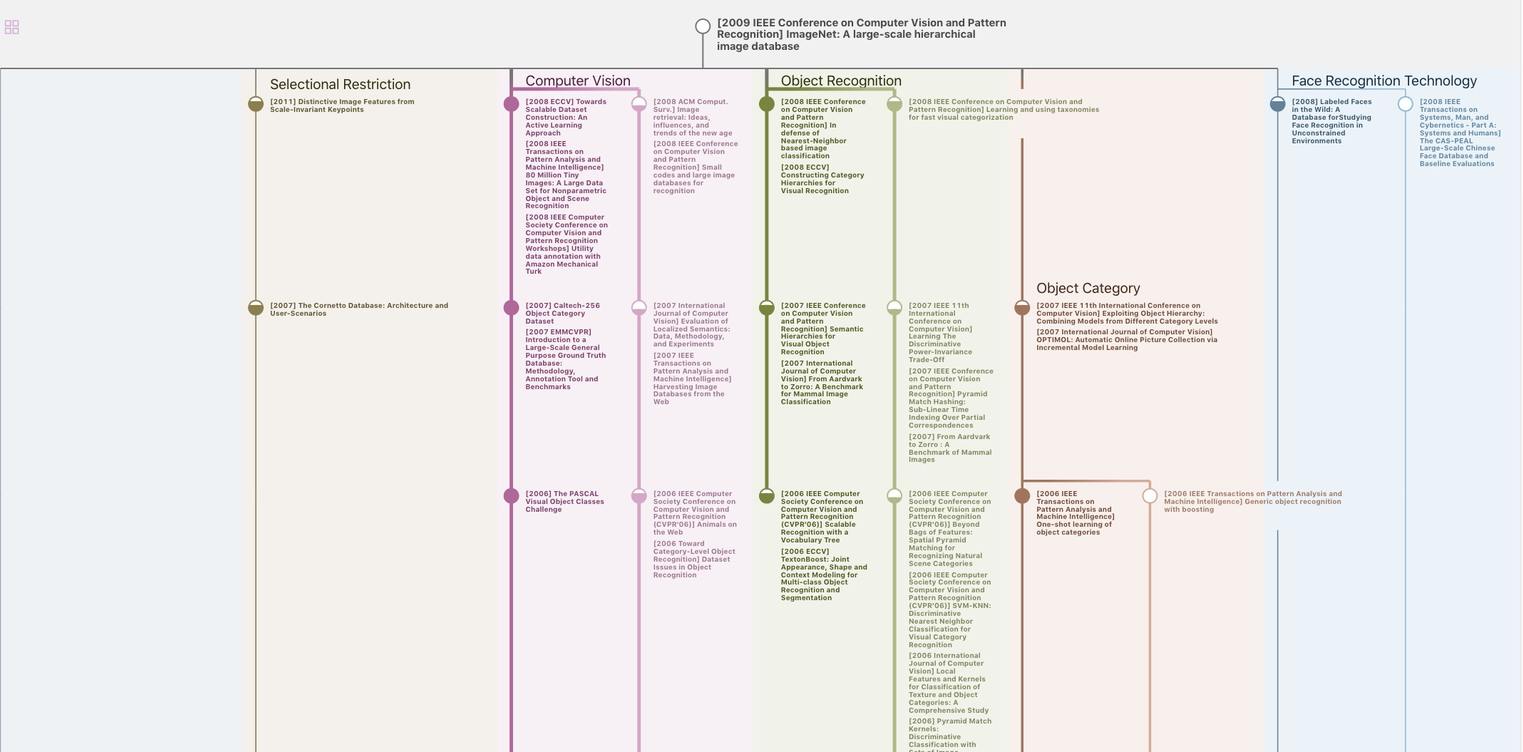
生成溯源树,研究论文发展脉络
Chat Paper
正在生成论文摘要