Unsupervised Word Segmentation with BERT Oriented Probing and Transformation
FINDINGS OF THE ASSOCIATION FOR COMPUTATIONAL LINGUISTICS (ACL 2022)(2022)
摘要
Word Segmentation is a fundamental step for understanding many languages. Previous neural approaches for unsupervised Chinese Word Segmentation (CWS) only exploit shallow semantic information, which can miss important context. Large scale Pre-trained language models (PLM) have achieved great success in many areas. In this paper, we propose to take advantage of the deep semantic information embedded in PLM (e.g., BERT) with a self-training manner, which iteratively probes and transforms the semantic information in PLM into explicit word segmentation ability. Extensive experiment results show that our proposed approach achieves a state-of-the-art F1 score on two CWS benchmark datasets. The proposed method can also help understand low resource languages and protect language diversity.(1)
更多查看译文
AI 理解论文
溯源树
样例
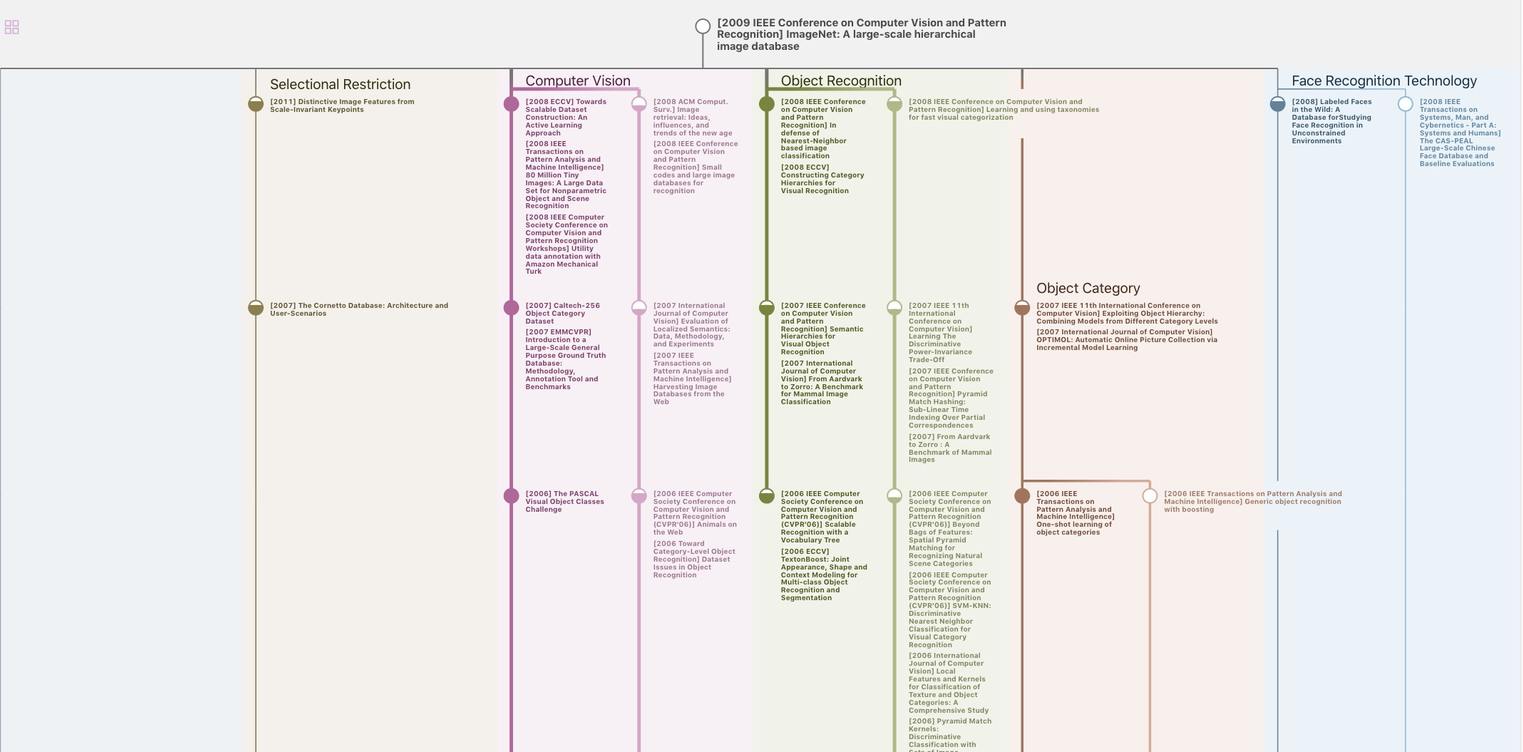
生成溯源树,研究论文发展脉络
Chat Paper
正在生成论文摘要