Extractive text summarization using clustering-based topic modeling
Soft Computing(2022)
摘要
Text summarization is the process of converting the input document into a short form, provided that it preserves the overall meaning associated with it. Primarily, text summarization is achieved in two ways, i.e., abstractive and extractive. Extractive summarizers select a few best sentences out of the input document, while abstractive methods may modify the sentence structure or introduce new sentences. The proposed approach is an extractive text summarization technique, where we have expanded topic modeling specifically to be applied to multiple lower-level specialized entities (i.e., groups) embedded in a single document. Our goal is to overcome the lack of coherence issues found in the summarization techniques. Topic modeling was initially proposed to model text data at the multi-document and word levels without considering sentence modeling. Subsequently, it has been applied at the sentence level and used for the document summarization; however, certain limitations were associated. Topic modeling does not perform as expected when applied to a single document at the sentence level. To address this shortcoming, we have proposed a summarization approach that is incorporated at the individual document and clusters level (instead of the sentence level). We aim to choose the best statement from each group (containing sentences of the same kind) found in the given text. We have tried to select the perfect topic by evaluating the probability distribution of the words and respective topics’ at the cluster level. The method is evaluated on two standard datasets and shows significant performance gains over existing text summarization techniques. Compared to other text summarization techniques, the Rouge parameters for automatic evaluation show a considerable improvement in F-measure, precision, and recall of the generated summary. Furthermore, a manual evaluation has demonstrated that the proposed approach outperforms the current state-of-the-art text summarization approaches.
更多查看译文
关键词
Extractive summarization, Topic modeling, Clustering, Semantic measure
AI 理解论文
溯源树
样例
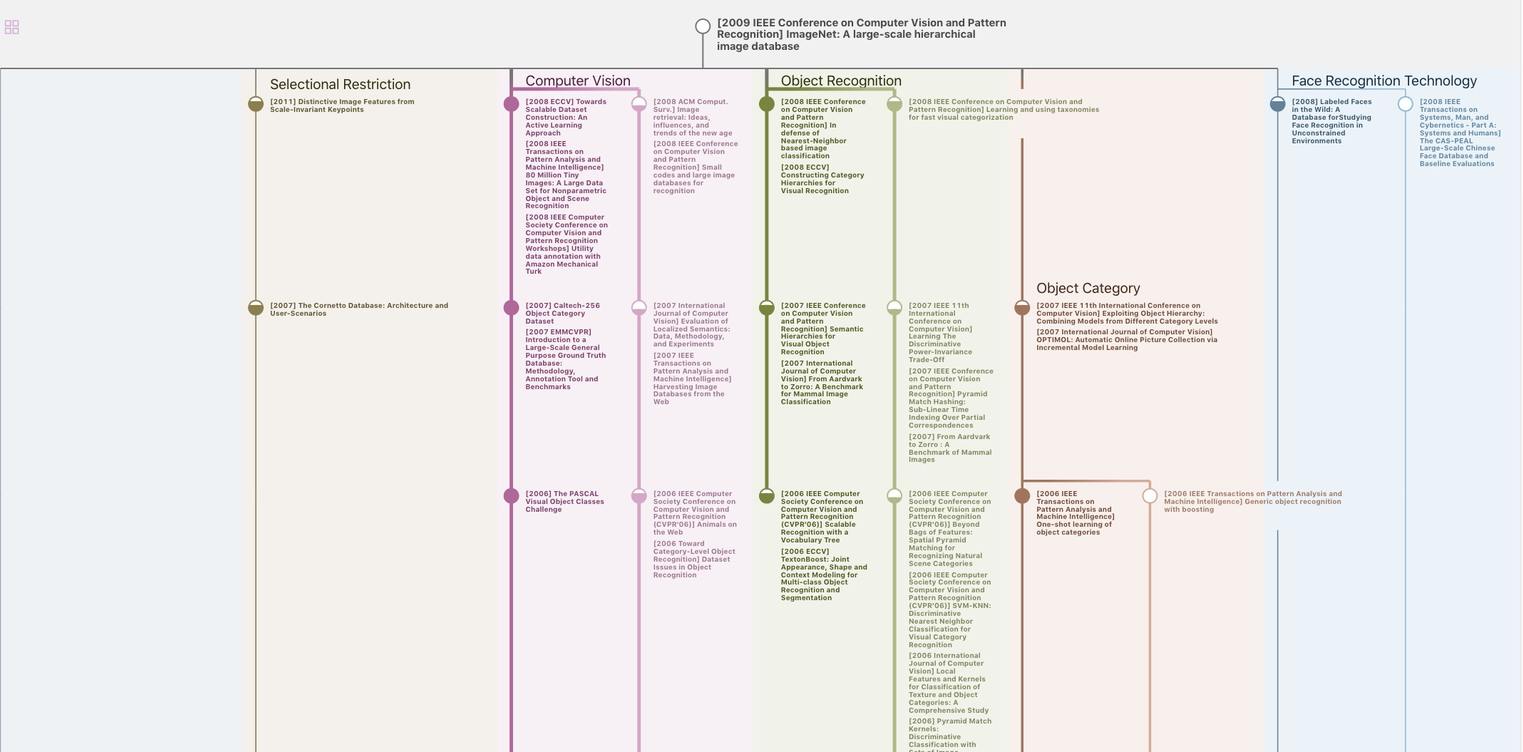
生成溯源树,研究论文发展脉络
Chat Paper
正在生成论文摘要