Heterogeneous Graph Neural Network for Multi-behavior Feature-Interaction Recommendation
ARTIFICIAL NEURAL NETWORKS AND MACHINE LEARNING - ICANN 2022, PT IV(2022)
Abstract
Graph neural network has great advantage in learning vector representation of users and items for modern recommender systems. Modeling user-item interaction bipartite graph is helpful for learning the collaborative signals between users and items. However, this modeling scheme ignores the influence of the objectively existing attribute information of item itself, and cannot well explain why users focus on items. A feature interaction-based graph convolutional collaborative filtering algorithm, Attention Interaction Graph Collaborative Filtering (ATGCF) is proposed to address the limitations of existing works in this paper. It can deeply explore user preferences for multi-feature items. Firstly, we inject user and multi-feature into a user-feature interaction layer composed of multi-head-attention and fully connected layers to capture the user potential preference for multi-feature. Then we build a user-features-item bipartite graph and design the corresponding graph aggregation layer to obtain the high-order connectivity between the three. Experiment results on three real-world datasets verify the effectiveness of our model in exploiting multi-feature data, and ATGCF algorithm outperforms the other baselines in terms of recall, precision and NDCG evaluationmetrics. Moreover, further experiments demonstrate that our model can achieve better results with less data.
MoreTranslated text
Key words
Multi-head attention, Feature interaction, Collaborative filtering, Heterogeneous graph networks, Recommendation system
AI Read Science
Must-Reading Tree
Example
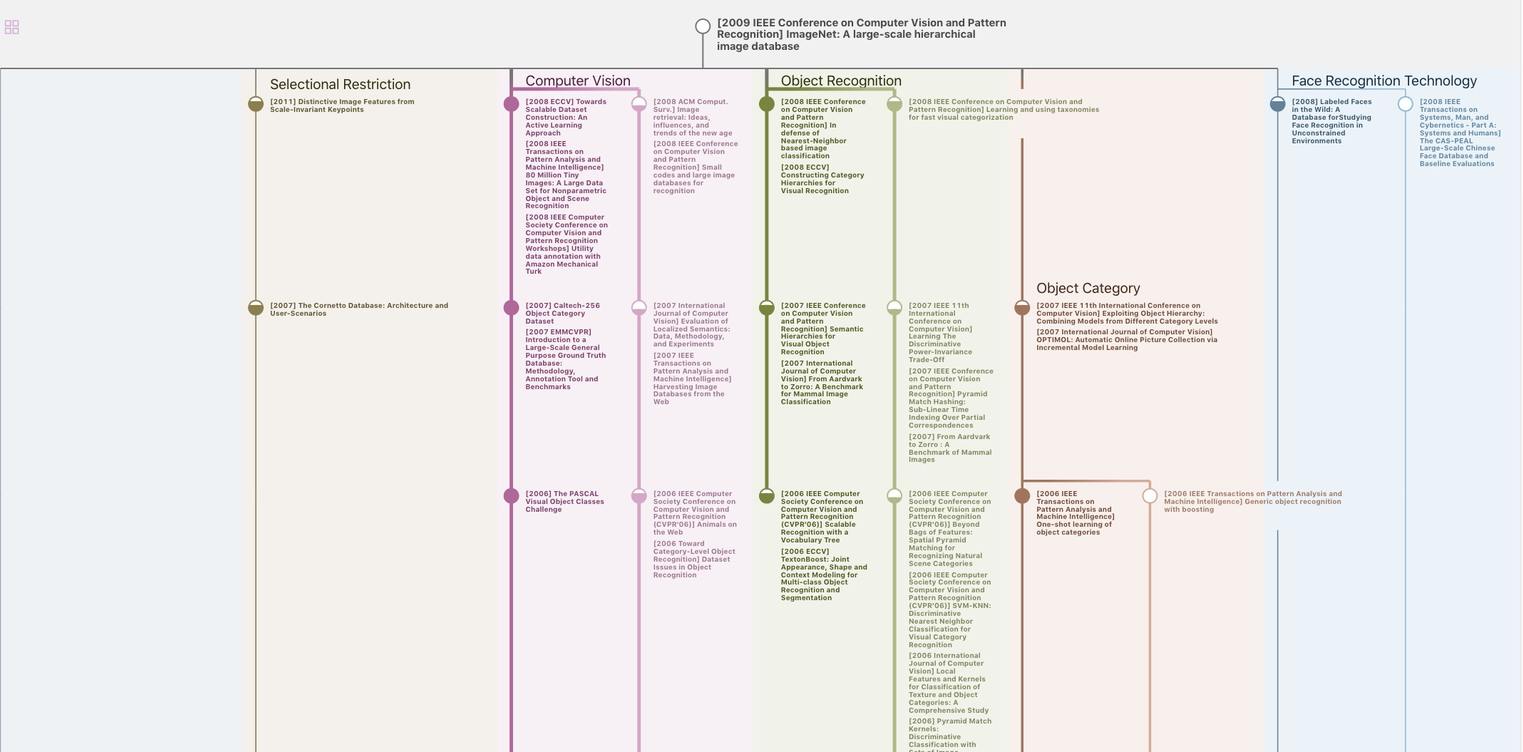
Generate MRT to find the research sequence of this paper
Chat Paper
Summary is being generated by the instructions you defined