Hypergraph Variational Autoencoder for Multimodal Semi-supervised Representation Learning
ARTIFICIAL NEURAL NETWORKS AND MACHINE LEARNING - ICANN 2022, PT IV(2022)
Abstract
In many real-world settings, the external environment is perceived through multi-modal information, such as visual, radar, lidar, etc. Naturally, the fact motivates us to exploit interaction intra modals and integrate multiple source information using limited labels on the multimodal dataset as a semi-supervised task. A challenging issue in multimodal semi-supervised learning is the complicated correlations under pairwise modalities. In this paper, we propose a hypergraph variational autoencoder (HVAE) which can mine high-order interaction of multimodal data and introduce extra prior knowledge for inferring multimodal fusion representation. On one hand, the hypergraph structure can represent high-order data correlation in multimodal scenes. On the other hand, a prior distribution is introduced by mask-based variational inference to enhance multi-modal characterization. Moreover, the variational lower bound is leveraged to collaborate semi-supervised learning. We conduct experiments on semi-supervised visual object recognition task, and extensive experiments on two datasets demonstrate the superiority of our method against the existing baselines.
MoreTranslated text
Key words
Hypergraph,Multimodal,Semi-supervised
AI Read Science
Must-Reading Tree
Example
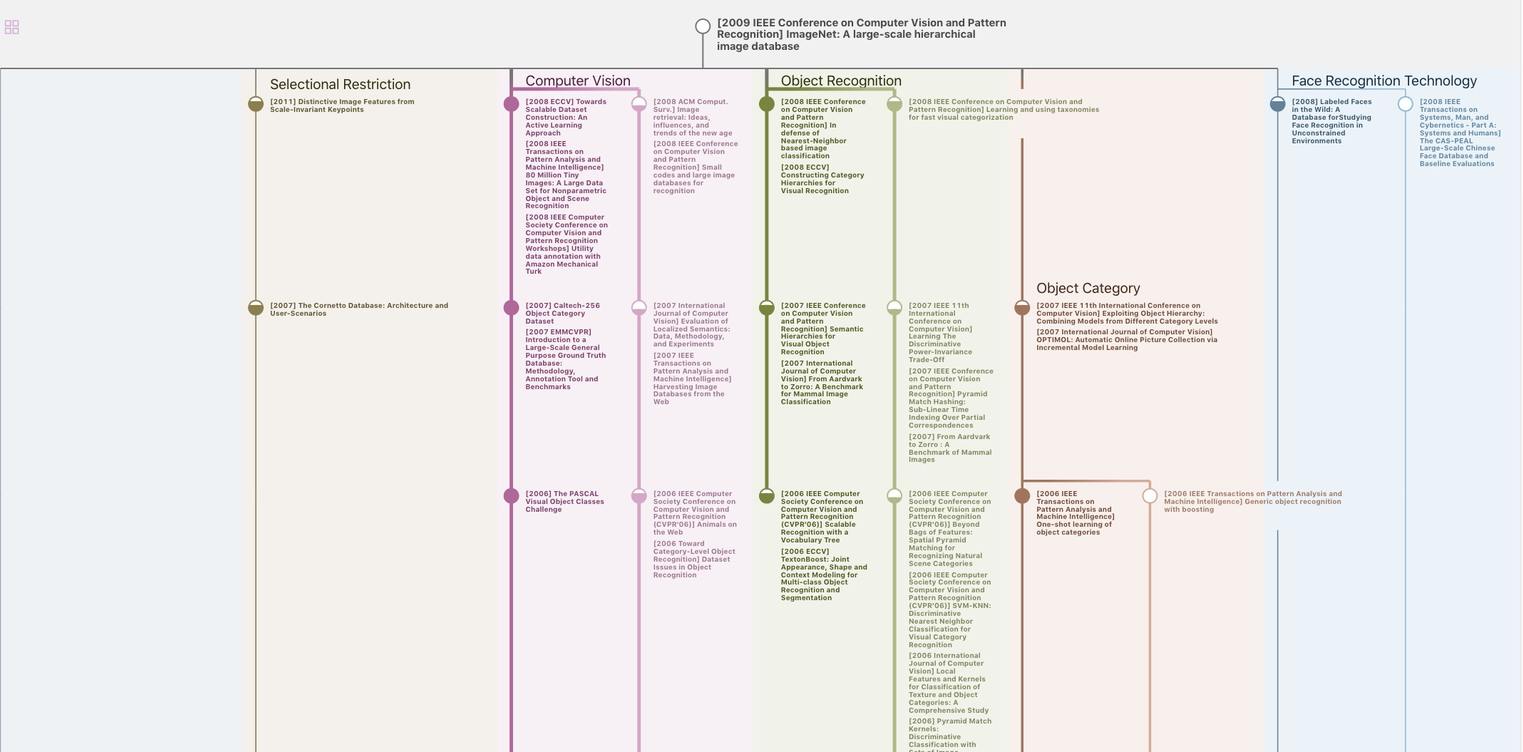
Generate MRT to find the research sequence of this paper
Chat Paper
Summary is being generated by the instructions you defined