Event-triggered multi-agent credit allocation pursuit-evasion algorithm
NEURAL PROCESSING LETTERS(2022)
摘要
The reinforcement learning is used to study the problem of multi-agent pursuit-evasion games in this article. The main problem of current reinforcement learning applied to multi-agents is the low learning efficiency of agents. To solve this problem, a credit allocation mechanism is adopted in the Multi-agent Deep Deterministic Policy Gradient frame (hereinafter referred to as the MADDPG), the core idea of which is to enable individuals who contribute more to the group to occupy a higher degree of dominance in subsequent training iterations. An event-triggered mechanism is utilized for the simplification of calculation. An observer is set for the feedback value, and the credit allocation algorithm is activated only when the observer believes that the agent group is in a local optimal training dilemma. The final simulation and experiment show that, In most cases, the event-triggered multiagent credit allocation algorithm (hereinafter referred to as the EDMCA algorithm) obtained better results and discussed the parameter settings of the observer.
更多查看译文
关键词
Multi-Agent Credit Allocation, Event Trigger, Multi-agent Pursuit-Evasion Games
AI 理解论文
溯源树
样例
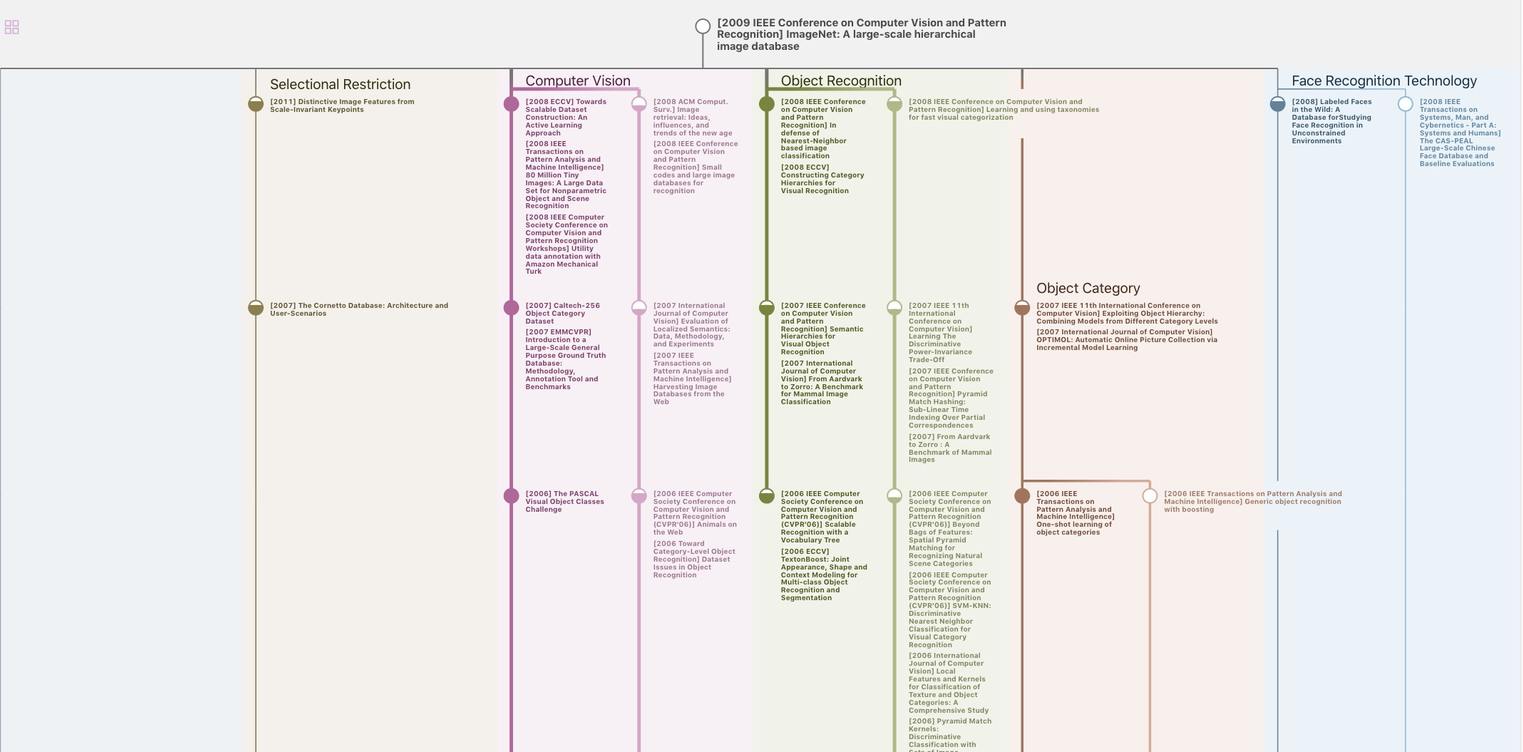
生成溯源树,研究论文发展脉络
Chat Paper
正在生成论文摘要