Predicting Protein-DNA Binding Sites by Fine-Tuning BERT
INTELLIGENT COMPUTING THEORIES AND APPLICATION, ICIC 2022, PT II(2022)
摘要
The study of Protein-DNA binding sites is one of the fundamental problems in genome biology research. It plays an important role in understanding gene expression and transcription, biological research, and drug development. In recent years, language representation models have had remarkable results in the field of Natural Language Processing (NLP) and have received extensive attention from researchers. Bidirectional Encoder Representations for Transformers (BERT) has been shown to have state-of-the-art results in other domains, using the concept of word embedding to capture the semantics of sentences. In the case of small datasets, previous models often cannot capture the upstream and downstream global information of DNA sequences well, so it is reasonable to refer the BERT model to the training of DNA sequences. Models pre-trained with large datasets and then fine-tuned with specific datasets have excellent results on different downstream tasks. In this study, firstly, we regard DNA sequences as sentences and tokenize them using K-mer method, and later utilize BERT to matrix the fixed length of the tokenized sentences, perform feature extraction, and later perform classification operations. We compare this method with current state-of-the-art models, and the DNABERT method has better performance with average improvement 0.013537, 0.010866, 0.029813, 0.052611, 0.122131 in ACC, F1-score, MCC, Precision, Recall, respectively. Overall, one of the advantages of BERT is that the pre-training strategy speeds up the convergence in the network in migration learning and improves the learning ability of the network. DNABER model has advantageous generalization ability on other DNA datasets and can be utilized on other sequence classification tasks.
更多查看译文
关键词
Protein-DNA binding sites, Transcription factor, Traditional machine learning, Deep learning, Transformers, BERT
AI 理解论文
溯源树
样例
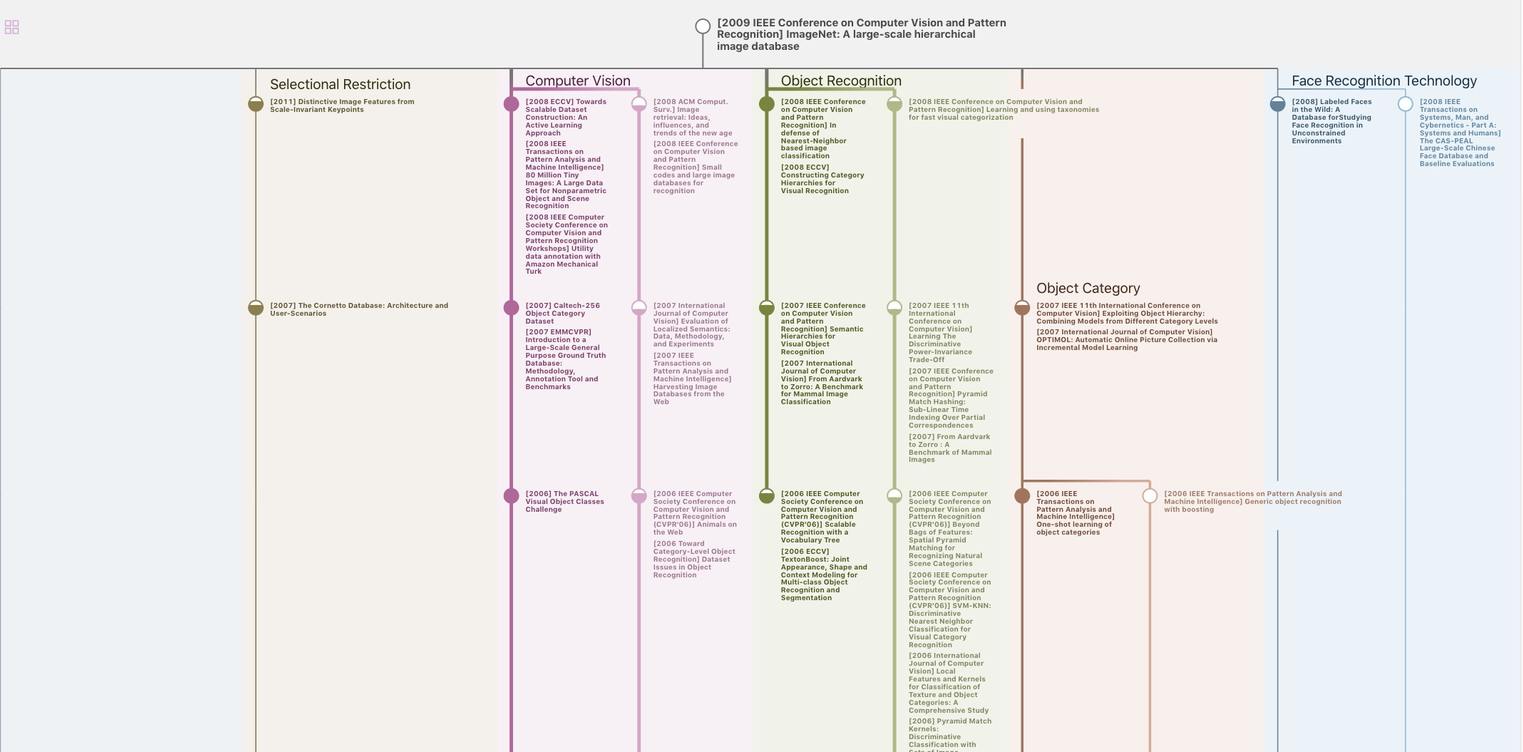
生成溯源树,研究论文发展脉络
Chat Paper
正在生成论文摘要