Prediction of MiRNA-Disease Association Based on Higher-Order Graph Convolutional Networks
INTELLIGENT COMPUTING THEORIES AND APPLICATION, ICIC 2022, PT II(2022)
摘要
A large number of studies have shown that the formation and development of diseases are closely related to the abnormal expression of miRNAs. Studying the association between miRNAs and diseases can not only effectively prevent but also be beneficial to treat the disease. However, traditional biological experiments need a lot of manpower, material resources. Therefore, for exploring the potential associations between miRNAs and diseases, we proposed a new model called MIXHOPMDA. First, we construct heterogeneous bipartite graph of miRNA and disease, and ensure feature vectors of miRNA and disease are projected into the same dimensional vector space. Second, we use the delta operator to operate on different powers of different adjacency matrices simultaneously. Thirdly, we obtain the scoring matrix through the fully connected layer and then select the specified threshold to judge the possibility of the potential association between miRNA and disease. On HMDD v2.0, the average AUC of our model MIXHOPMDA based on 5-fold cross-validation is 93.37%. We conduct case studies on esophageal neoplasms and finally found that 47 of the top 50 miRNAs are verified by dbDEMC or miR2Disease. In conclusion, MIXHOPMDA can be used as a new model to predict the potential associations between miRNAs and diseases. The source code and related datasets can be obtained from https://github.com/SouthLion/MXHOPMDA.
更多查看译文
关键词
MiRNA-disease associations, DropNode, Graph convolutional networks, Delta operator
AI 理解论文
溯源树
样例
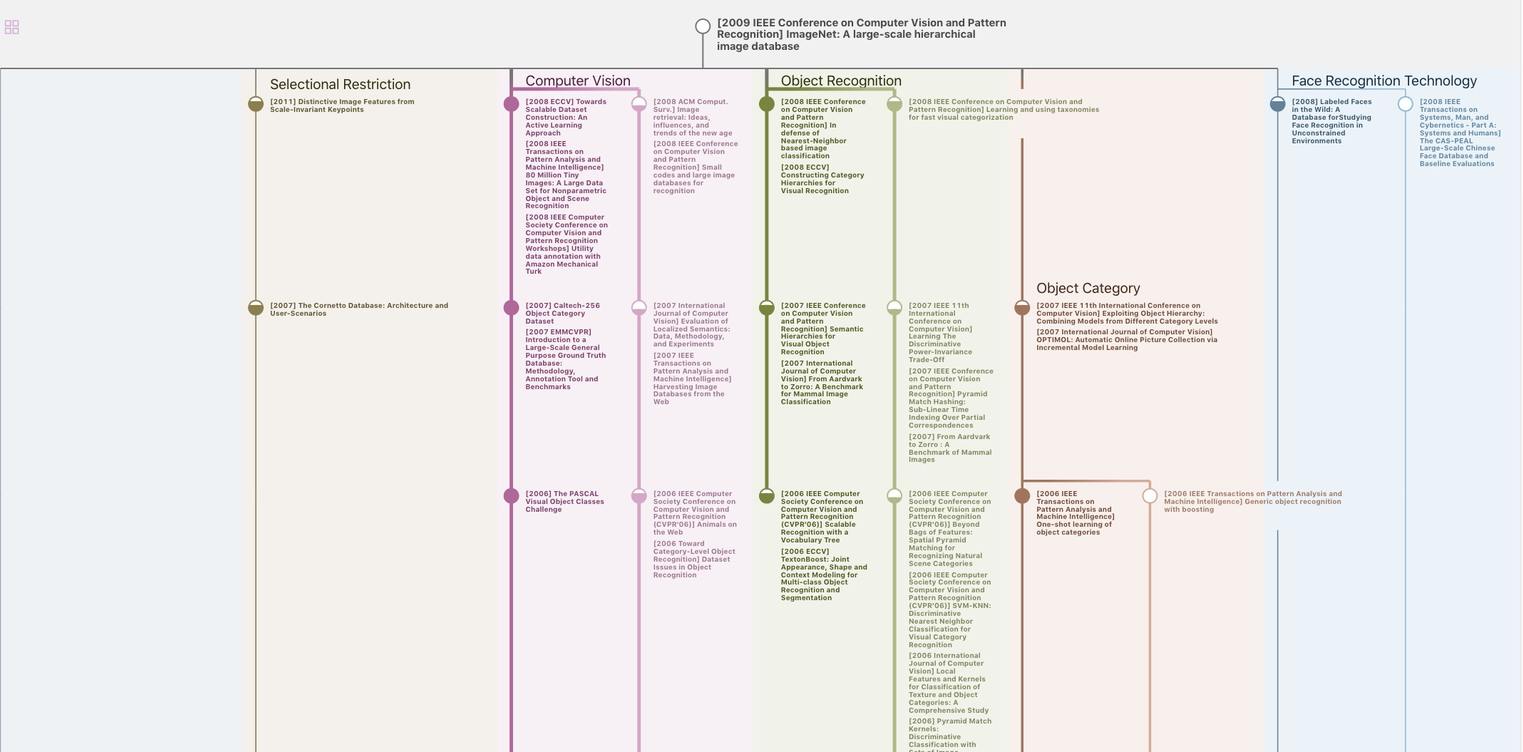
生成溯源树,研究论文发展脉络
Chat Paper
正在生成论文摘要