Prediction of Element Distribution in Cement by CNN
INTELLIGENT COMPUTING THEORIES AND APPLICATION, ICIC 2022, PT II(2022)
摘要
Cement-based materials are widely used in today's society. Their quality directly determines the quality of buildings. Therefore, it is urgent to improve the physical properties of cement. To study high-performance cement, researchers commonly use a scanning electron microscope for element analysis to explore the reasons that affect the performance of cement. A scanning electron microscope (SEM) is a common tool to study materials' internal microstructure and physical properties. It can obtain high-quality images, so it has many applications. Nevertheless, it also has some inherent disadvantages: Firstly, its cost is expensive, and many scientific research institutions do not have such strong economic strength; Secondly, even if it can be rented, it tends to consume considerable time and economic cost, which causes difficulties for the researchers. To solve the above problems, we have made a lot of attempts and finally adopted deep learning to solve this problem. Deep learning, the most widely used technology in artificial intelligence, developed rapidly and its related technology is widely used in material science. In this paper, we make full use of the advantages of deep learning and propose a new method to analyze the element distribution in cement for simple and rapid element analysis. This method implements the function similar to that of scanning electron microscope. It adopts convolutional neural networks and takes the cement backscattered electron (BSE) image as input. After the input image passes through the convolutional neural networks, the distribution map of some elements are obtained. Therefore, we can obtain the distribution of elements of interest in cement samples through this method. Our method can realize the rapid element analysis of cement and reduce the time and money cost of analyzing elements in physical experiments.
更多查看译文
关键词
Cement element prediction, Convolutional neural network, Energy Dispersive Spectroscopy Prediction
AI 理解论文
溯源树
样例
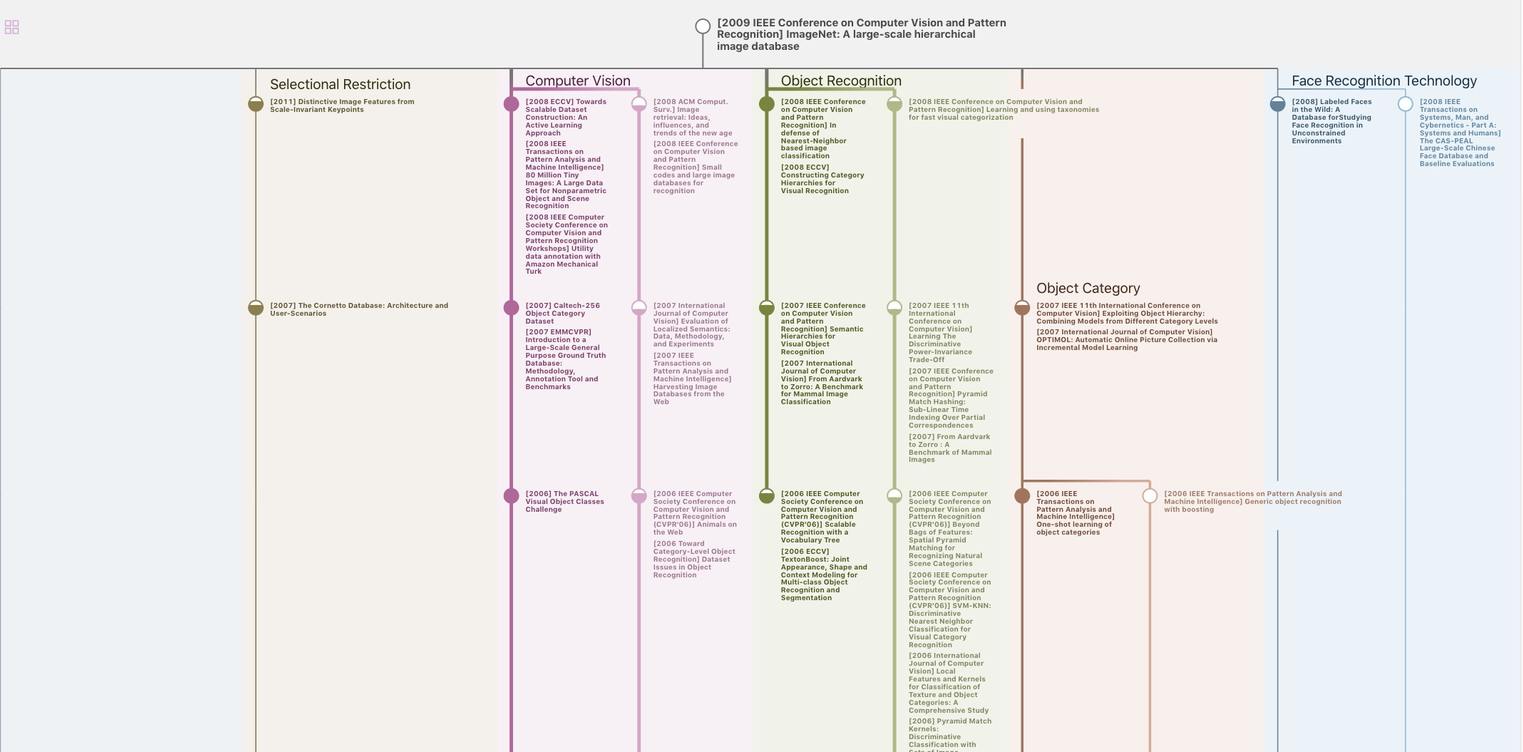
生成溯源树,研究论文发展脉络
Chat Paper
正在生成论文摘要