Predicting Drug-Disease Associations via Meta-path Representation Learning based on Heterogeneous Information Net works
INTELLIGENT COMPUTING THEORIES AND APPLICATION, ICIC 2022, PT II(2022)
摘要
Identifying new indications of drugs plays an important role in the drug research and development process. However, traditional methods are labor-intensive and financially demanding to discover new indications. Computational methods are regarded as an effective way to predict underlying drug-disease associations (DDAs). Therefore it is a great urgent to develop computational-based methods to improve the accuracy of DDAs prediction. In this paper, a novel Meta-path Representation Learning-based model called MRLDDA is proposed to predict new DDAs on a heterogeneous information network (HIN). Specifically, MRLDDA first constructs a meta-path strategy based on rich HIN, i.e., drug-protein-disease-drug, and then the network representation of drugs and diseases is obtained by a heterogeneous representation model. Finally, a typical machine learning strategy-random forest classifier is applied to solve the prediction task of DDAs. Experimental results on the two benchmark datasets show that MRLDDA has a better prediction performance for the new DDAs under ten-fold cross-validation, with AUC of 0.8427 on B-Dataset and 0.9482 on F-Dataset.
更多查看译文
关键词
Drug-disease associations, Heterogeneous information network, Meta-path generation strategy, Drugs, Diseases
AI 理解论文
溯源树
样例
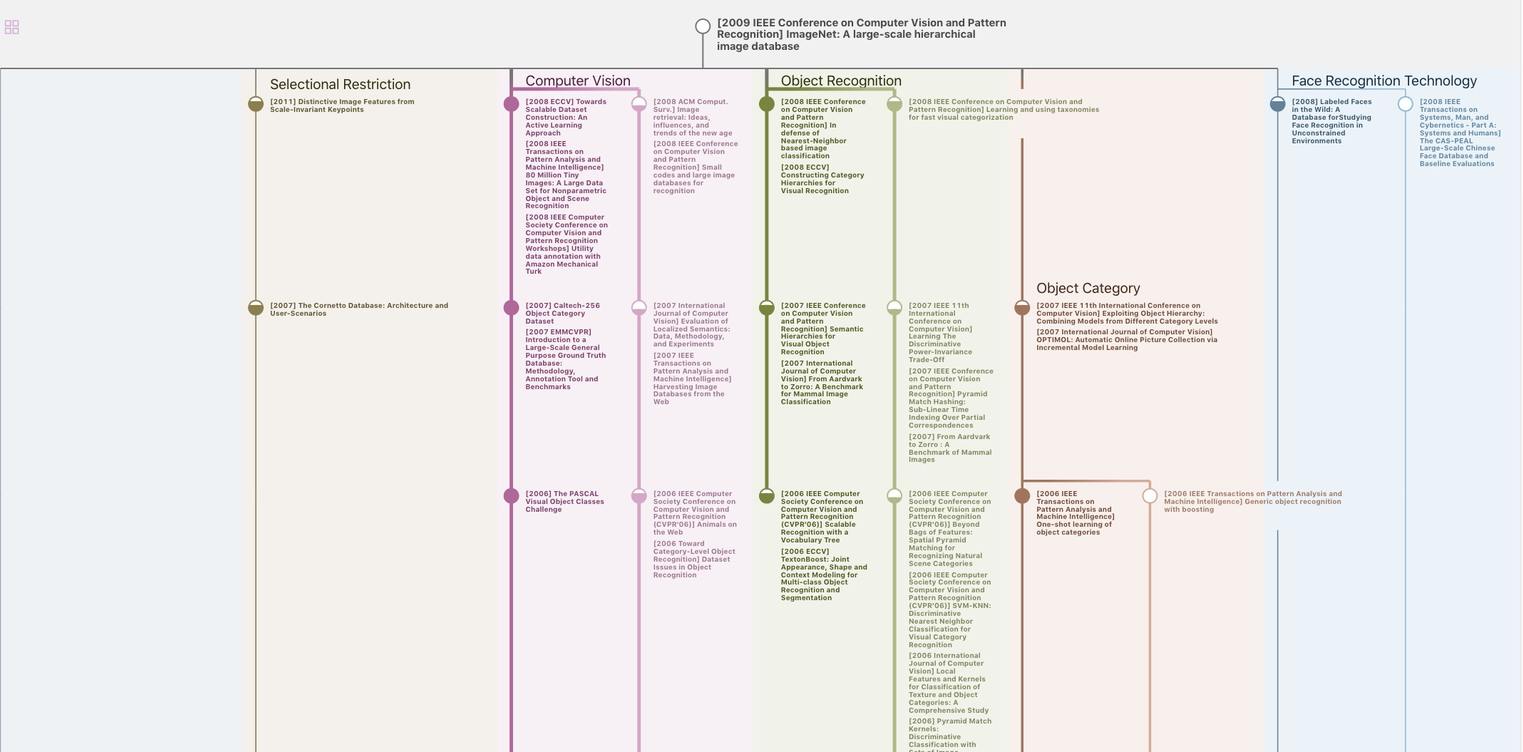
生成溯源树,研究论文发展脉络
Chat Paper
正在生成论文摘要