Efficient Transfer Learning for Visual Tasks via Continuous Optimization of Prompts
IMAGE ANALYSIS AND PROCESSING, ICIAP 2022, PT I(2022)
摘要
Traditional methods for adapting pre-trained vision models to downstream tasks involve fine-tuning some or all of the model's parameters. There are a number of trade-offs with this approach. When too many parameters are fine-tuned, the model may lose the benefits associated with pre-training, such as the ability to generalize to out-of-distribution data. But, if instead too few parameters are fine-tuned, the model may be unable to adapt effectively for the tasks downstream. In this paper, we propose Visual Prompt Tuning (VPT) as an alternative to fine-tuning for Transformer-based vision models. Our method is closely related to, and inspired by, prefix-tuning of language models [22]. We find that, by adding additional parameters to a pre-trained model, VPT offers similar performance to fine-tuning the final layer. In addition, for low-data settings and for specialized tasks, such as traffic sign recognition, satellite photo recognition and handwriting classification, the performance of Transformer-based vision models is improved with the use of VPT.
更多查看译文
关键词
Computer vision, Few-shot, Fine-tuning, Prompt engineering, Prefix-tuning, CLIP, Transformers, Vision transformers
AI 理解论文
溯源树
样例
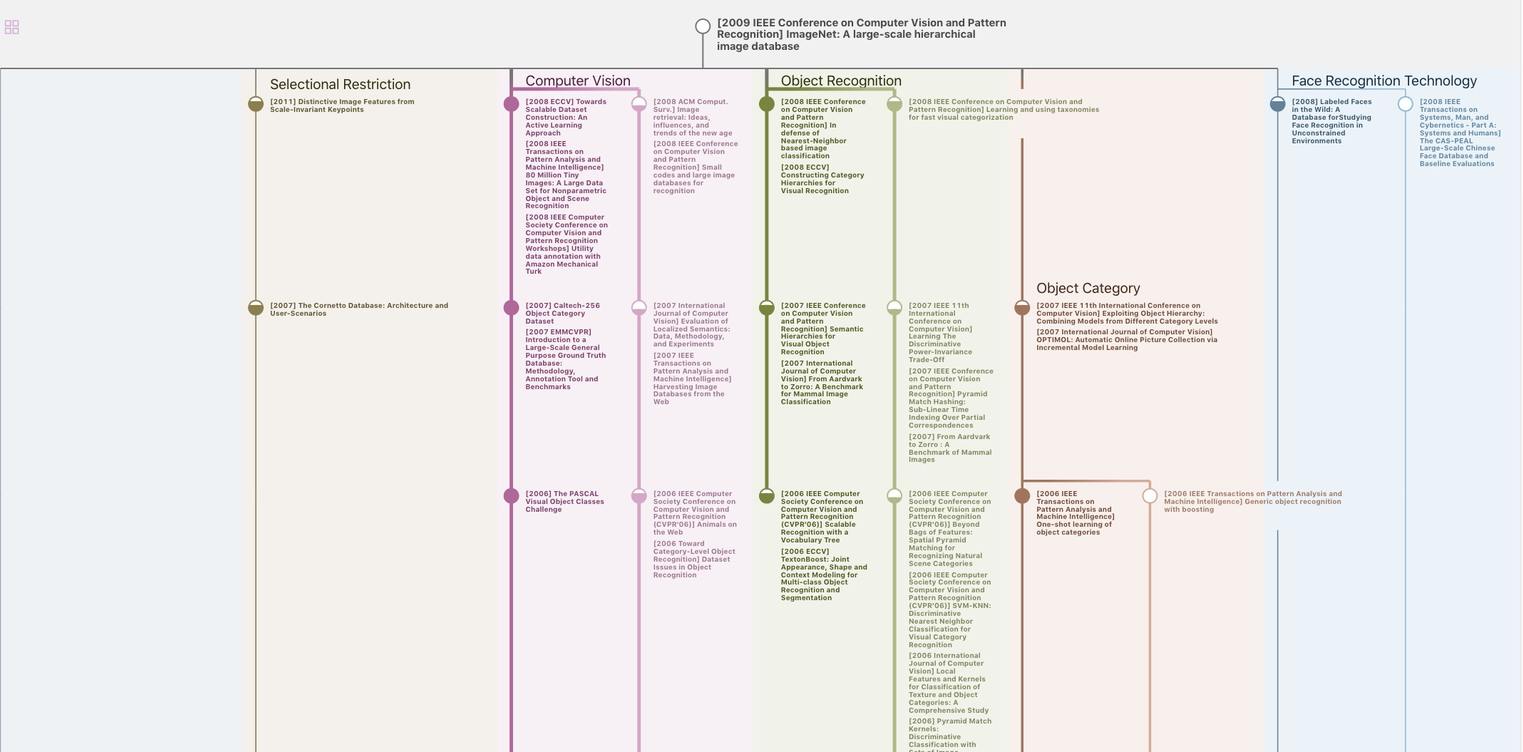
生成溯源树,研究论文发展脉络
Chat Paper
正在生成论文摘要