USIS: A unified semantic image synthesis model trained on a single or multiple samples
Neurocomputing(2022)
摘要
Semantic image synthesis methods learn to generate new images conditioned on predefined semantic label maps. Existing methods require access to large-volume samples labeled with semantic maps, which limits their applications. We propose USIS, a Unified Semantic Image Synthesis model which can be trained on only a single or multiple pairs of images and semantic maps. Once trained, a USIS model can generate new images according to unseen semantic maps, as existing semantic image synthesis methods do. Specifically, we design a hierarchical architecture to reconstruct training samples and gradually learn the distributions of multi-scale patches in samples from coarse to fine. To avoid the error accumulation across scales, we propose a mixed training strategy to stabilize the training process. Extensive experiments on one- or multiple-sample datasets show our proposed model achieves state-of-the-art performance in terms of visual fidelity.
更多查看译文
关键词
Semantic image generation,Image translation,Single-image generative models
AI 理解论文
溯源树
样例
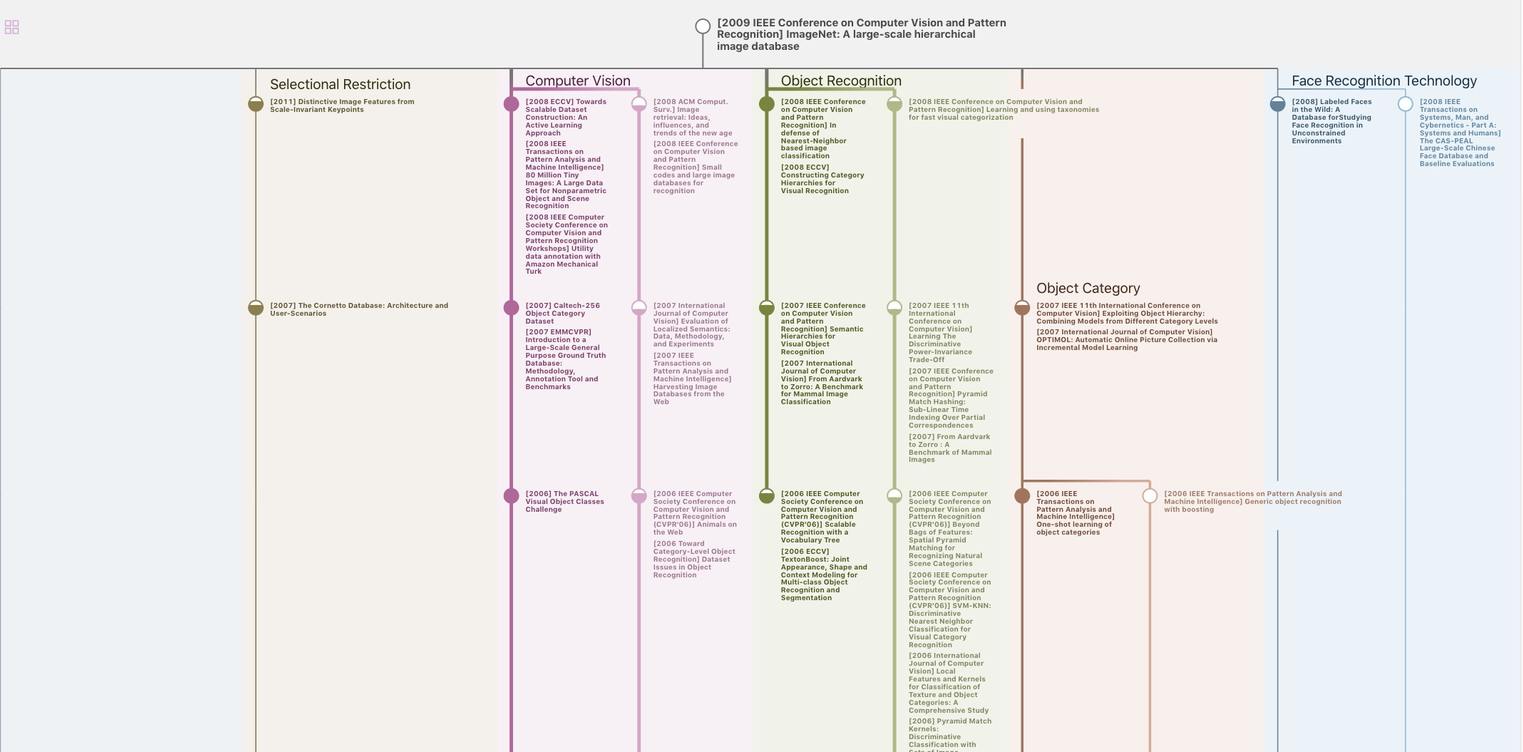
生成溯源树,研究论文发展脉络
Chat Paper
正在生成论文摘要