Sparse and Topological Coding for Visual Localization of Autonomous Vehicles
FROM ANIMALS TO ANIMATS 16(2022)
Abstract
Efficient encoding of visual information is essential to the success of vision-based navigation tasks in large-scale environments. To do so, we propose in this article the Sparse Max-Pi neural network (SMP), a novel compute-efficient model of visual localization based on sparse and topological encoding of visual information. Inspired by the spatial cognition of mammals, the model uses a "topologic sparse dictionary" to efficiently compress the visual information of a landmark, allowing rich visual information to be represented with very small codes. This descriptor, inspired by the neurons in the primary visual cortex (V1), are learned using sparse coding, homeostasis and self-organising map mechanisms. Evaluated in cross-validation on the Oxford-car dataset, our experimental results show that the SMP model is competitive with the state of the art. It thus provides comparable or better performance than CoHog and NetVlad, two state-of-the-art VPR models.
MoreTranslated text
Key words
Visual place recognition, Sparse coding, Autonomous vehicle, Visual cortex, Bio-inspired robotics
AI Read Science
Must-Reading Tree
Example
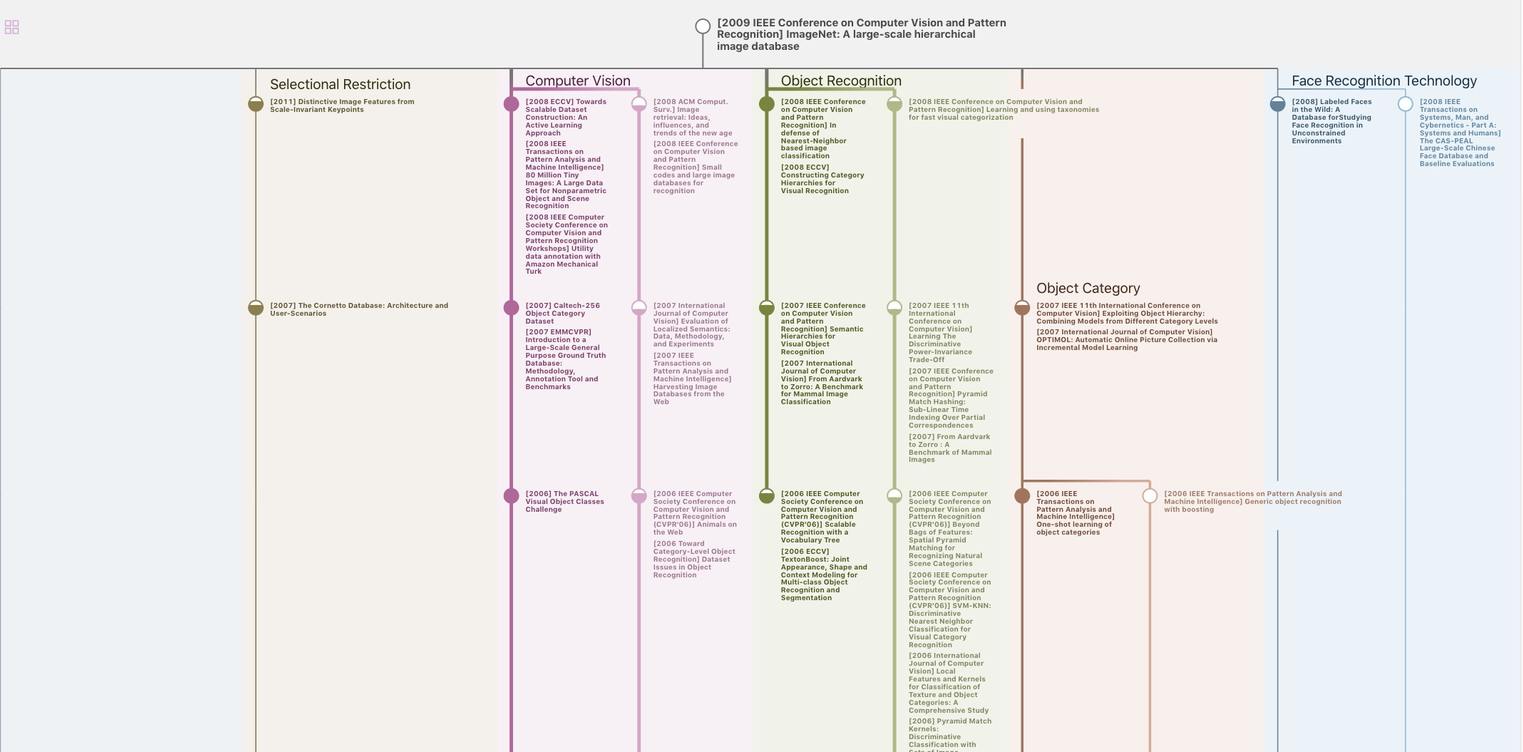
Generate MRT to find the research sequence of this paper
Chat Paper
Summary is being generated by the instructions you defined