Drone Surveillance Using Detection, Tracking and Classification Techniques
IMAGE ANALYSIS AND PROCESSING, ICIAP 2022 WORKSHOPS, PT II(2022)
摘要
In this work, we explore the process of designing a long-term drone surveillance system by fusing object detection, tracking and classification methods. Given a video stream from an RGB-camera, a detection module based on YOLOV5 is trained for finding drones within its field of view. Although in drone detection, high accuracy and robustness is achieved with the underlying complex architecture, the detection speed is hindered on ultra HD-streams. To solve this problem, we integrate a high efficient object tracker to update target status while avoiding running the detection at each frame. Benefited from lightweight backbone networks with powerful Transformer design, the object tracker achieves real-time speed on standalone CPU devices. Moreover, a drone classification model is applied on the output of the detection and tracking mechanisms to further distinguish drones from other background distractors (birds, balloons). By leveraging inference optimization with TensorRT and ONNX, our system achieves extremely high inference speed on NVIDIA GPUs. A ROS package is designed to integrate the aforementioned components together and provide a flexible, end-to-end drone surveillance tool for real-time applications. Comprehensive experiments on both standard benchmarks and field tests demonstrate the effectiveness and stability of proposed system.
更多查看译文
关键词
Drone detection and classification, Object tracking, Super resolution
AI 理解论文
溯源树
样例
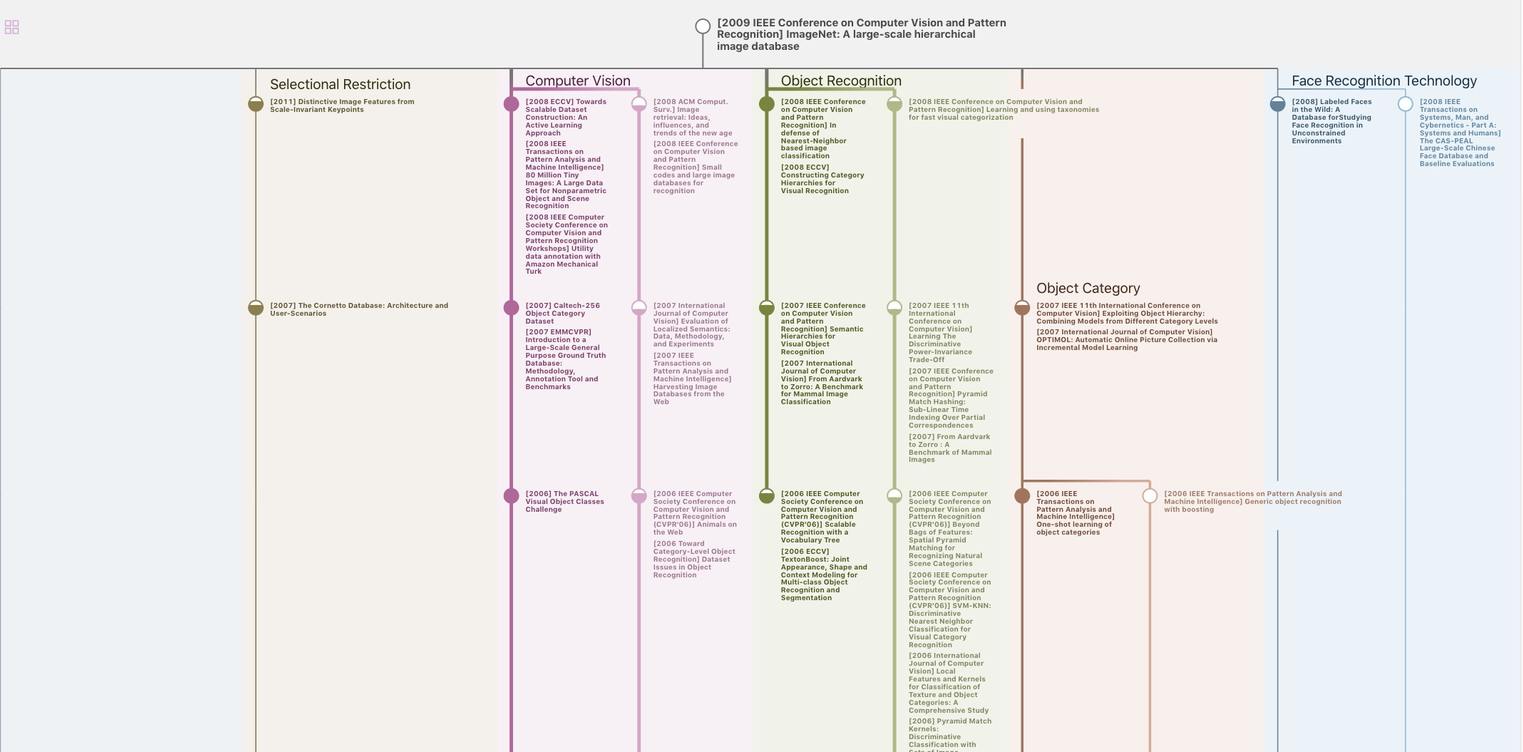
生成溯源树,研究论文发展脉络
Chat Paper
正在生成论文摘要