Global Surrogate Modeling by Neural Network-Based Model Uncertainty
COMPUTATIONAL SCIENCE - ICCS 2022, PT III(2022)
摘要
This work proposes a novel adaptive global surrogate modeling algorithm which uses two neural networks, one for prediction and the other for the model uncertainty. Specifically, the algorithm proceeds in cycles and adaptively enhances the neural network-based surrogate model by selecting the next sampling points guided by an auxiliary neural network approximation of the spatial error. The proposed algorithm is tested numerically on the one-dimensional Forrester function and the two-dimensional Branin function. The results demonstrate that global surrogate modeling using neural network-based function prediction can be guided efficiently and adaptively using a neural network approximation of the model uncertainty.
更多查看译文
关键词
Global surrogate modeling, Neural networks, Model uncertainty, Error based exploration
AI 理解论文
溯源树
样例
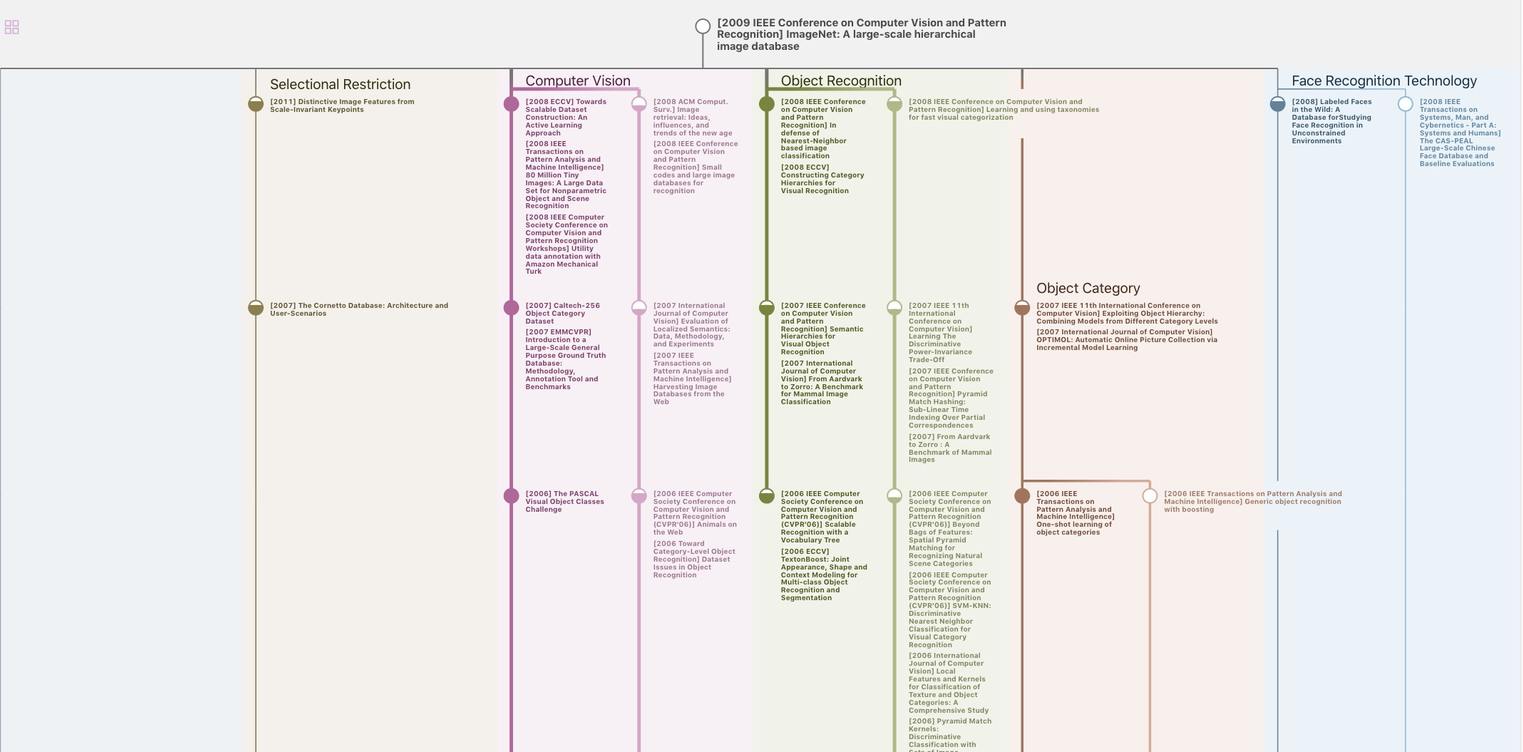
生成溯源树,研究论文发展脉络
Chat Paper
正在生成论文摘要