Swin Faster R-CNN for Senescence Detection of Mesenchymal Stem Cells in Bright-Field Images
MEDICAL OPTICAL IMAGING AND VIRTUAL MICROSCOPY IMAGE ANALYSIS, MOVI 2022(2022)
摘要
iPSC-derived mesenchymal stem cells (iMSCs) can differentiate into a wide range of mesodermal cell types for tissue repair, and play an important role in cell therapy in regenerative medicine. The therapeutic effect of mesenchymal stem cells (MSCs) is largely affected by their differentiation ability and cell activity, and the therapeutic effect of senescent cells is often worse than that of young cells. Biologically senescent detection of iMSCs can only be performed by vital staining, which is time-consuming, labor-intensive, and financial-intensive. To solve this problem, we apply a deep learning method to the senescence identification research of iMSCs to achieve the purpose of rapid and accurate detection without vital staining. We propose a Swin Transformer-based Faster R-CNN network for senescence identification ofMSCs in bright-field images. In particular, we exploit the self-attention mechanism of the Swin Transformer to extract features of dense cell regions in the picture, enabling the network to learn the strong discriminative properties of different classes of cells. In addition, we perform multi-scale fusion on the features extracted by the backbone network to address the problem of inconsistent cell sizes in the data. Experiments on self-collected datasets show that our method achieves good performance on each generation of datasets and achieves the highest detection precision (mAP) of 0.816 on our collected mixed datasets.
更多查看译文
关键词
Mesenchymal stem cells, Cell senescent detection, Bright-field microscopy image, Swin transformer, Faster R-CNN
AI 理解论文
溯源树
样例
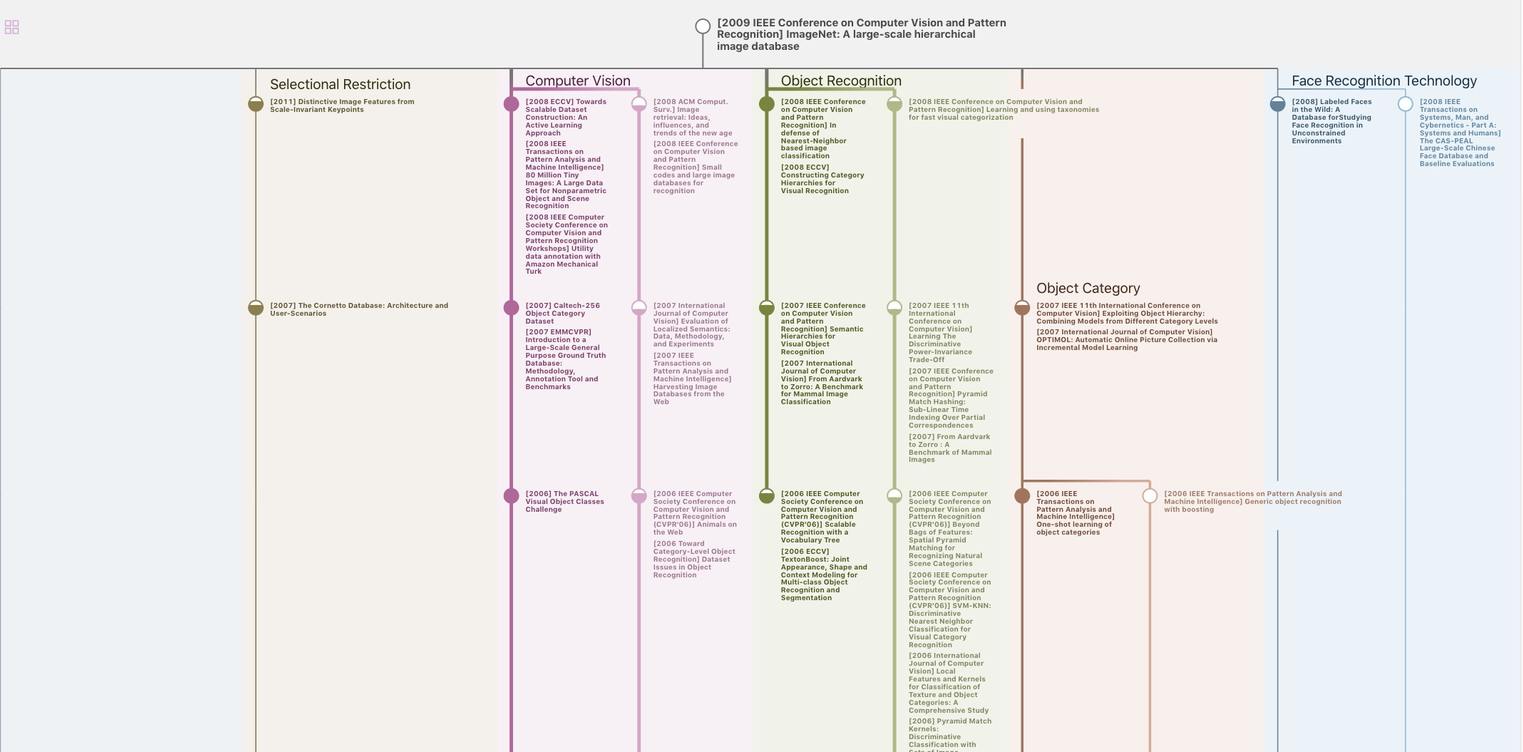
生成溯源树,研究论文发展脉络
Chat Paper
正在生成论文摘要