Exploiting high-order behaviour patterns for cross-domain sequential recommendation
CONNECTION SCIENCE(2022)
摘要
The cross-domain sequential recommendation aims to predict the next item based on a sequence of recorded user behaviours in multiple domains. We propose a novel Cross-domain Sequential Recommendation approach with Graph-Collaborative Filtering (CsrGCF) to alleviate the sparsity issue of user-interaction data. Specifically, we design time-aware and relation-aware graph attention mechanisms with collaborative filtering to exploit high-order behaviour patterns of users for promising results in both domains. Time-aware Graph Attention mechanism (TGAT) is designed to learn the inter-domain sequence-level representation of items. Relationship-aware Graph Attention mechanism (RGAT) is proposed to learn collaborative items' and users' feature representations. Moreover, to simultaneously improve the recommendation performance in the two domains, a Cross-domain Feature Bidirectional Transfer module (CFBT) is proposed, transferring user's common sharing features in both domains and retaining user's domain-specific features in a specific domain. Finally, cross-domain and sequential information jointly recommend the next items that users like. We conduct extensive experiments on two real-world datasets that show that CsrGCF outperforms several state-of-the-art baselines in terms of Recall and MRR. These demonstrate the necessity of exploiting high-order behaviour patterns of users for a cross-domain sequential recommendation. Meanwhile, retaining domain-specific features is an important step in the process of cross-domain feature bidirectional transferring.
更多查看译文
关键词
Cross-domain sequential recommendation, graph-collaborative filtering, data sparsity, graph attention mechanism
AI 理解论文
溯源树
样例
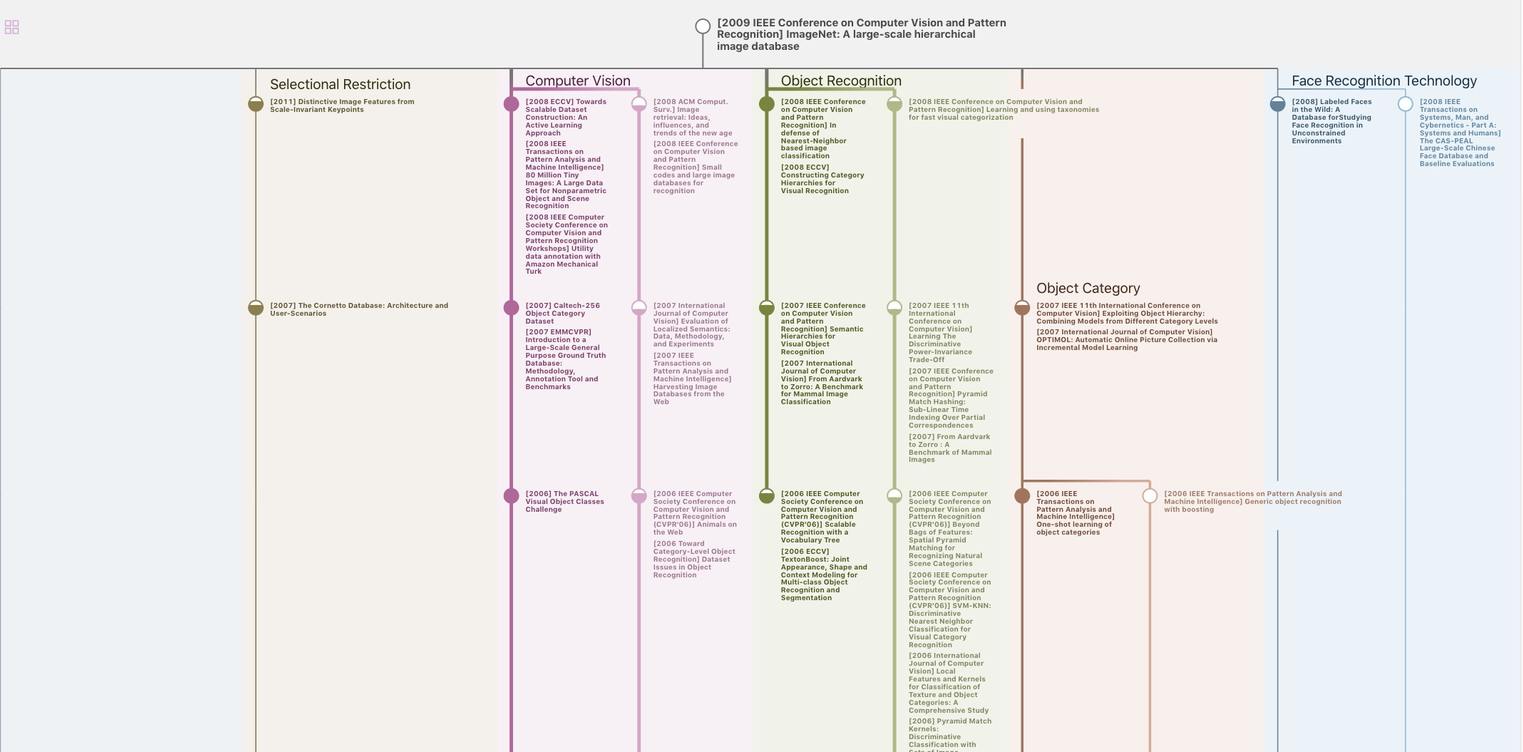
生成溯源树,研究论文发展脉络
Chat Paper
正在生成论文摘要