A weakly supervised knowledge attentive network for aspect-level sentiment classification
JOURNAL OF SUPERCOMPUTING(2022)
摘要
Deep neural networks have achieved good performance in recent years for aspect-level sentiment classification (ASC), whereas most neural ASC models neglect the commonsense knowledge absent from text but essential for aspect affective understanding, which largely limits the performance of neural ASC. In this paper, we propose a Weakly Supervised Knowledge Attentive Network, which resolves the above problems via knowledge attention and weakly supervised learning. Specifically, we first present a Knowledge Attentive Network (KAN) to capture more aspect-related information by incorporating external commonsense knowledge into the attention mechanism. Then, we propose a weakly supervised learning method, which allows our KAN model to learn more knowledge from the pseudo-samples generated upon the rich-resource document-level sentiment classification datasets. Extensive experiments on four benchmark datasets show the significant advantages of our proposed approach. In particular, we obtain state-of-the-art performance in terms of accuracy on all the datasets.
更多查看译文
关键词
Sentiment analysis, Knowledge attentive network, Aspect-level sentiment analysis
AI 理解论文
溯源树
样例
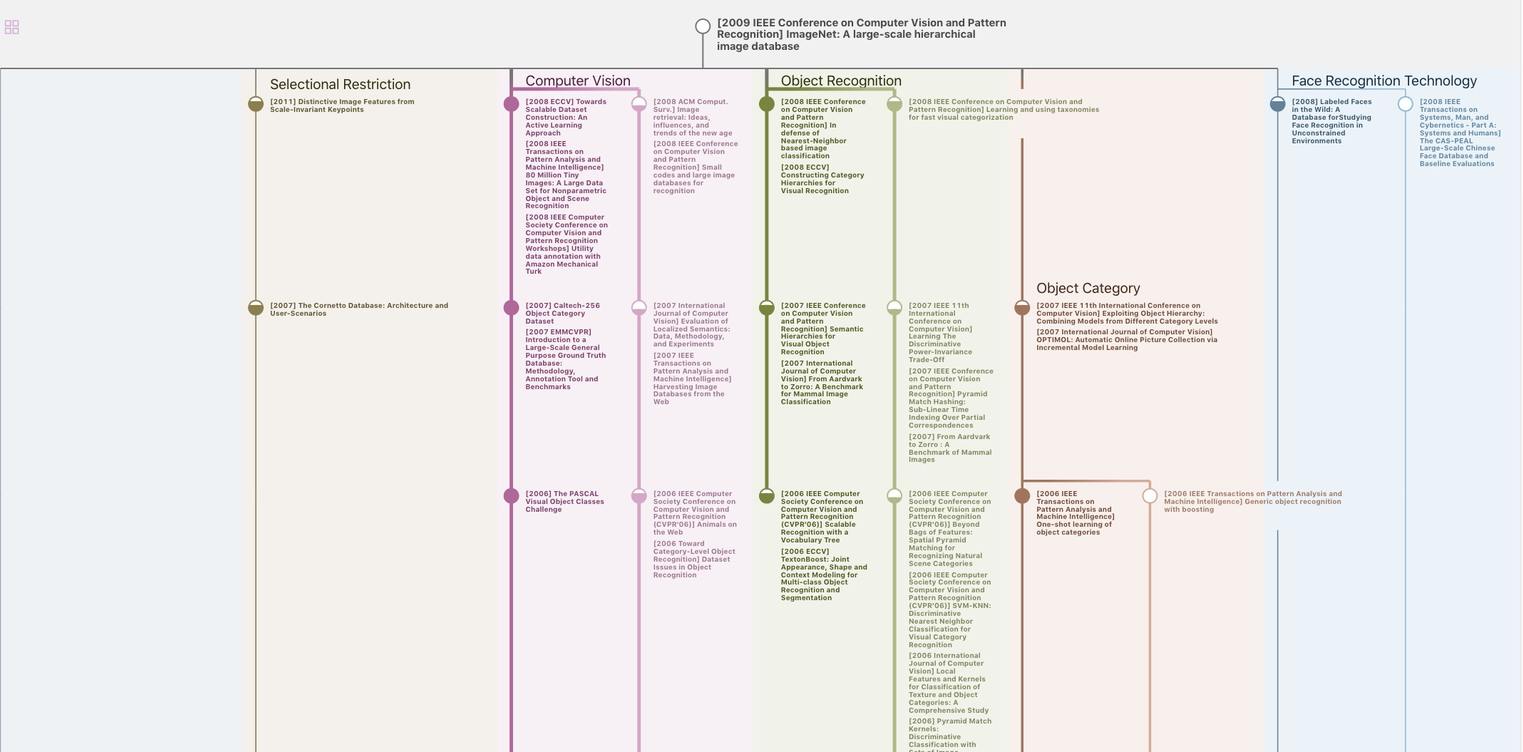
生成溯源树,研究论文发展脉络
Chat Paper
正在生成论文摘要