Which Visual Features Impact the Performance of Target Task in Self-supervised Learning?
COMPUTATIONAL SCIENCE - ICCS 2022, PT I(2022)
摘要
Self-supervised methods gain popularity by achieving results on par with supervised methods using fewer labels. However, their explaining techniques ignore the general semantic concepts present in the picture, limiting to local features at a pixel level. An exception is the visual probing framework that analyzes the vision concepts of an image using probing tasks. However, it does not explain if analyzed concepts are critical for target task performance. This work fills this gap by introducing amnesic visual probing that removes information about particular visual concepts from image representations and measures how it affects the target task accuracy. Moreover, it applies Marr's computational theory of vision to examine the biases in visual representations. As a result of experiments and user studies conducted for multiple self-supervised methods, we conclude, among others, that removing information about 3D forms from the representation decrease classification accuracy much more significantly than removing textures.
更多查看译文
关键词
Explainability, Self-supervision, Probing tasks
AI 理解论文
溯源树
样例
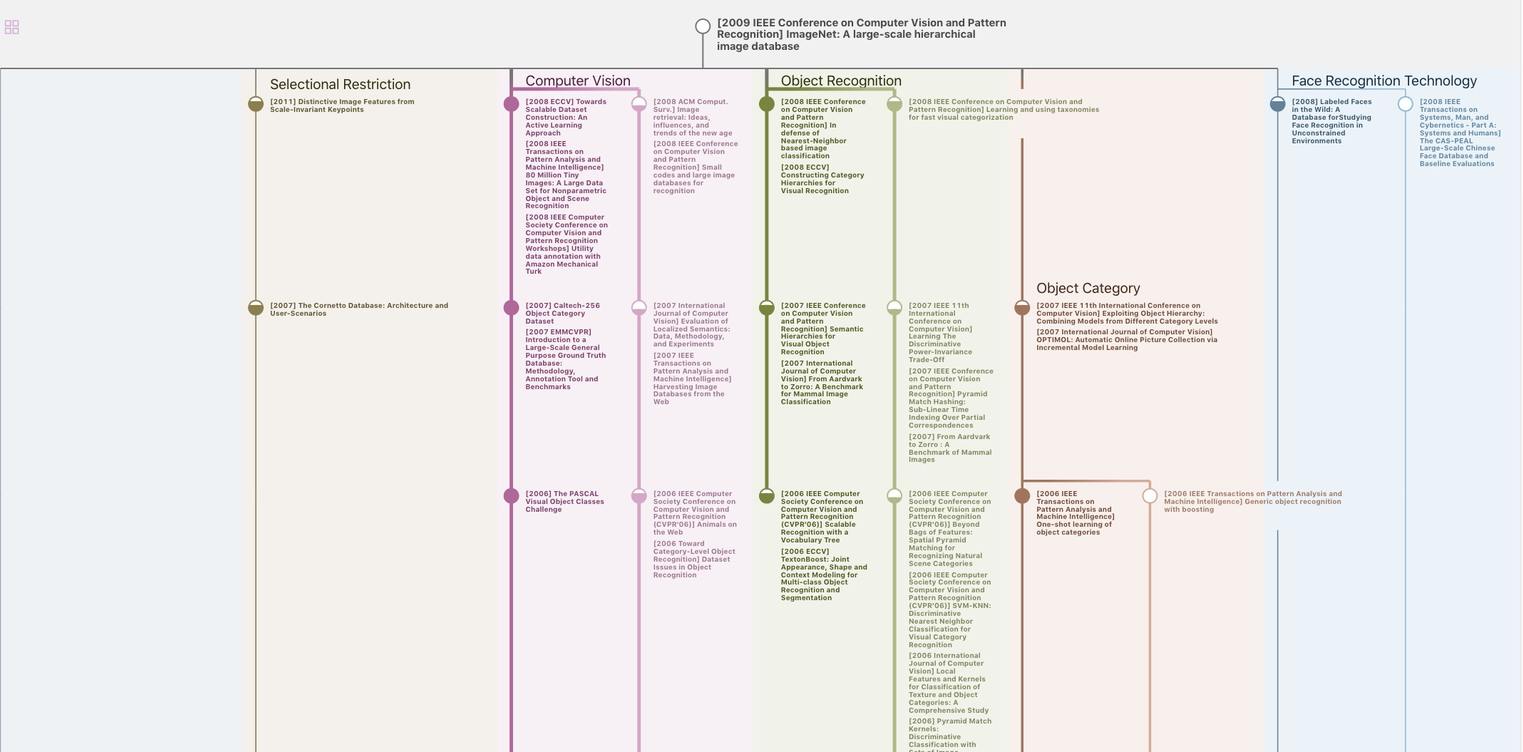
生成溯源树,研究论文发展脉络
Chat Paper
正在生成论文摘要