Feature Selection Optimized by the Artificial Immune Algorithm Based on Genome Shuffling and Conditional Lethal Mutation
Applied intelligence(2022)
摘要
Improving classification performance is an essential goal for various practical applications. Feature selection has become an important data preprocessing step in machine learning systems. However, many effective methods based on heuristic search strategies have the problem of high running costs. This paper proposes an efficient multiobjective feature selection method based on artificial immune algorithm optimization. It introduces a clone selection algorithm to explore the search space of optimal feature subsets. According to the target requirements of feature selection, combined with biological research results, this method introduces genome shuffling technology and a conditional lethal mutation mechanism to improve the search performance of the algorithm. Experimental comparisons are conducted on 21 benchmark datasets with 17 advanced feature selection methods in terms of classification accuracy, the number of feature subsets, and computational cost. The results show that the algorithm achieves the smallest number of selected features (only 3.26% compared to the lowest) and better average classification accuracy with a much lower average computational cost than others (only 3.62% compared to the lowest).
更多查看译文
关键词
Feature selection,Dimensionality reduction,Multiobjective optimization,Artificial immune algorithm,Classification
AI 理解论文
溯源树
样例
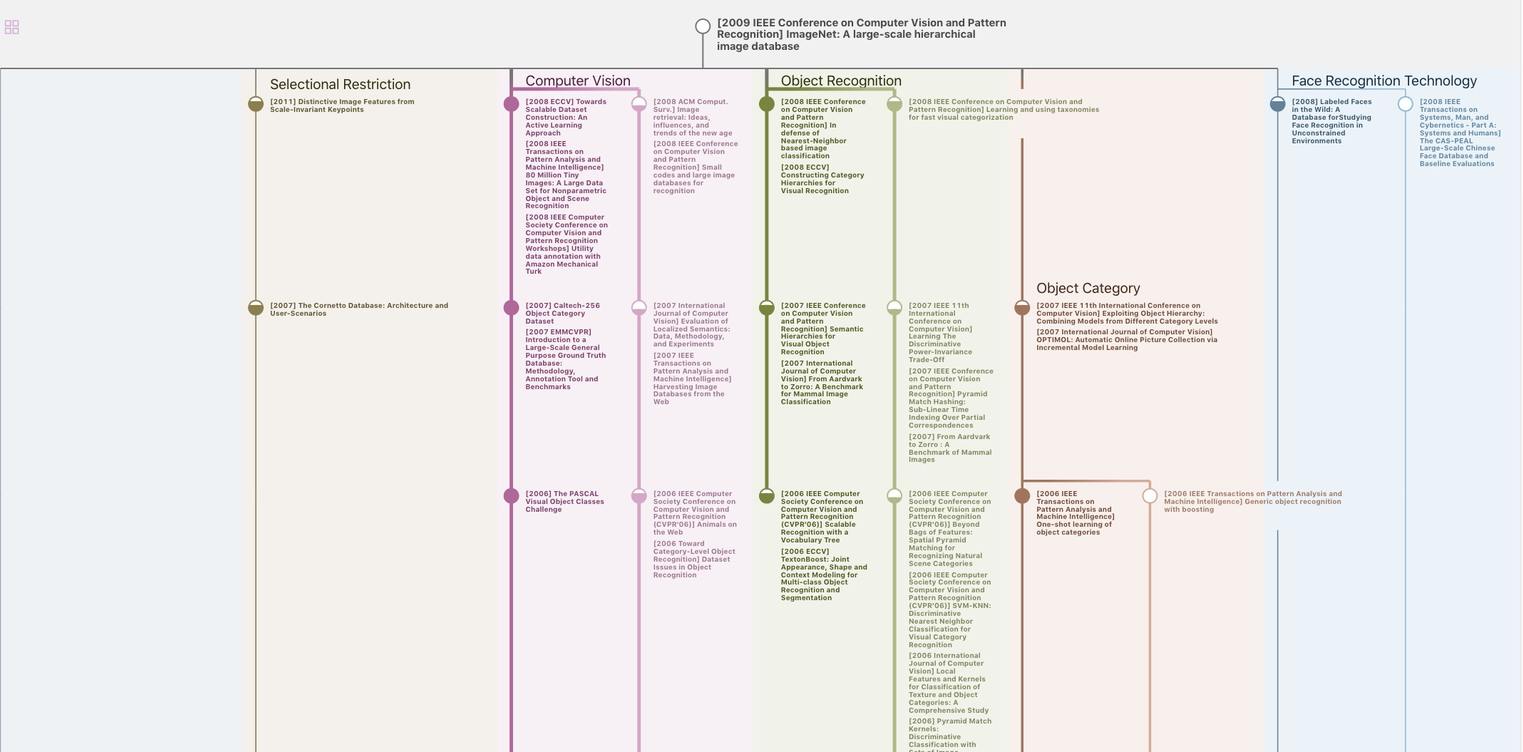
生成溯源树,研究论文发展脉络
Chat Paper
正在生成论文摘要