Adaptive context- and scale-aware aggregation with feature alignment for one-shot object detection
Neurocomputing(2022)
摘要
Given a query image of a novel object category at the inference stage, One-Shot Object Detection (OSOD) aims to target the detection towards reference category through the guidance of query image without fine-tuning. It can be widely applied to many realistic applications but remains challenging so far. Existing attention-based models mainly utilize query features to modulate the target branch to finish features retrieval and information propagation, which generally cannot comprehensively exploit context extracted from the only template to mine out co-occurrent object features, also neglect the cross-scale and feature spatial misalignment problems, leading to imprecise results. Observing these problems, we propose an adaptive context- and scale-aware feature aggregation module (ACS), that harnesses global–local context enrichment to fully preserve contextual features, and performs conditioned multi-scale interaction to learn scale-invariant representations. To tackle the spatial misalignment issue between the query image and generated proposals, we leverage the spatial transformer network (STN) to align features, which facilitates the subtask of classification. Extensive experiments on multiple OSOD benchmarks show that our proposed approach significantly outperforms the baseline by a large margin and achieves state-of-the-art results, demonstrating its effectiveness. Meanwhile, the visualization results of geometric semantic matching between query-target image pairs also verify the robustness of our proposed algorithm.
更多查看译文
关键词
One-shot object detection,Context enrichment,Multi-scale interaction,Feature alignment
AI 理解论文
溯源树
样例
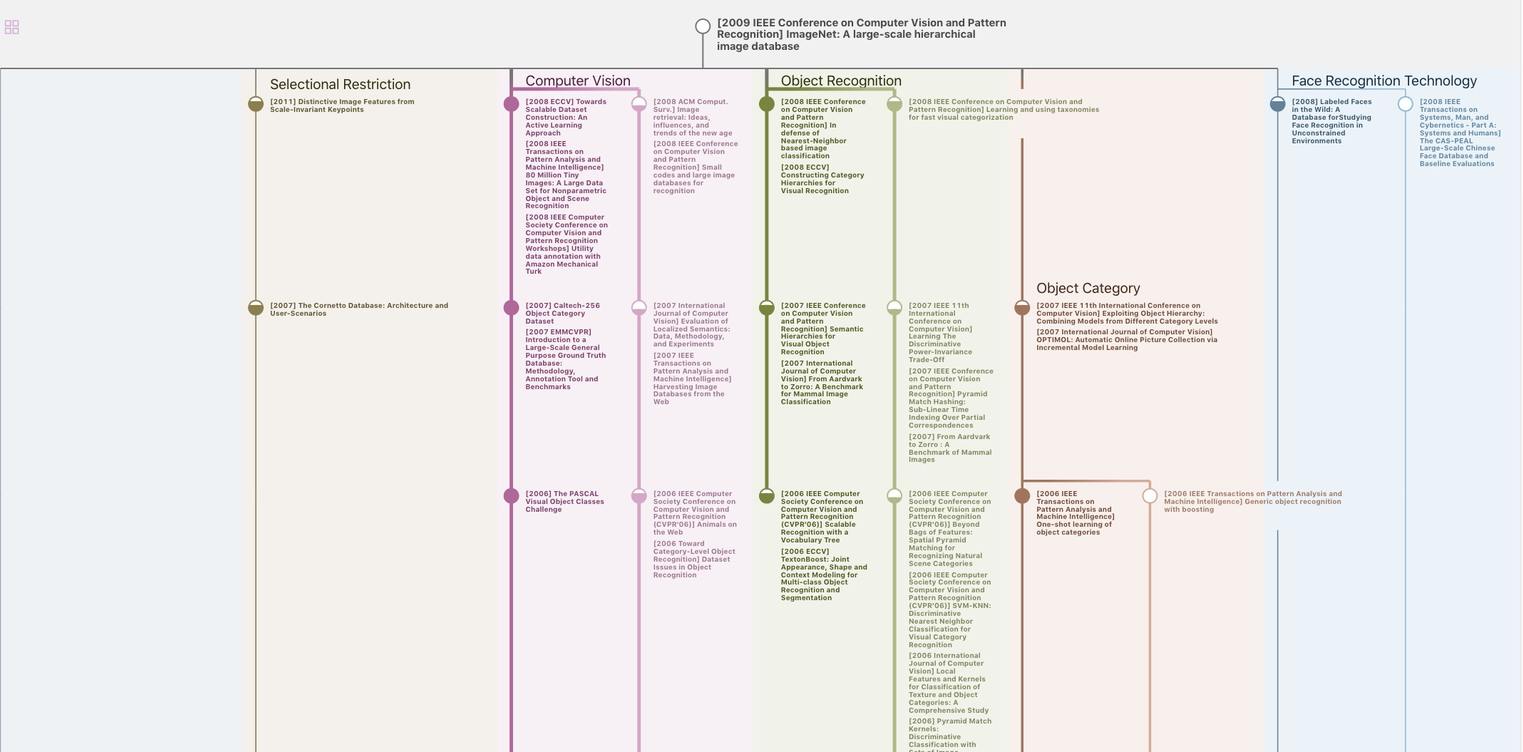
生成溯源树,研究论文发展脉络
Chat Paper
正在生成论文摘要